TextBlock: Towards Scene Text Spotting without Fine-grained Detection
International Multimedia Conference(2022)
摘要
ABSTRACTScene text spotting systems which integrate text detection and recognition modules have witnessed a lot of success in recent years. Existing works mostly follow the framework of word/character-level fine-grained detection and isolated-instance recognition, which overemphasize the role of detector and ignore the rich context information in recognition. After rethinking the conventional framework, and inspired by the glimpse-focus spotting pipeline of human beings, we ask:1) "can machine spot text without accurate detection just like human beings?", and if yes, 2) "is text block another alternative for scene text spotting other than word or character?". Based on these questions, we propose a new perspective of coarse-grained detection with multi-instance recognition for text spotting. Specifically, a pioneering network termed TextBlock is developed, and a heuristic text block generation method as well as a multi-instance block-level recognition module are proposed. In this way, the burden of detection is relieved, and the contextual semantic information is well explored for recognition. To train the block-level recognizer, a synthetic dataset including about 800K images is formed. As a by-product of attention, fine-grained detection can be recovered with the recognizer. Equipped with a detector without many bells and whistles (e.g., Faster R-CNN), TextBlock achieves competitive or even better performance compared with previous sophisticated text spotters on several public benchmarks. As a primary attempt, we expect this framework will have a potential impact on scene text spotting research in the future.
更多查看译文
AI 理解论文
溯源树
样例
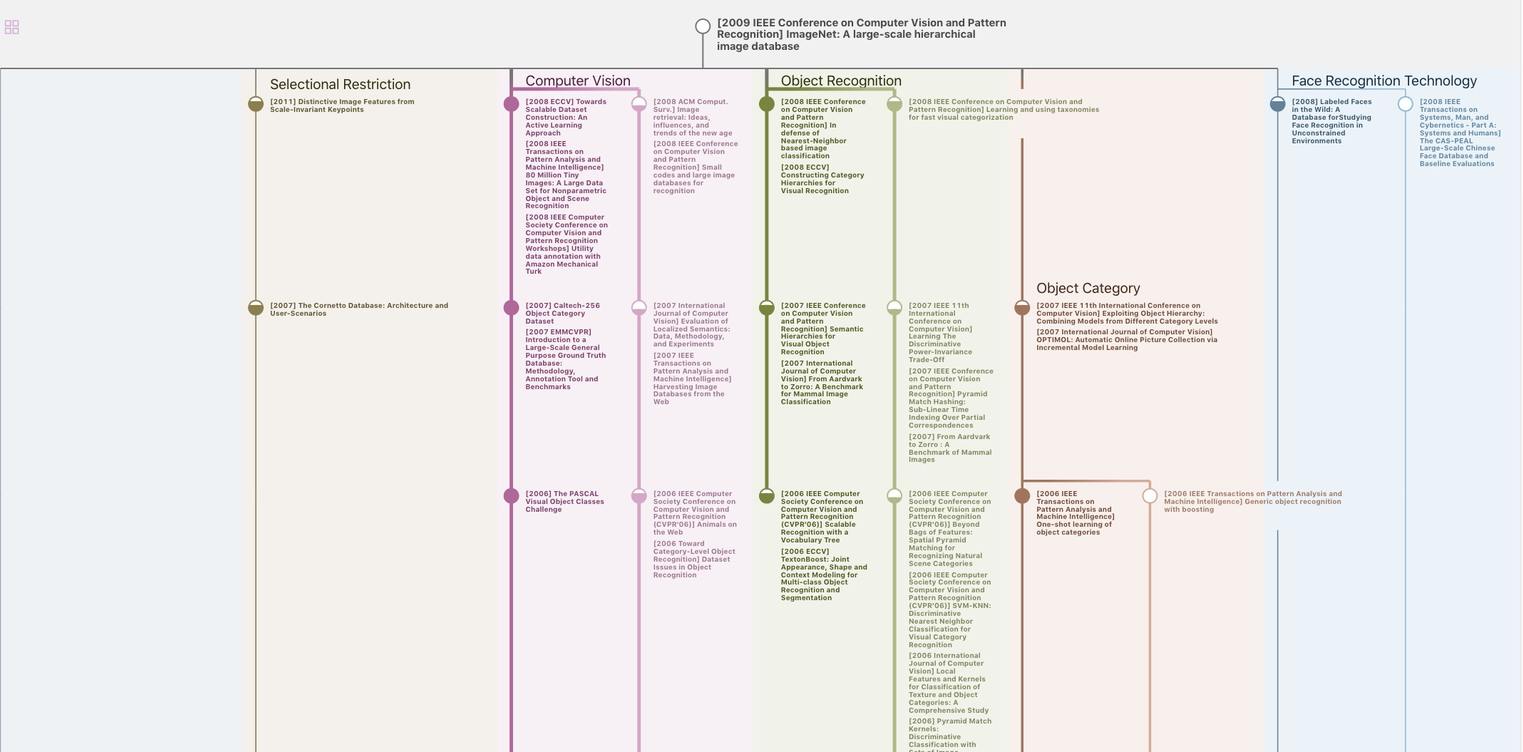
生成溯源树,研究论文发展脉络
Chat Paper
正在生成论文摘要