GCL: Graph Calibration Loss for Trustworthy Graph Neural Network
International Multimedia Conference(2022)
摘要
ABSTRACTDespite the great success of Graph Neural Networks (GNNs), the trustworthiness is still lack-explored. A very recent study suggests that GNNs are under-confident on the predictions which is opposite to deep neural networks. In this paper, we investigate why this is the case. We discover that the "shallow" network of GNNs is the central cause. To address this challenge, we propose a novel Graph Calibration Loss (GCL), the first end-to-end calibration method for GNNs, which reshapes the standard Cross Entropy loss and is encouraged to assign up-weights loss to high-confidence examples. Through empirical observation and theoretical justification, we discover the GCL's calibration mechanism is to add a minimal-entropy regulariser to KL-divergence to bring down the entropy of correctly classified samples. To evaluate the effectiveness of the GCL, we train several representative GNNs models which use the GCL as loss function on various citation networks datasets, and further apply the GCL to a self-training framework. Compared to the existed methods, the proposed method achieves state-of-the-art calibration performance on node classification task and even improves the standard classification accuracy in almost all cases.
更多查看译文
AI 理解论文
溯源树
样例
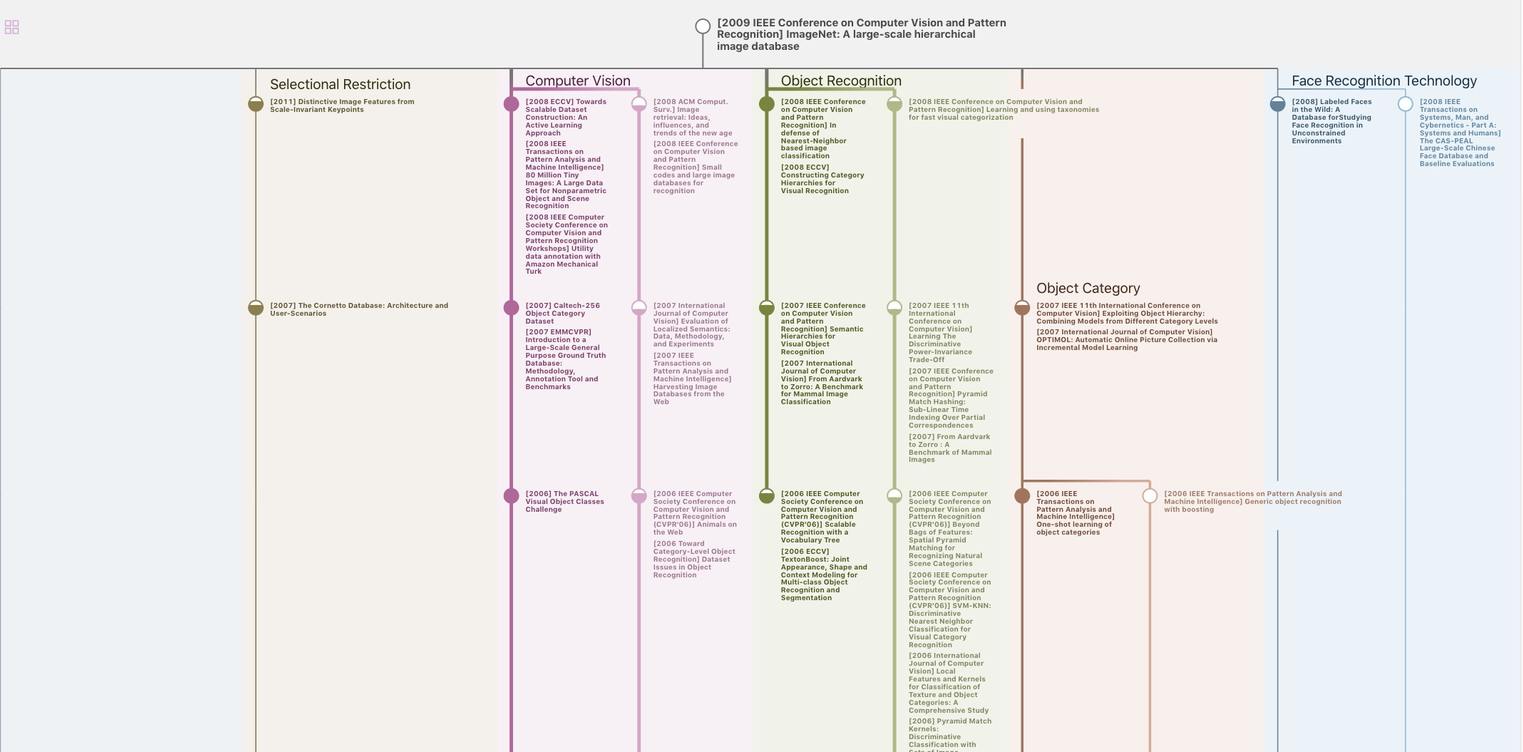
生成溯源树,研究论文发展脉络
Chat Paper
正在生成论文摘要