Text-Image De-Contextualization Detection Using Vision-Language Models
IEEE International Conference on Acoustics, Speech, and Signal Processing (ICASSP)(2022)
摘要
Text-image de-contextualization, which uses inconsistent image-text pairs, is an emerging form of misinformation and drawing increasing attention due to the great threat to information authenticity. With real content but semantic mismatch in multiple modalities, the detection of de-contextualization is a challenging problem in media forensics. Inspired by the recent advances in vision-language models with powerful relationship learning between images and texts, we leverage the vision-language models to the media de-contextualization detection task. Two popular models, namely CLIP and VinVL, are evaluated and compared on several news and social media datasets to show their performance in detecting image-text inconsistency in de-contextualization. We also summarize interesting observations and shed lights to the use of vision-language models in de-contextualization detection.
更多查看译文
关键词
de-contextualization,online misinformation,out-of-text detection,text-image inconsistency
AI 理解论文
溯源树
样例
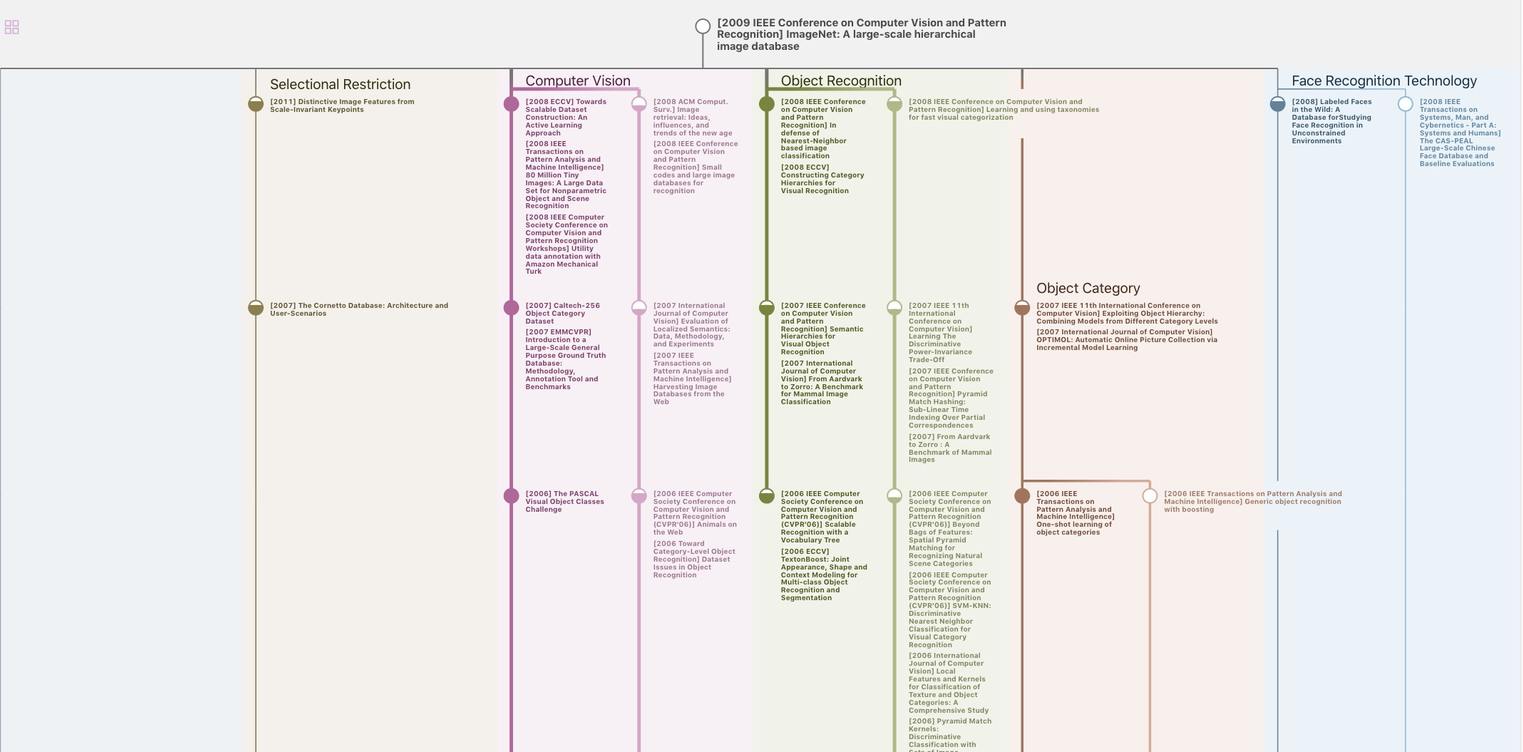
生成溯源树,研究论文发展脉络
Chat Paper
正在生成论文摘要