Modeling Human Memory in Multi-Object Tracking with Transformers
IEEE International Conference on Acoustics, Speech, and Signal Processing (ICASSP)(2022)
Abstract
When tracking objects, humans rely on a memory mechanism, memorize the track of an object then look for it in the current scene. In this paper, we propose Memory-based Multi-object Tracking with Transformers (MMTT) to mimic human behavior in multi-object tracking. Unlike Re-ID-based methods, MMTT solves multi-object tracking in an explicit way, with a Track Encoder to extract track memory, a Detection Encoder to extract detection interactions, and a Memory Decoder to simulate the "look" process. The design of MMTT has the ability to model both spatial and temporal information of a single track. We evaluate on commonly used MOT datasets and the experimental results demonstrate its superior effectiveness. We hope this paper can provide a novel direction for the MOT task. The code and models will be made publicly available upon acceptance.
MoreTranslated text
Key words
Multi-object tracking,Transformer,Human memory modeling,Deep learning
AI Read Science
Must-Reading Tree
Example
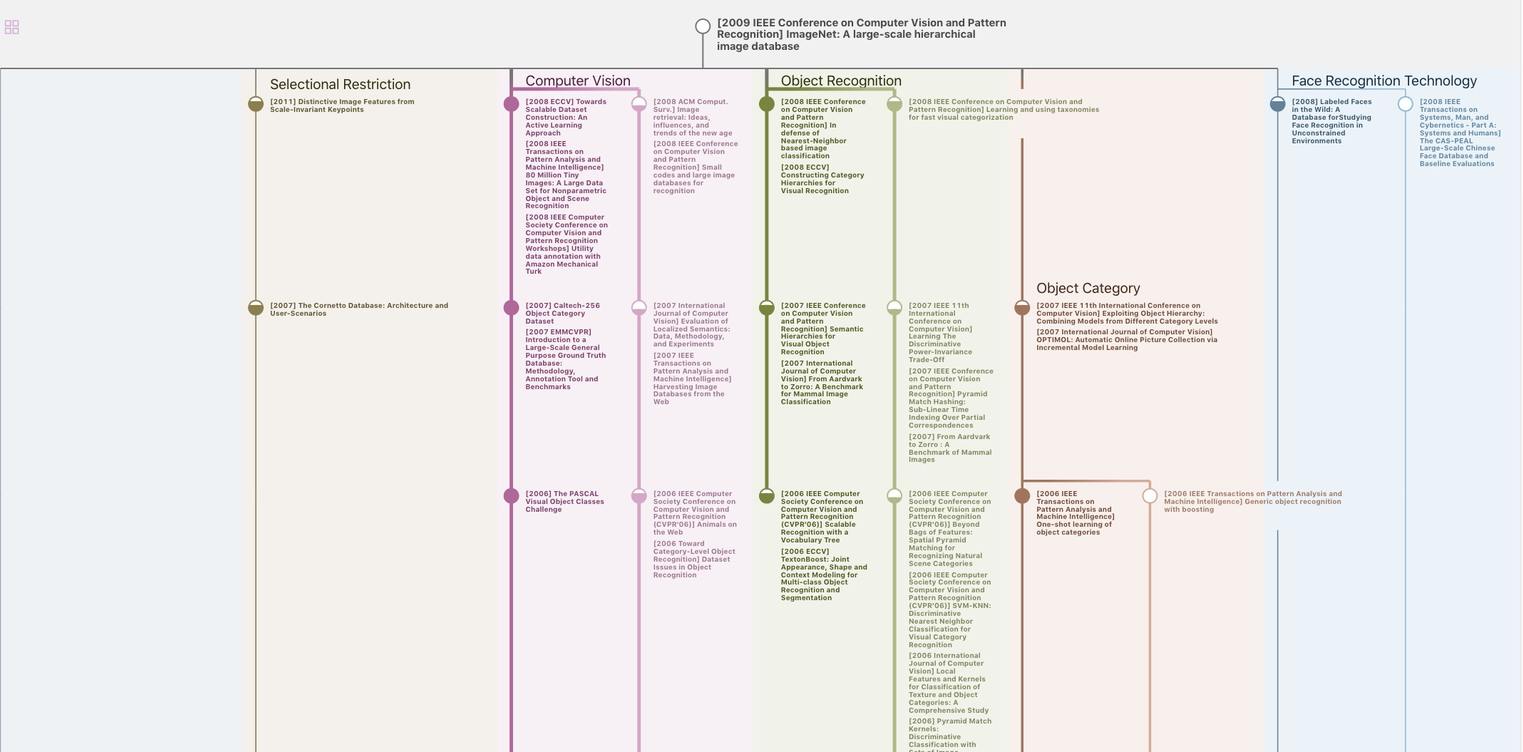
Generate MRT to find the research sequence of this paper
Chat Paper
Summary is being generated by the instructions you defined