Robust Diversified Graph Contrastive Network for Incomplete Multi-view Clustering
International Multimedia Conference(2022)
摘要
ABSTRACTIncomplete multi-view clustering is a challenging task which aims to partition the unlabeled incomplete multi-view data into several clusters. The existing incomplete multi-view clustering methods neglect to utilize the diversified correlations inherent in data and handle the noise contained in different views. To address these issues, we propose a Robust Diversified Graph Contrastive Network (RDGC) for incomplete multi-view clustering, which integrates multi-view representation learning and diversified graph contrastive regularization into a unified framework. Multi-view unified and specific encoding network is developed to fuse different views into a unified representation, which can flexibly estimate the importance of views for incomplete multi-view data. Robust diversified graph contrastive regularization is proposed which captures the diversified data correlations to improve the discriminating power of the learned representation and reduce the information loss caused by the view missing problem. Moreover, our method can effectively resist the influence of noise and unreliable views by leveraging the robust contrastive learning loss. Extensive experiments conducted on four multi-view clustering datasets demonstrate the superiority of our method over the state-of-the-art methods.
更多查看译文
关键词
clustering,graph,multi-view
AI 理解论文
溯源树
样例
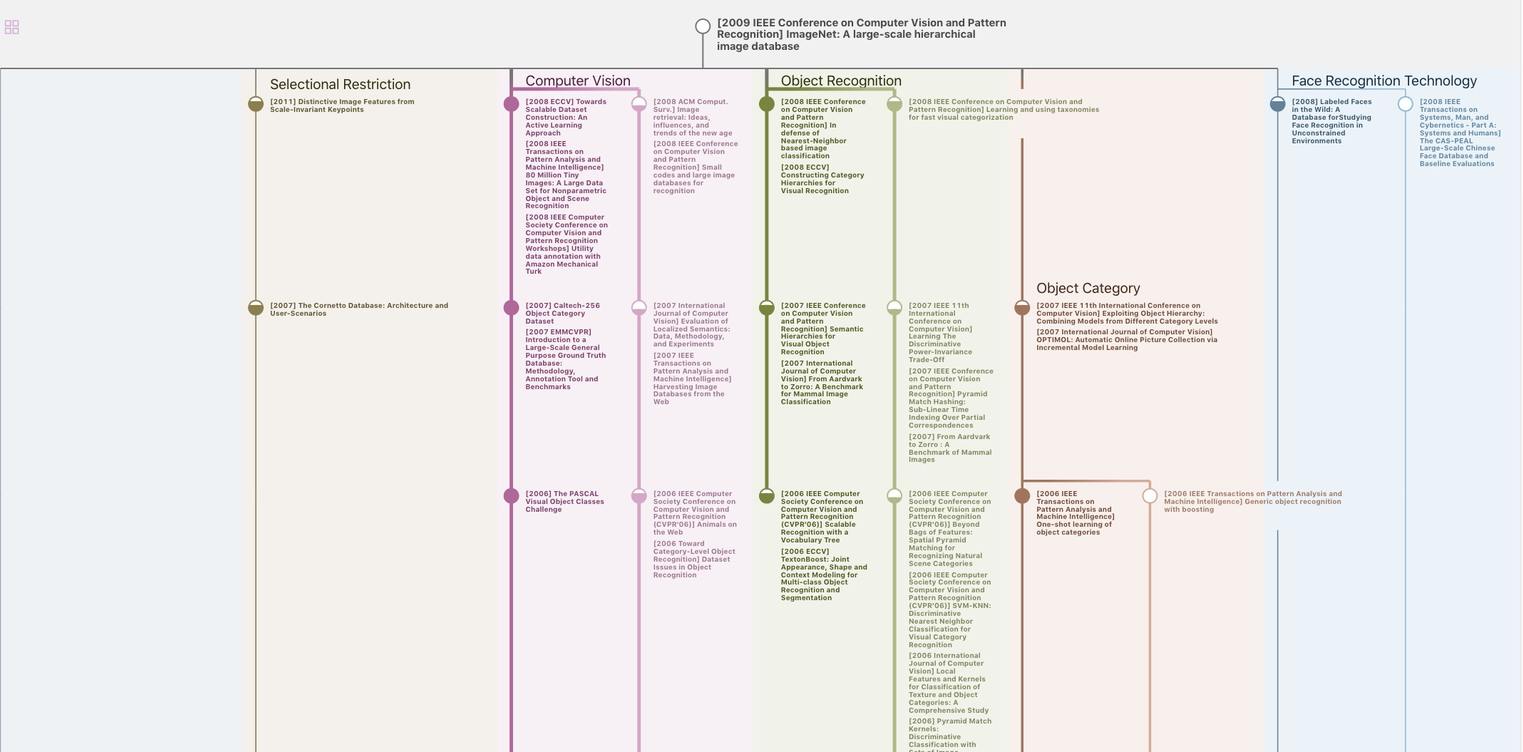
生成溯源树,研究论文发展脉络
Chat Paper
正在生成论文摘要