Coarse-To-Fine Unsupervised Change Detection for Remote Sensing Images Via Object-Based MRF and Inception UNET
IEEE International Conference on Acoustics, Speech, and Signal Processing (ICASSP)(2022)
摘要
With the rapid development of various satellite sensor techniques, remote sensing imagery has been an important source of data in change detection applications. This paper aims to propose an unsupervised change detection method based on Object-based Markov Random Filed (OMRF) and Inception UNet (IUNet). Our method first utilizes a difference image (DI) obtained from two bi-temporal images as the initial feature, and proposes the OMRF algorithm based on homogeneous region to pre-classify the DI thus derive the coarse change map. The IUNet is then constructed to extract the points with high confidence from the coarse change map for training. Eventually, the trained model is fed to classify the original feature, then the final change map is obtained. Experimental results indicate that our method yields great detection results even without supervision.
更多查看译文
关键词
change detection,object-based markov random filed,coarse-to-fine model,unsupervised learning
AI 理解论文
溯源树
样例
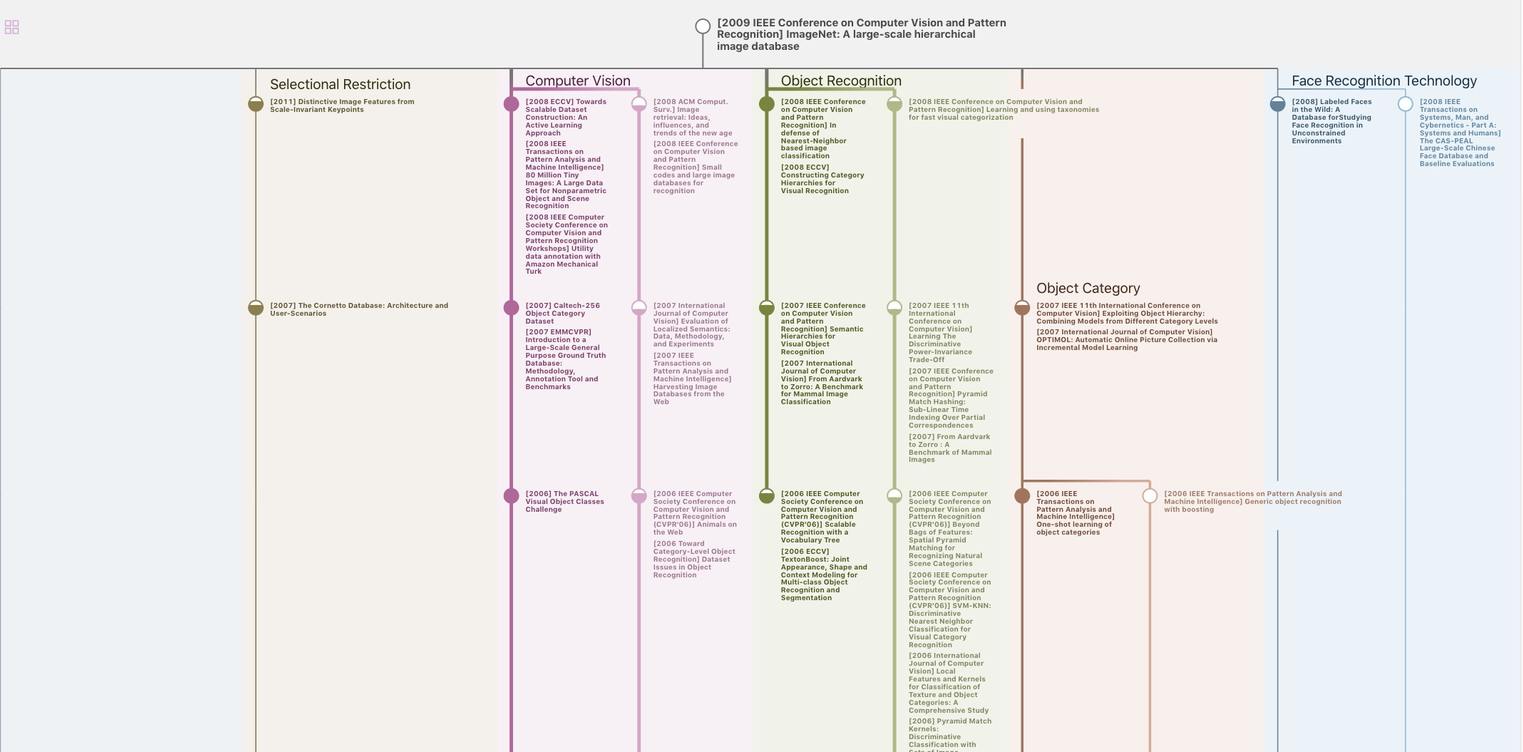
生成溯源树,研究论文发展脉络
Chat Paper
正在生成论文摘要