No-Reference Image Quality Assessment Using Dynamic Complex-Valued Neural Model
International Multimedia Conference(2022)
摘要
ABSTRACTDeep convolutional neural networks (CNNs) have become a promising approach to no-reference image quality assessment (NR-IQA). This paper aims at improving the power of CNNs for NR-IQA in two aspects. Firstly, motivated by the deep connection between complex-valued transforms and human visual perception, we introduce complex-valued convolutions and phase-aware activations beyond traditional real-valued CNNs, which improves the accuracy of NR-IQA without bringing noticeable additional computational costs. Secondly, considering the content-awareness of visual quality perception, we include a dynamic filtering module for better extracting content-aware features, which predicts features based on both local content and global semantics. These two improvements lead to a complex-valued content-aware neural NR-IQA model with good generalization. Extensive experiments on both synthetically and authentically distorted data have demonstrated the state-of-the-art performance of the proposed approach.
更多查看译文
关键词
quality assessment,neural model,image,no-reference,complex-valued
AI 理解论文
溯源树
样例
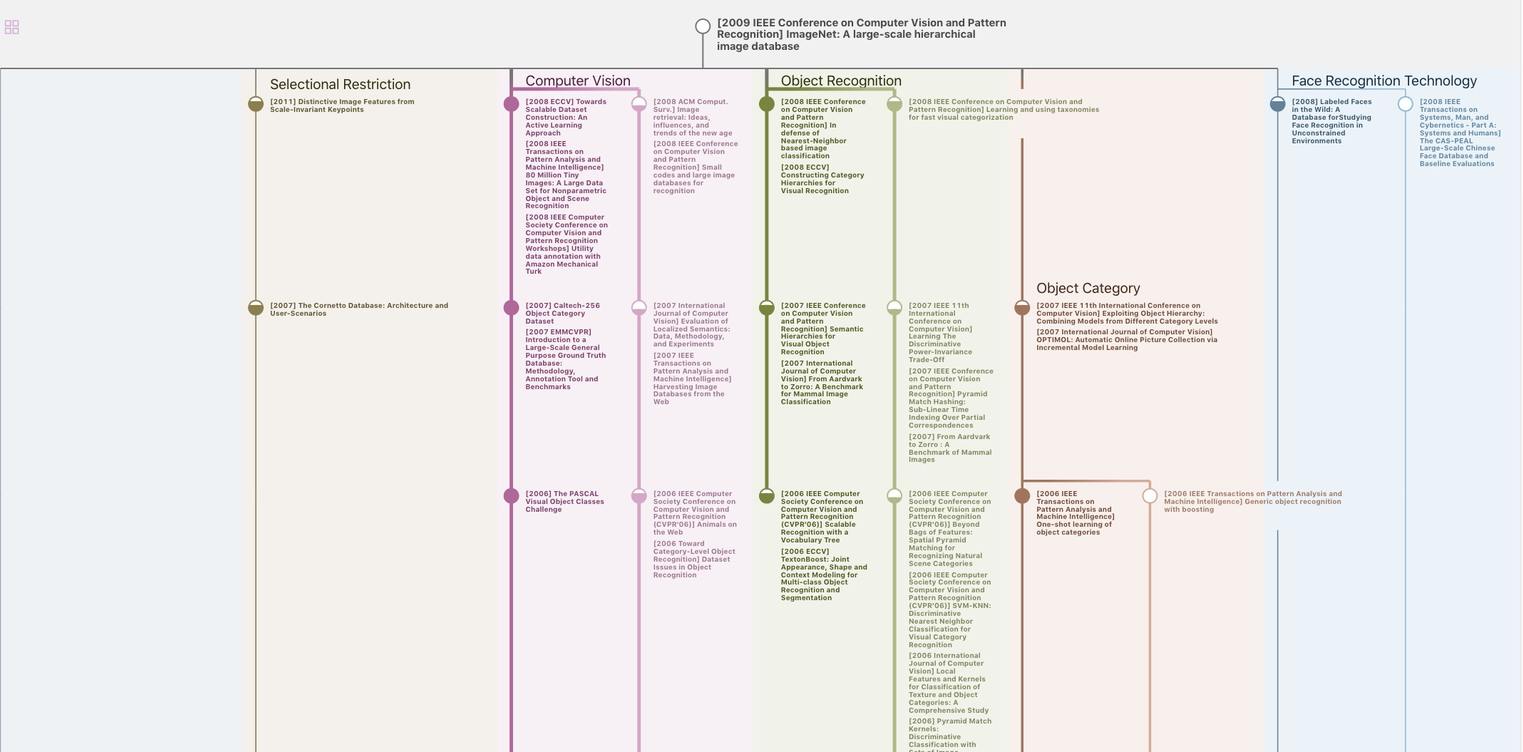
生成溯源树,研究论文发展脉络
Chat Paper
正在生成论文摘要