Joint Temporal Convolutional Networks and Adversarial Discriminative Domain Adaptation for EEG-Based Cross-Subject Emotion Recognition
IEEE International Conference on Acoustics, Speech, and Signal Processing (ICASSP)(2022)
摘要
Cross-subject emotion recognition is one of the most challenging tasks in electroencephalogram (EEG)-based emotion recognition. To guarantee the constancy of feature representations across domains and to eliminate differences between domains, we explored the feasibility of combining temporal convolutional networks (TCNs) and adversarial discriminative domain adaptation (ADDA) algorithms in solving the problem of domain shift in EEG-based crosssubject emotion recognition. In light of EEG signals that have specific temporal properties, we chose the temporal model TCN as the feature encoder. To verify the validity of the proposed method, we conducted experiments on two public datasets: DEAP and DREAMER. The experimental results show that for the leave-one-subject-out evaluation, average accuracies of 64.33% (valence) and 63.25% (arousal) were obtained on the DEAP dataset, and average accuracies of 66.56% (valence) and 63.69% (arousal) were achieved on the DREAMER dataset. Extensive experiments demonstrate that our method for EEG-based cross- subject emotion recognition is effective.
更多查看译文
关键词
Emotion recognition,EEG,Temporal convolutional network (TCN),Adversarial discriminative domain adaptation (ADDA)
AI 理解论文
溯源树
样例
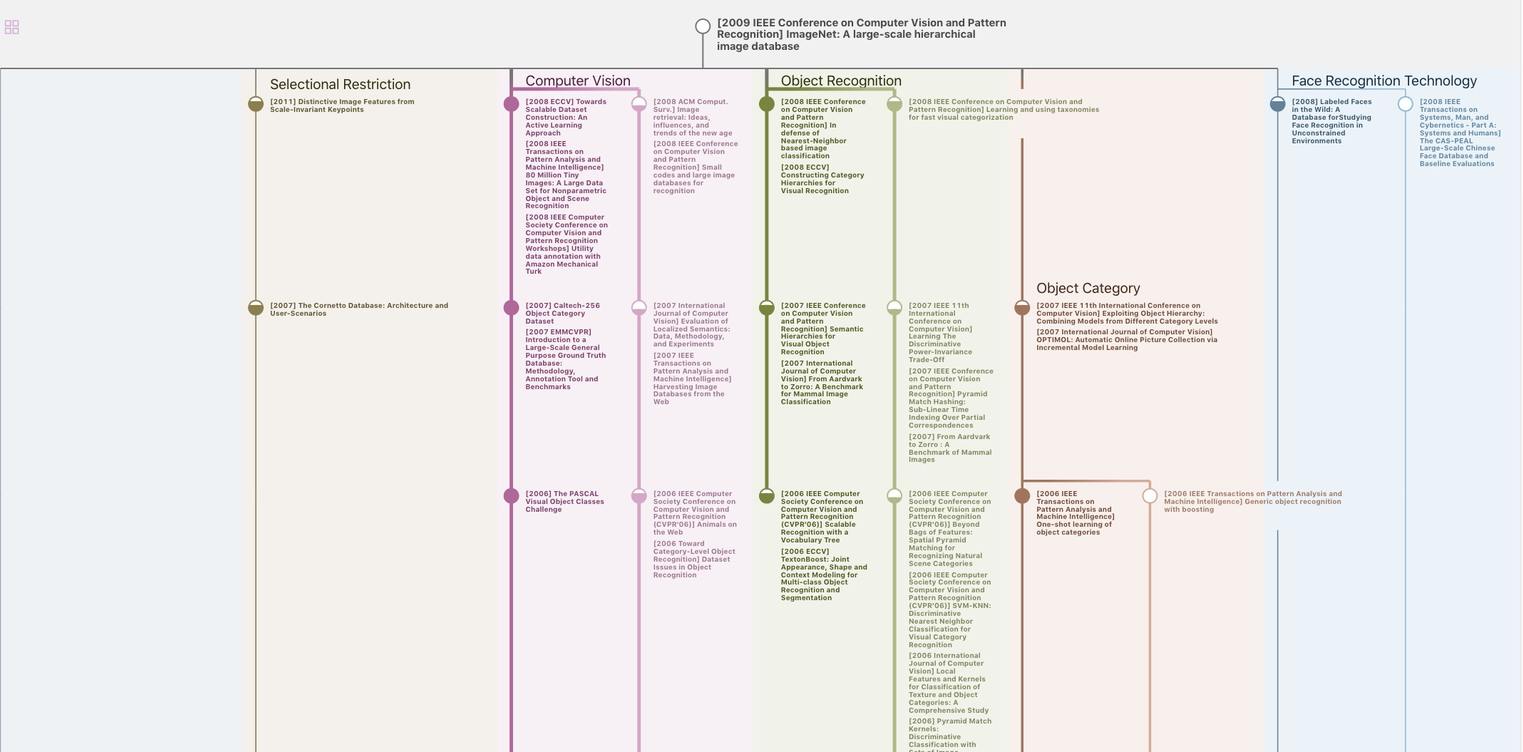
生成溯源树,研究论文发展脉络
Chat Paper
正在生成论文摘要