Few-shot X-ray Prohibited Item Detection: A Benchmark and Weak-feature Enhancement Network
International Multimedia Conference(2022)
摘要
ABSTRACTX-ray prohibited items detection of security inspection plays an important role in protecting public safety. It is a typical few-shot object detection (FSOD) task because some categories of prohibited items are highly scarce due to low-frequency appearance, e.g. pistols, which has been ignored by recent X-ray detection works. In contrast to most FSOD studies that rely on rich feature correlations from natural scenarios, the more practical X-ray security inspection usually faces the dilemma of only weak features learnable due to heavy occlusion, color fading, etc, which causes a severe performance drop when traditional FSOD methods are adopted. However, professional X-ray FSOD evaluation benchmarks and effective models of this scenario have been rarely studied in recent years. Therefore, in this paper, we propose the first X-ray FSOD dataset on the typical industrial X-ray security inspection scenario consisting of 12,333 images and 41,704 instances from 20 categories, which could benchmark and promote FSOD studies in such more challenging scenarios. Further, we propose the Weak-feature Enhancement Network (WEN) containing two core modules, i.e. Prototype Perception (PR) and Feature Reconciliation (FR), where PR first generates a prototype library by aggregating and extracting the basis feature from critical regions around instances, to generate the basis information for each category; FR then adaptively adjusts the impact intensity of the corresponding prototype and forces the model to precisely enhance the weak features of specific objects through the basis information. This mechanism is also effective in traditional FSOD tasks. Extensive experiments on X-ray FSOD and Pascal VOC datasets demonstrate that WEN outperforms other baselines in both X-ray and common scenarios.
更多查看译文
关键词
detection,few-shot,x-ray,weak-feature
AI 理解论文
溯源树
样例
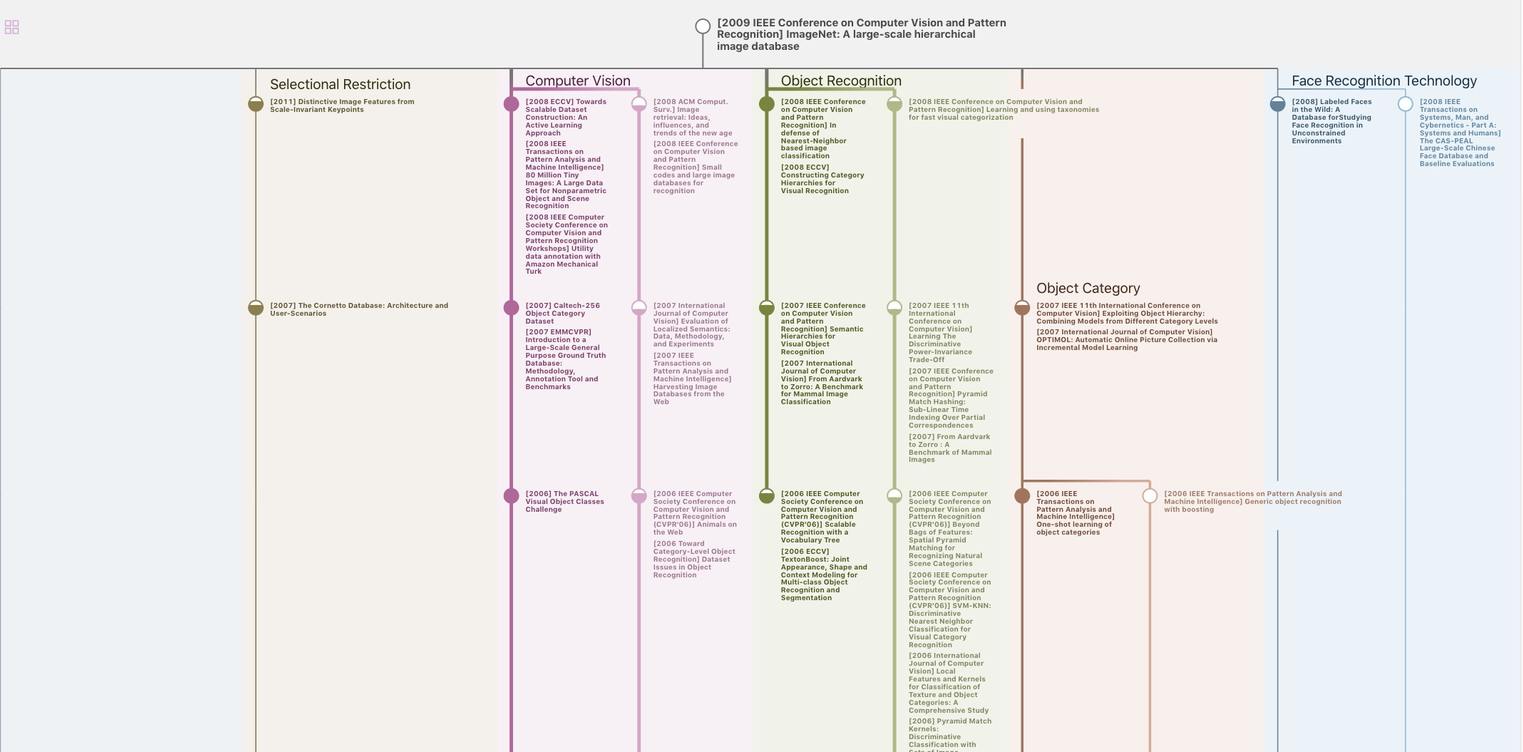
生成溯源树,研究论文发展脉络
Chat Paper
正在生成论文摘要