Dynamic Incomplete Multi-view Imputing and Clustering
International Multimedia Conference(2022)
摘要
ABSTRACTIncomplete multi-view clustering (IMVC) is deemed a significant research topic in multimedia to handle data loss situations. Current late fusion incomplete multi-view clustering methods have attracted intensive attention owing to their superiority in using consensus partition for effective and efficient imputation and clustering. However, 1) their imputation quality and clustering performance depend heavily on the static prior partition, such as predefined zeros filling, destroying the diversity of different views; 2) the size of base partitions is too small, which would lose advantageous details of base kernels to decrease clustering performance. To address these issues, we propose a novel IMVC method, named Dynamic Incomplete Multi-view Imputing and Clustering (DIMIC). Concretely, the observed views dynamically generate a consensus proxy with the guidance of a shared cluster matrix for more effective imputation and clustering, rather than a fixed predefined partition matrix. Furthermore, the proper size of base partitions is employed to protect sufficient kernel details for further enhancing the quality of the consensus proxy. By designing a solver with a linear computational and memory complexity on extensive experiments, our effectiveness, superiority, and efficiency are validated on multiple public datasets with recent advances.
更多查看译文
关键词
dynamic incomplete,clustering,multi-view
AI 理解论文
溯源树
样例
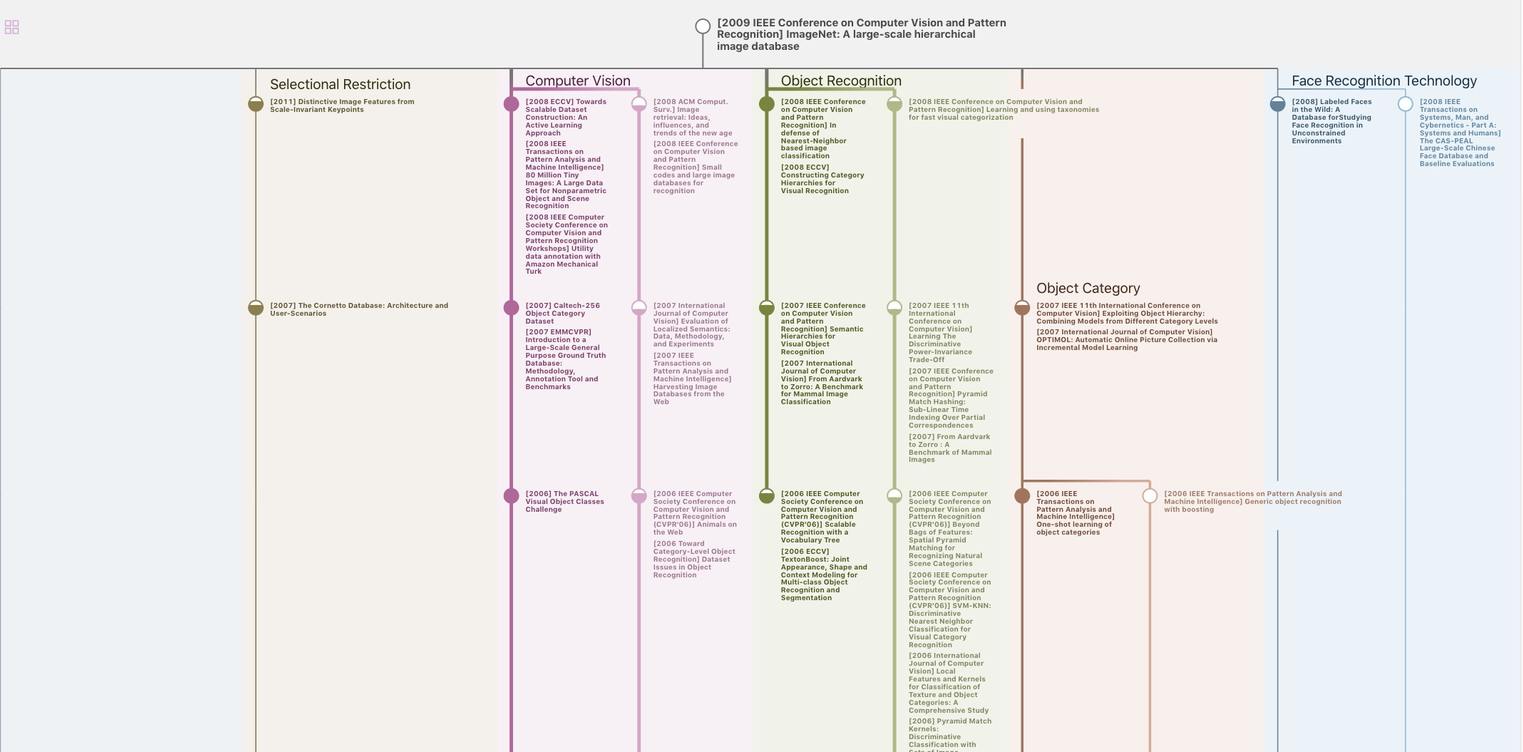
生成溯源树,研究论文发展脉络
Chat Paper
正在生成论文摘要