Linking Entities across Relations and Graphs
2022 IEEE 38th International Conference on Data Engineering (ICDE)(2022)
摘要
This paper proposes a notion of parametric simulation to link entities across a relational database
$\mathcal{D}$
and a graph
$G$
. Taking functions and thresholds for measuring vertex close-ness, path associations and important properties as parameters, parametric simulation identifies tuples
$t$
in
$\mathcal{D}$
and vertices
$v$
in
$G$
that refer to the same real-world entity, based on topological and semantic matching. We develop machine learning methods to learn the parameter functions and thresholds. We show that parametric simulation is in quadratic-time, by providing such an algorithm. Putting these together, we develop HER, a parallel system to check whether
$(t,v)$
makes a match, find all vertex matches of
$t$
in
$G$
, and compute all matches across
$\mathcal{D}$
and
$G$
, all in quadratic-time. Using real-life and synthetic data, we empirically verify that HER is accurate with
$\mathbf{F}$
-measure of 0.94 on average, and is able to scale with database
$\mathcal{D}$
and graph
$G$
.
更多查看译文
关键词
Entity resolution,Data integration,Graph data management,Parametric simulation,Heterogeneous entity resolution
AI 理解论文
溯源树
样例
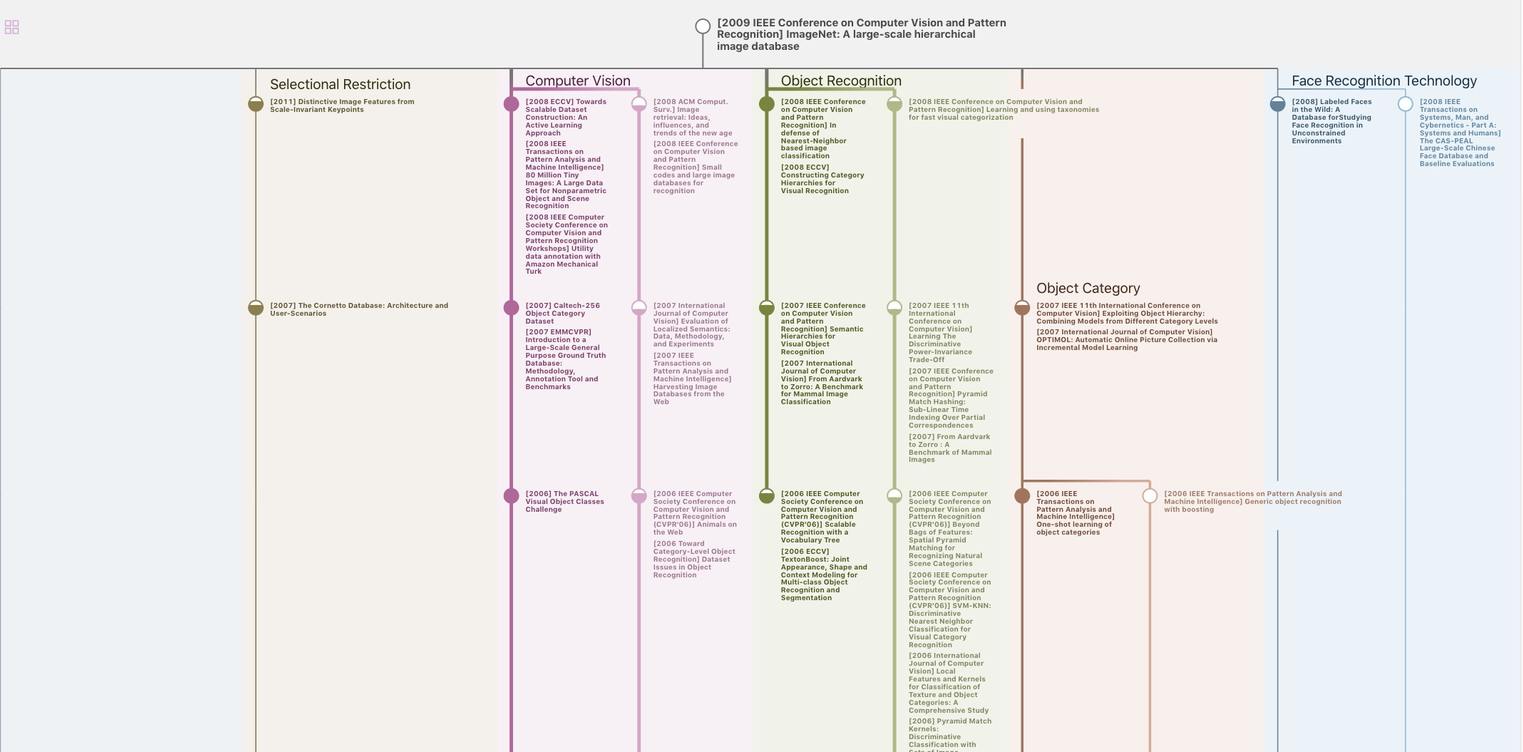
生成溯源树,研究论文发展脉络
Chat Paper
正在生成论文摘要