Domain-Agnostic Meta-Learning for Cross-Domain Few-Shot Classification
ICASSP 2022 - 2022 IEEE International Conference on Acoustics, Speech and Signal Processing (ICASSP)(2022)
摘要
Few-shot classification requires one to classify instances of novel classes, given only a few examples of each class. Although promising meta-learning methods have been proposed recently, there is no guarantee that existing solutions would generalize to novel classes from an unseen domain. In this paper, we tackle the challenging task of cross-domain few-shot classification and propose Domain-Agnostic Meta-Learning (DAML) algorithm. Our DAML, serving as an optimization strategy, learns to adapt the model to novel classes in both seen and unseen domains by data sampled from multiple domains with desirable task settings. In our experiments, we apply DAML on three popular metric-based models under cross-domain settings. Experiments on several benchmarks (mini-ImageNet, CUB, Cars, Places, Plantae and META-DATASET) show that DAML significantly improves the generalization ability of learning models, and addresses cross-domain few-shot classification with promising results.
更多查看译文
关键词
Meta-learning,Few-shot classification
AI 理解论文
溯源树
样例
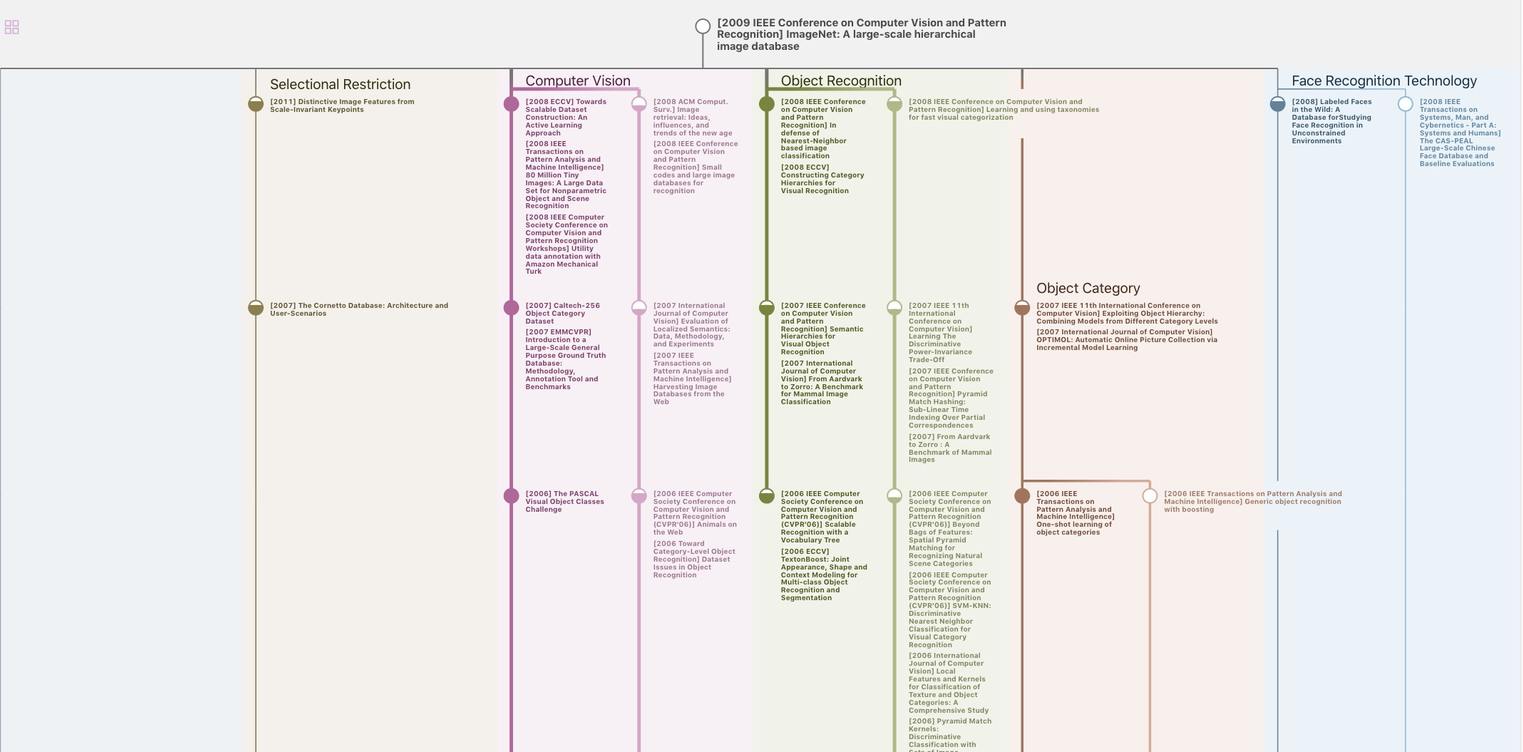
生成溯源树,研究论文发展脉络
Chat Paper
正在生成论文摘要