Align and Adapt: A Two-stage Adaptation Framework for Unsupervised Domain Adaptation
International Multimedia Conference(2022)
摘要
ABSTRACTUnsupervised domain adaptation aims to transfer knowledge from a labeled but heterogeneous source domain to an unlabeled target domain, alleviating the labeling efforts. Early advances in domain adaptation focus on invariant representations learning (IRL) methods to align domain distributions. Recent studies further utilize semi-supervised learning (SSL) methods to regularize domain-invariant representations based on the cluster assumption, making the category boundary more clear. However, the misalignment in the IRL methods might be intensified by SSL methods if the target instances are more proximate to the wrong source centroid, resulting in incompatibility between these techniques. In this paper, we hypothesize this phenomenon derives from the distraction of the source domain, and further give a novel two-stage adaptation framework to adapt the model toward the target domain. In addition, we propose DCAN to reduce the misalignment in IRL methods in the first stage, and we propose PCST to encode the semantic structure of unlabeled target data in the second stage. Extensive experiments demonstrate that our method outperforms current state-of-the-art methods on four benchmarks (Office-31, ImageCLEF-DA, Office-Home, and VisDA-2017).
更多查看译文
关键词
domain adaptation,two-stage
AI 理解论文
溯源树
样例
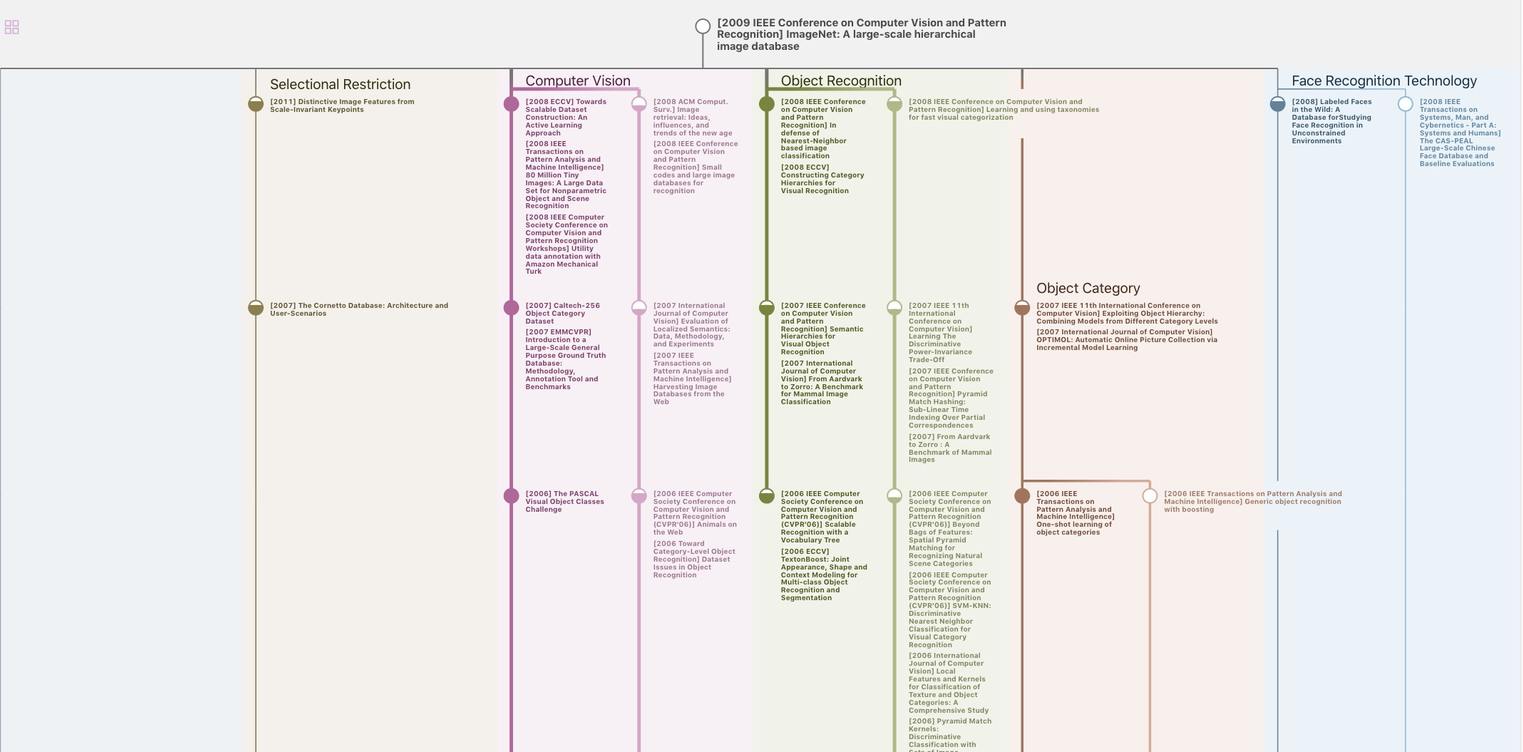
生成溯源树,研究论文发展脉络
Chat Paper
正在生成论文摘要