Target-oriented Semi-supervised Domain Adaptation for WiFi-based HAR
IEEE INFOCOM 2022 - IEEE Conference on Computer Communications(2022)
Univ Sci & Technol China | Univ Mississippi | Beijing Inst Technol | Tsinghua Univ | Xi An Jiao Tong Univ
- Pretraining has recently greatly promoted the development of natural language processing (NLP)
- We show that M6 outperforms the baselines in multimodal downstream tasks, and the large M6 with 10 parameters can reach a better performance
- We propose a method called M6 that is able to process information of multiple modalities and perform both single-modal and cross-modal understanding and generation
- The model is scaled to large model with 10 billion parameters with sophisticated deployment, and the 10 -parameter M6-large is the largest pretrained model in Chinese
- Experimental results show that our proposed M6 outperforms the baseline in a number of downstream tasks concerning both single modality and multiple modalities We will continue the pretraining of extremely large models by increasing data to explore the limit of its performance
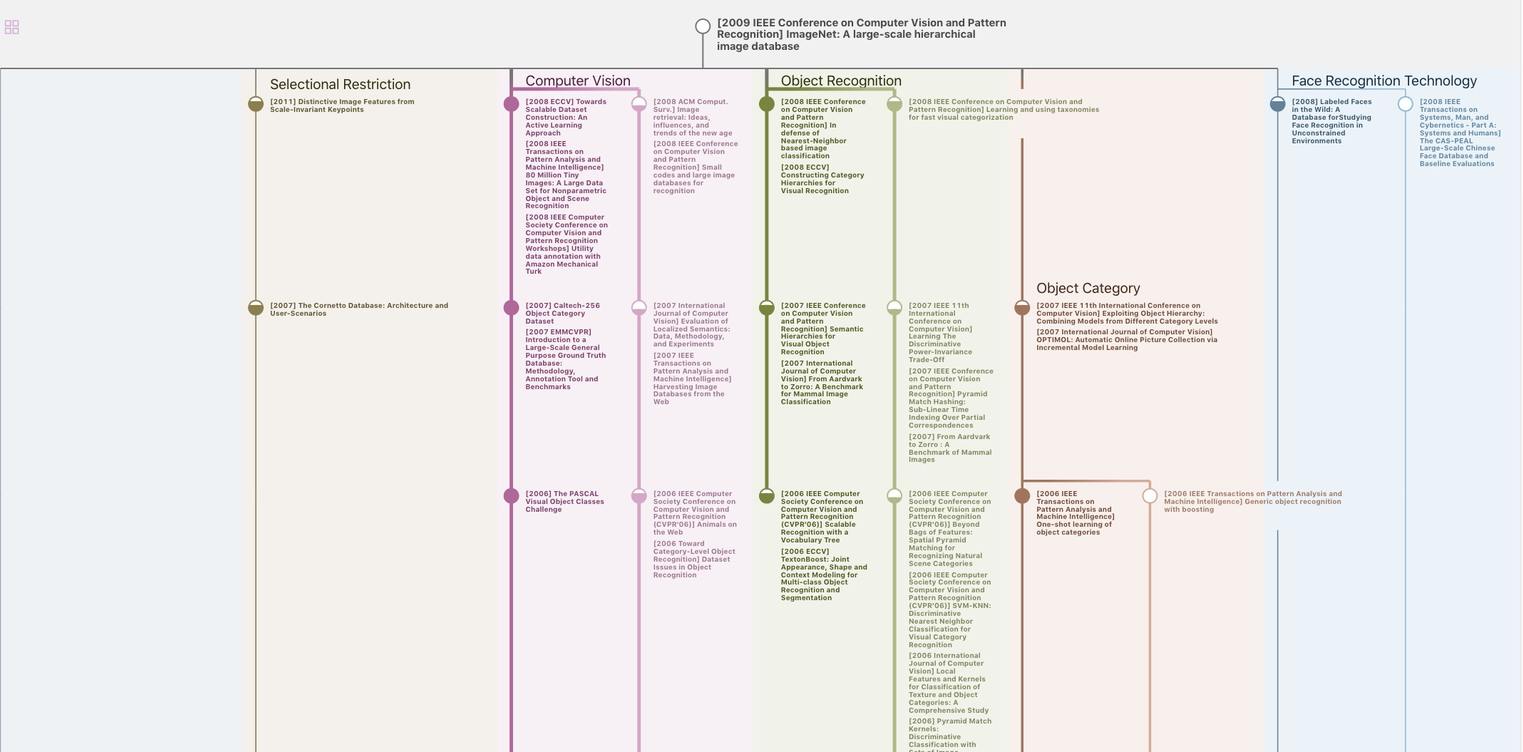
Online Domain Adaptive Classification for Mobile-to-Edge Computing.
被引用0
Federated Inverse Reinforcement Learning for Smart ICUs with Differential Privacy
被引用12
MAA: Modulation-adaptive Acoustic Gesture Recognition
被引用0
I-Sample: Augment Domain Adversarial Adaptation Models for WiFi-based HAR
被引用3
Cross-network Embeddings Transfer for Traffic Analysis
被引用0
RoSeFi: A Robust Sedentary Behavior Monitoring System with Commodity WiFi Devices
被引用0
被引用0
MetaFormer: Domain-Adaptive WiFi Sensing with Only One Labelled Target Sample.
被引用0
被引用0
WiCAR: A Class-Incremental System for WiFi Activity Recognition
被引用0