Proximal-Based Adaptive Simulated Annealing for Global Optimization
IEEE International Conference on Acoustics, Speech, and Signal Processing (ICASSP)(2022)
摘要
Simulated annealing (SA) is a widely used approach to solve global optimization problems in signal processing. The initial non-convex problem is recast as the exploration of a sequence of Boltzmann probability distributions, which are increasingly harder to sample from. They are parametrized by a temperature that is iteratively decreased, following the so-called cooling schedule. Convergence results of SA methods usually require the cooling schedule to be set a priori with slow decay. In this work, we introduce a new SA approach that selects the cooling schedule on the fly. To do so, each Boltzmann distribution is approximated by a proposal density, which is also sequentially adapted. Starting from a variational formulation of the problem of joint temperature and proposal adaptation, we derive an alternating Bregman proximal algorithm to minimize the resulting cost, obtaining the sequence of Boltzmann distributions and proposals. Numerical experiments in an idealized setting illustrate the potential of our method compared with state-of-the-art SA algorithms.
更多查看译文
关键词
Global optimization,simulated annealing,adaptive cooling schedule,Kullback-Leibler divergence,alternating minimization
AI 理解论文
溯源树
样例
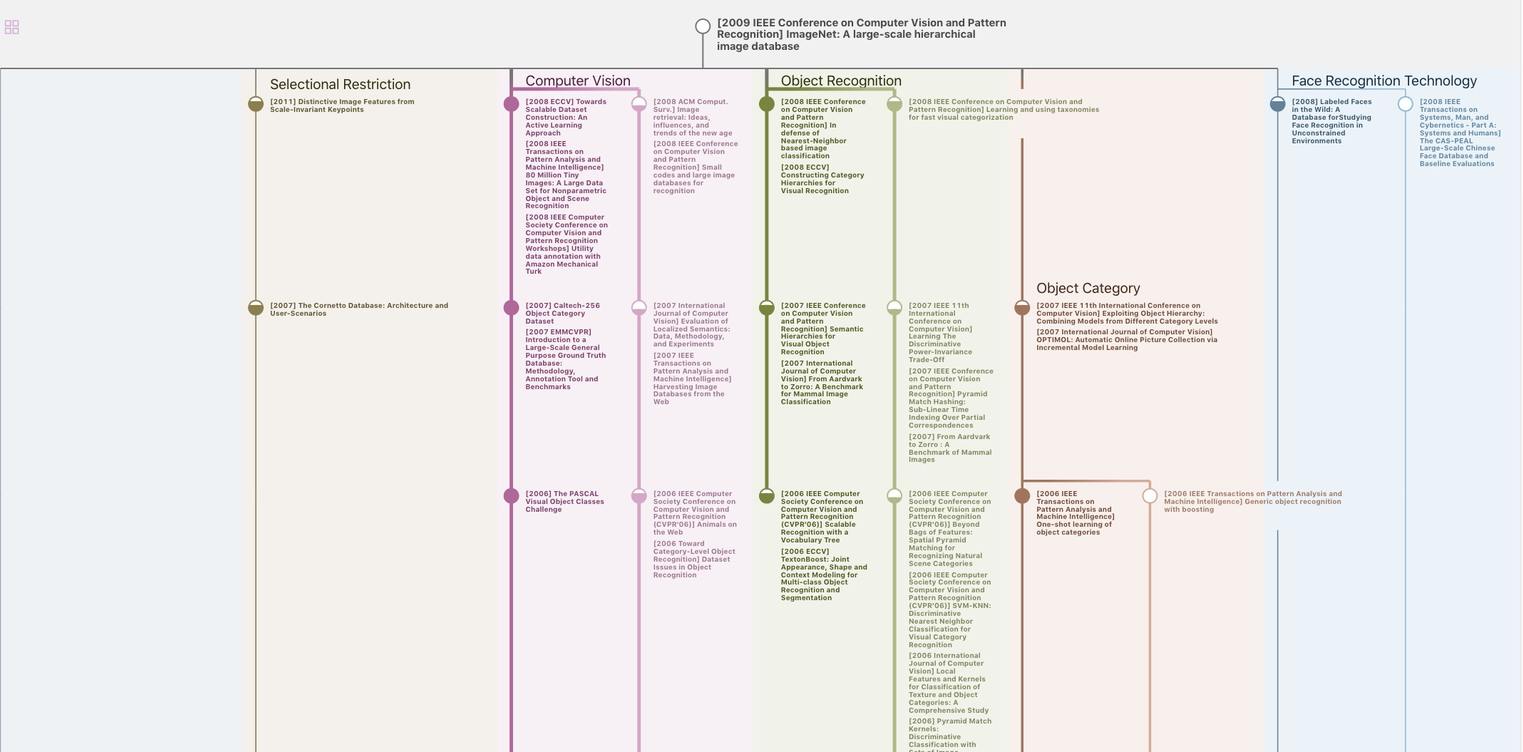
生成溯源树,研究论文发展脉络
Chat Paper
正在生成论文摘要