Farming Your ML-based Query Optimizer's Food
2022 IEEE 38TH INTERNATIONAL CONFERENCE ON DATA ENGINEERING (ICDE 2022)(2022)
摘要
Machine learning (ML) is becoming a core component in query optimizers, e.g., to estimate costs or cardinalities. This means large heterogeneous sets of labeled query plans or jobs (i.e., plans with their runtime or cardinality output) are needed. However, collecting such a training dataset is a very tedious and time-consuming task: It requires both developing numerous jobs and executing them to acquire ground-truth labels. We demonstrate DATAFARM, a novel framework for efficiently generating and labeling training data for ML-based query optimizers to overcome these issues. DATAFARM enables generating training data tailored to users' needs by learning from their existing workload patterns, input data, and computational resources. It uses an active learning approach to determine a subset of jobs to be executed and encloses the human into the loop, resulting in higher quality data. The graphical user interface of DATAFARM allows users to get informative details of the generated jobs and guides them through the generation process step-by-step. We show how users can intervene and provide feedback to the system in an iterative fashion. As an output, users can download both the generated jobs to use as a benchmark and the training data (jobs with their labels).
更多查看译文
关键词
ML based query optimization,training data,human in the loop,active learning,data generation
AI 理解论文
溯源树
样例
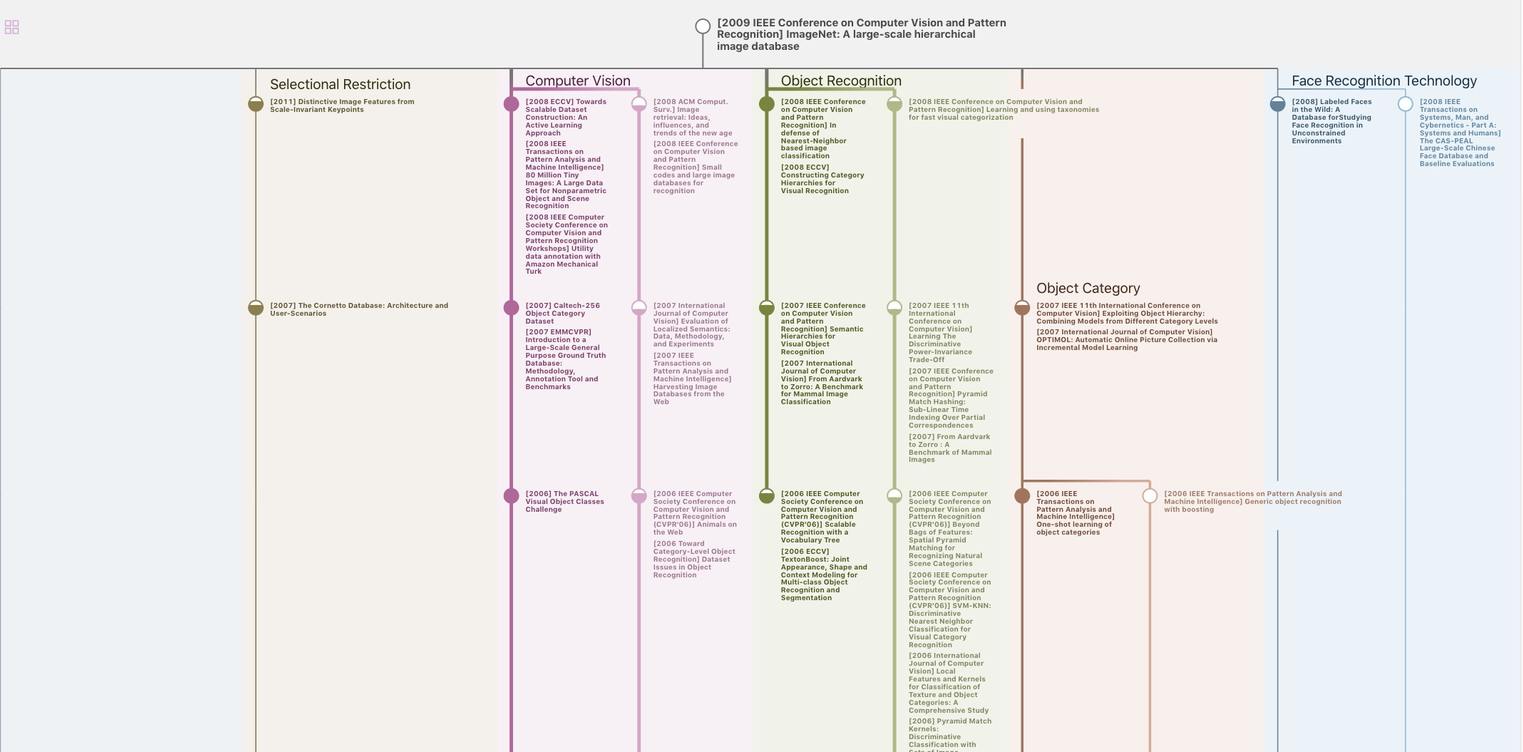
生成溯源树,研究论文发展脉络
Chat Paper
正在生成论文摘要