OCR-Pose: Occlusion-aware Contrastive Representation for Unsupervised 3D Human Pose Estimation
Proceedings of the 30th ACM International Conference on Multimedia(2022)
摘要
Occlusion is a significant problem in 3D human pose estimation from the 2D counterpart. On one hand, without explicit annotation, the 3D skeleton is hard to be accurately estimated from the occluded 2D pose. On the other hand, one occluded 2D pose might correspond to multiple 3D skeletons with low confidence parts. To address these issues, we decouple the 3D representation feature into view-invariant part termed occlusion-aware feature and view-dependent part termed rotation feature to facilitate subsequent optimization of the former. Then we propose an occlusion-aware contrastive representation based scheme (OCR-Pose) consisting of Topology Invariant Contrastive Learning module (TiCLR) and View Equivariant Contrastive Learning module (VeCLR). Specifically, TiCLR drives invariance to topology transformation, i.e., bridging the gap between an occluded 2D pose and the unoccluded one. While VeCLR encourages equivariance to view transformation, i.e., capturing the geometric similarity of the 3D skeleton in two views. Both modules optimize occlusion-aware constrastive representation with pose filling and lifting networks via an iterative training strategy in an end-to-end manner. OCR-Pose not only achieves superior performance against state-of-the-art unsupervised methods on unoccluded benchmarks, but also obtains significant improvements when occlusion is involved. Our project is available at https://sites.google.com/view/ocr-pose.
更多查看译文
关键词
3D vision,human pose estimation,representation learning
AI 理解论文
溯源树
样例
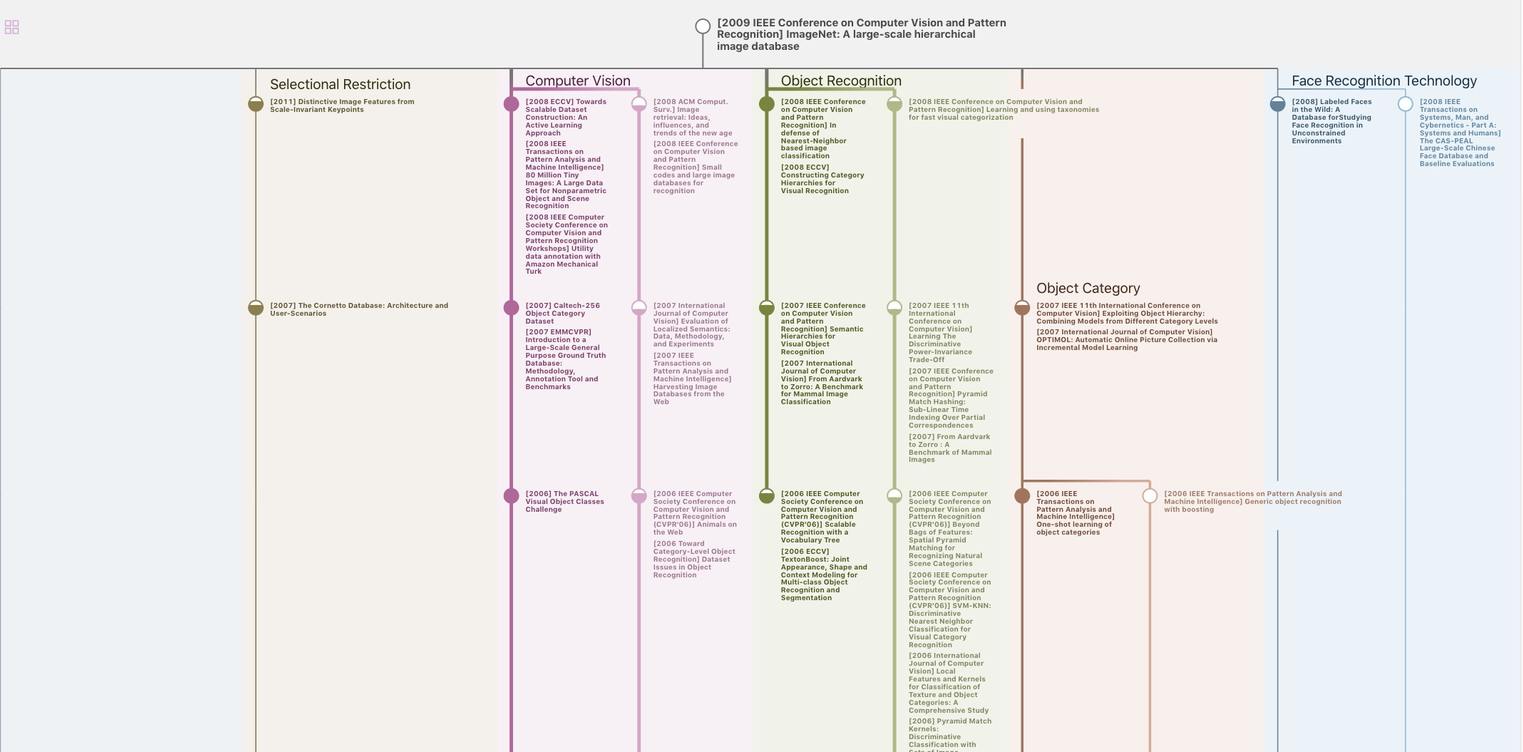
生成溯源树,研究论文发展脉络
Chat Paper
正在生成论文摘要