Mixing Transactions with Arbitrary Values on Blockchains
2022 IEEE 38TH INTERNATIONAL CONFERENCE ON DATA ENGINEERING (ICDE 2022)(2022)
摘要
Due to the transparency of blockchain, adversaries can observe the details of a transaction, and then utilize the amount as a unique quasi-identifier to make deanonymization. Nowadays, to obscure the linkages between receivers and senders within a transaction on the blockchain, mixing services are widely applied in many real applications to enhance cryptocurrencies' anonymity. The basic idea of mixing services is to hide an output within several other outputs in a transaction such that adversaries cannot distinguish them by their amounts since they are purposely selected to have the same amount. For a set of original outputs with different amounts, mixing services need to decompose them into a set of decomposed outputs, where any decomposed output has some other decomposed outputs with the same amount. Since the transaction fee is related to the number of outputs, we are motivated to decompose original outputs into a minimal set of decomposed outputs, which is challenging to guarantee the privacy-preserving effect at the same time. In this paper, we formally define the anonymity-aware output decomposition (AA-OD) problem, which aims to find a c-decomposition with a minimum number of decomposed outputs for a given original output set. A c-decomposition guarantees that for any original output
$o$
, there are at most
$c$
of all decomposed outputs with an amount of
$x$
coming from
$o$
. We prove that the AA-OD problem is NP-hard. Thus, we propose an approximation algorithm, namely Boggart
1
1
Boggart is a magical creature in J. K. Rowling's Harry Potter series who can shift his shape and no one knows what it looks like., to solve the AA-OD problem with a (2/c + 3)-approximation bound on the number of decomposed outputs. We verify the efficiency and effectiveness of our approach through comprehensive experiments on both real and synthetic data sets.
更多查看译文
关键词
Blockchain, Mixing Service, Privacy
AI 理解论文
溯源树
样例
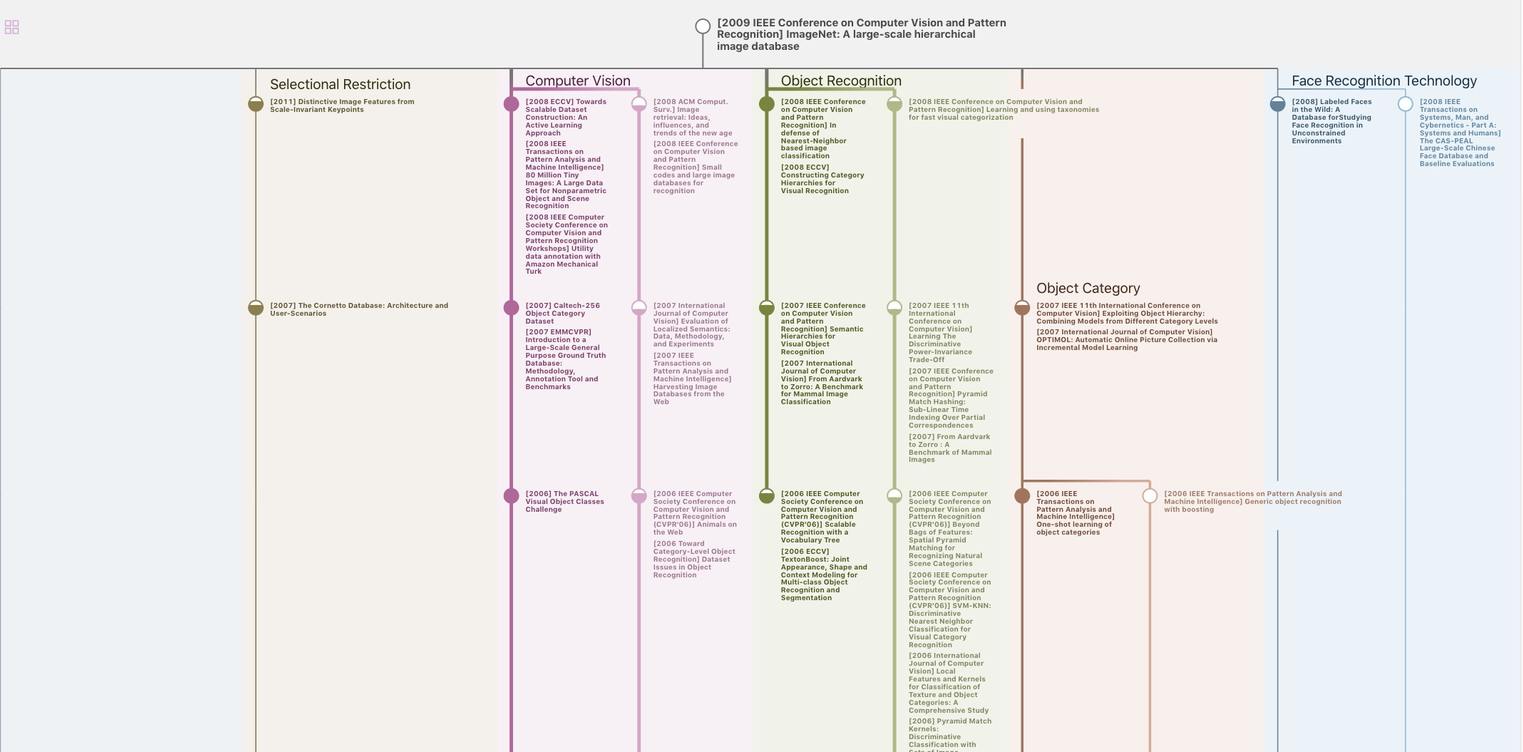
生成溯源树,研究论文发展脉络
Chat Paper
正在生成论文摘要