Linear-Time Sampling on Signed Graphs Via Gershgorin Disc Perfect Alignment
IEEE International Conference on Acoustics, Speech, and Signal Processing (ICASSP)(2022)
摘要
In graph signal processing (GSP), an appropriate underlying graph encodes pairwise (anti-)correlations of targeted discrete signals as edge weights. However, existing fast graph sampling schemes are designed and tested for positive graphs describing only positive correlations. In this paper, we show that for datasets with inherent strong anti-correlations, a suitable graph structure is instead a signed graph with both positive and negative edge weights, and in response, we propose a linear-time signed graph sampling method. Specifically, given an empirical covariance data matrix (C) over bar, we first employ graphical lasso to learn a sparse inverse matrix L, interpreted as a generalized graph Laplacian for signed graph G. We then propose a fast signed graph sampling scheme containing three steps: i) augment G to a balanced graph G(B) , ii) align all Gershgorin disc left-ends of corresponding Laplacian L-B at smallest eigenvalue lambda(min) (L-B) via similarity transform L-p = SLBS-1, leveraging a recent linear algebra theorem called Gershgorin disc perfect alignment (GDPA), and iii) perform sampling on L-p using a previous fast Gershgorin disc alignment sampling scheme (GDAS). Experimental results show that our signed graph sampling method outperformed existing fast sampling schemes noticeably on two political voting datasets.
更多查看译文
关键词
Graph sampling,Gershgorin circle theorem
AI 理解论文
溯源树
样例
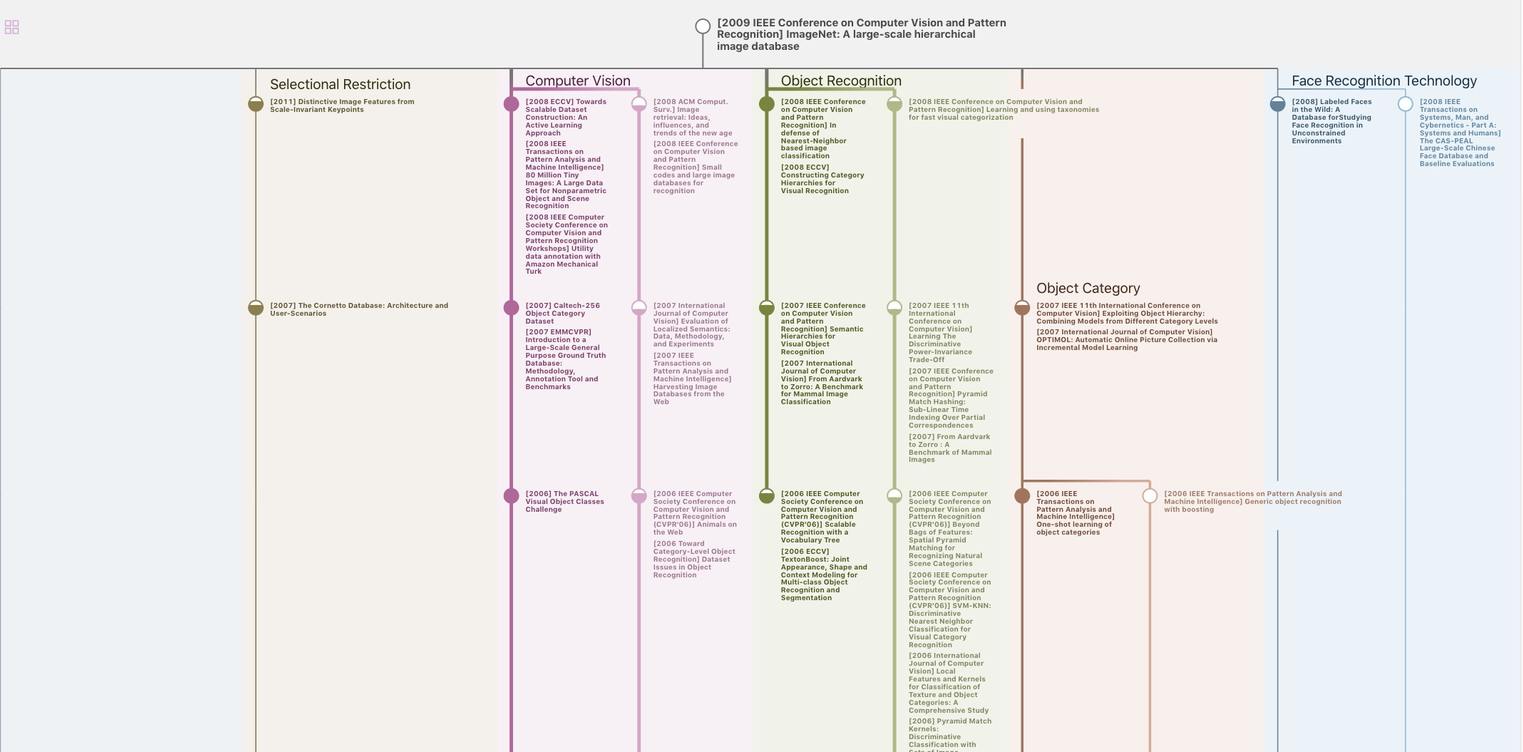
生成溯源树,研究论文发展脉络
Chat Paper
正在生成论文摘要