LANTERN: Boredom-conscious Natural Language Description Generation of Query Execution Plans for Database Education
Proceedings of the 2022 International Conference on Management of Data(2022)
Xidian Univ | Nanyang Technol Univ
Abstract
The database systems course in an undergraduate computer science degree program is gaining increasing importance due to the continuous supply of database-related jobs as well as the rise of Data Science. A key learning goal of learners taking such a course is to understand how SQL queries are executed in an RDBMS in practice. An RDBMS typically exposes a query execution plan (QEP) in a visual or textual format, which describes the execution steps for a given query. However, it is often daunting for a learner to comprehend these QEPs containing vendor-specific implementation details. In this demonstration, we present a novel, generic, and portable system called LANTERN that generates a natural language (NL)-based description of the execution strategy chosen by the underlying RDBMS to process a query. It provides a declarative framework called POOL for subject matter experts (SME) to efficiently create and manipulate the NL descriptions of physical operators of any RDBMS. It then exploits POOL to generate the NL descriptions of QEPs by integrating a rule-based and a deep learning-based techniques to infuse language variability in the descriptions. Such an NL generation strategy mitigates the impact of boredom on learners caused by repeated exposure of similar text generated by a rule-based system.
MoreTranslated text
Key words
database education,query execution plan,natural language generation
求助PDF
上传PDF
View via Publisher
AI Read Science
AI Summary
AI Summary is the key point extracted automatically understanding the full text of the paper, including the background, methods, results, conclusions, icons and other key content, so that you can get the outline of the paper at a glance.
Example
Background
Key content
Introduction
Methods
Results
Related work
Fund
Key content
- Pretraining has recently greatly promoted the development of natural language processing (NLP)
- We show that M6 outperforms the baselines in multimodal downstream tasks, and the large M6 with 10 parameters can reach a better performance
- We propose a method called M6 that is able to process information of multiple modalities and perform both single-modal and cross-modal understanding and generation
- The model is scaled to large model with 10 billion parameters with sophisticated deployment, and the 10 -parameter M6-large is the largest pretrained model in Chinese
- Experimental results show that our proposed M6 outperforms the baseline in a number of downstream tasks concerning both single modality and multiple modalities We will continue the pretraining of extremely large models by increasing data to explore the limit of its performance
Upload PDF to Generate Summary
Must-Reading Tree
Example
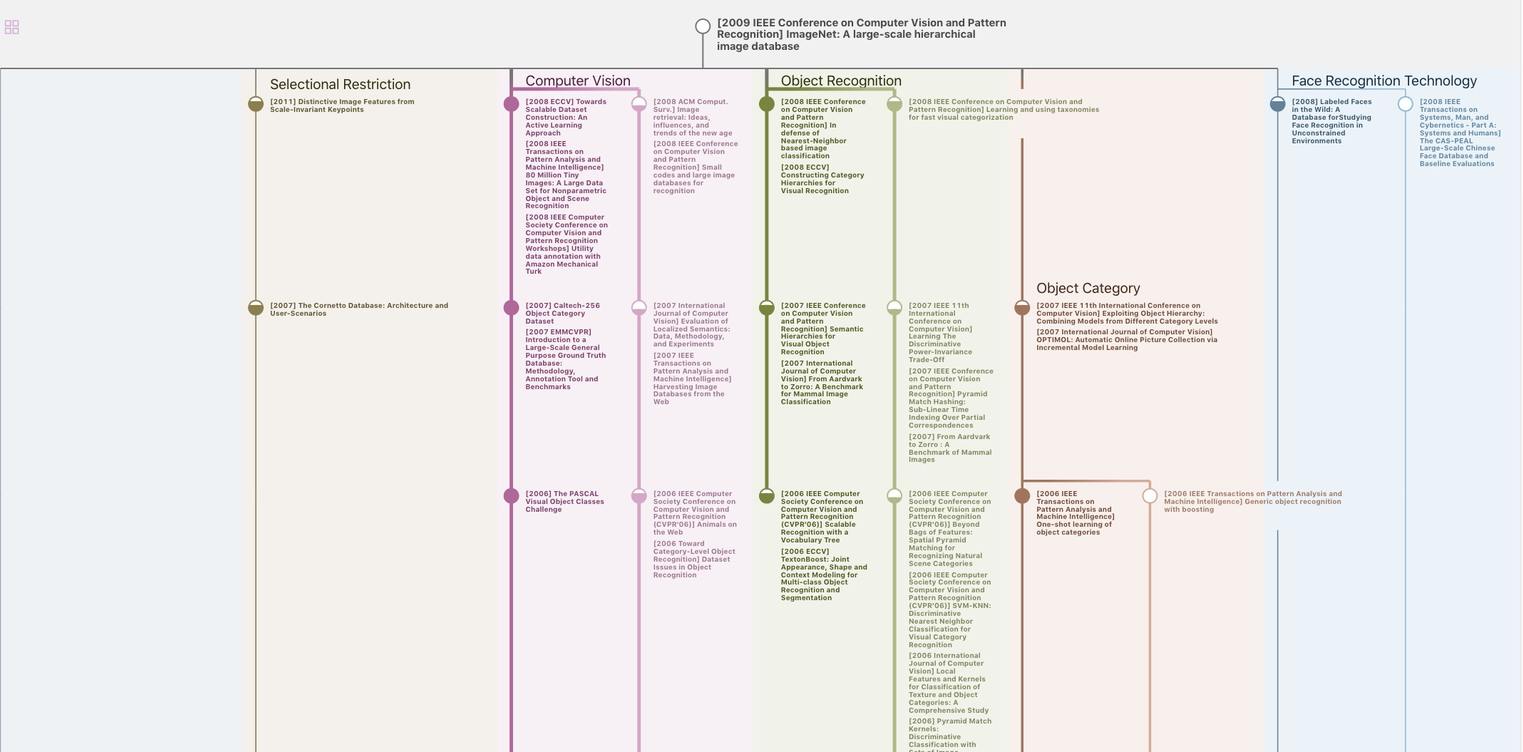
Generate MRT to find the research sequence of this paper
Related Papers
Proceedings of the VLDB Endowment 2022
被引用13
DBinsight: A Tool for Interactively Understanding the Query Processing Pipeline in RDBMSs
Proceedings of the 31st ACM International Conference on Information & Knowledge Management 2022
被引用1
Query Execution Plans and Semantic Errors: Usability and Educational Opportunities.
CHI Extended Abstracts 2023
被引用4
Data Disclaimer
The page data are from open Internet sources, cooperative publishers and automatic analysis results through AI technology. We do not make any commitments and guarantees for the validity, accuracy, correctness, reliability, completeness and timeliness of the page data. If you have any questions, please contact us by email: report@aminer.cn
Chat Paper