Semi-Supervised Source Localization With Residual Physical Learning
IEEE International Conference on Acoustics, Speech, and Signal Processing (ICASSP)(2022)
摘要
Machine learning (ML) approaches to source localization have demonstrated promising results in addressing reverberation. Even with large data volumes, the number of labels available for supervised learning in such environments is usually small. This challenge has recently been addressed using semi-supervised learning (SSL) based on deep generative modeling with variational autoencoders. A problem with ML approaches is they often ignore the intuitions from conventional signal processing approaches. We present a hybrid approach to ML-based source localization, which uses both SSL and conventional, analytic signal processing approaches to obtain source location estimates. An SSL approach is developed which accounts for the residual between analytic source location estimates true locations. Thus, the approach can exploit both labelled and unlabeled data, as well as analytic source location intuition, to provide better localization than either approach in isolation.
更多查看译文
关键词
Source localization,semi-supervised learning,generative modeling,deep learning
AI 理解论文
溯源树
样例
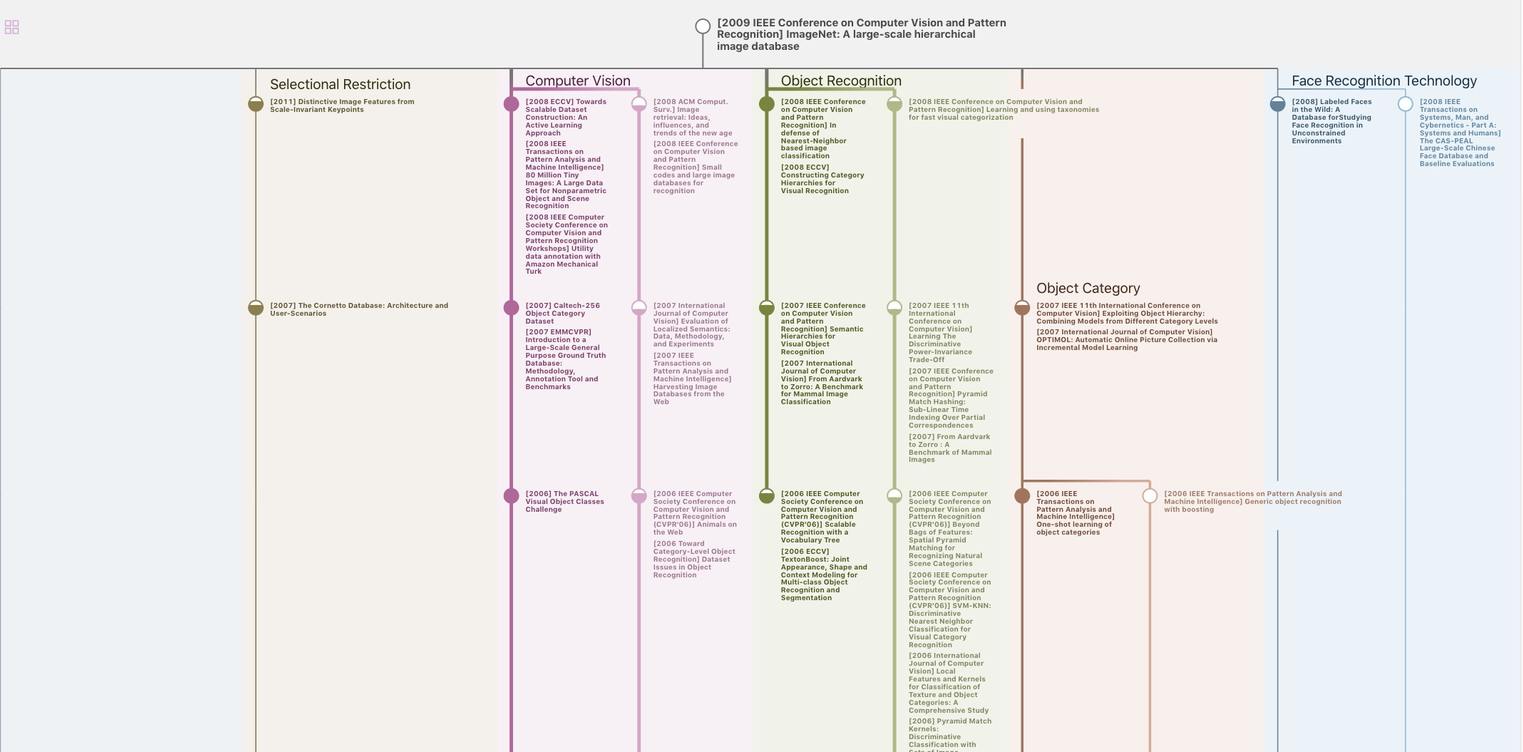
生成溯源树,研究论文发展脉络
Chat Paper
正在生成论文摘要