Diffeomorphic Neural Surface Parameterization for 3D and Reflectance Acquisition
International Conference on Computer Graphics and Interactive Techniques(2022)
摘要
BSTRACT This paper proposes a simple method which solves the problem of multi-view 3D reconstruction for objects with unknown and generic surface materials, imaged by a freely moving camera and lit by a freely moving point light source. The object can have arbitrary (diffuse or specular) and spatially-varying surface reflectances. Our solution consists of two small-sized neural networks (dubbed the ‘Shape-Net’ and ‘BRDF-Net’), used to parameterize the unknown shape and material map as functions on a canonical surface (e.g. unit sphere). Key to our method is a velocity field shape representation that drives the canonical surface to target shape through time. We show this parameterization can be implemented as a recurrent residual network that is guaranteed to be diffeomorphic and orientation-preserving. Our method yields an exceptionally clean formulation that can be optimized by standard gradient descent without initialization, and works with both near-field and distant light source. Synthetic and real experiments demonstrate the reliability and accuracy of our reconstructions, with extensions including novel-view-synthesis, relighting and material retouching done with ease. Our source codes are available at https://github.com/za-cheng/DNS.
更多查看译文
关键词
reflectance,surface,3d
AI 理解论文
溯源树
样例
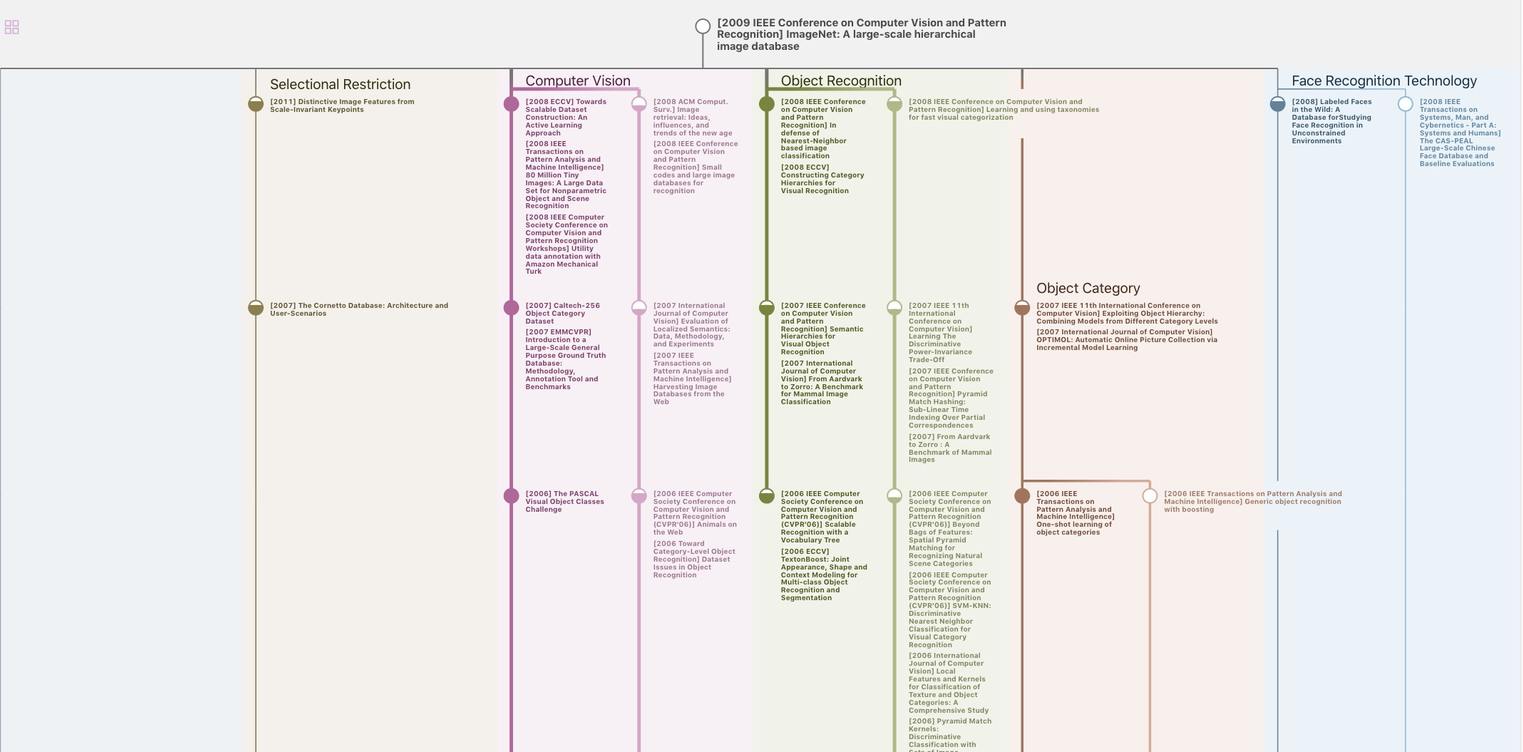
生成溯源树,研究论文发展脉络
Chat Paper
正在生成论文摘要