Improving Spoken Language Understanding by Enhancing Text Representation
IEEE International Conference on Acoustics, Speech, and Signal Processing (ICASSP)(2022)
摘要
Language Model (LM) which is commonly trained on a large corpora has been proven the robustness and effectiveness for tasks of Natural Language Understanding (NLU) in many applications such as virtual assistant or recommendation system. These applications normally receive outputs of automatic speech recognition (ASR) module as spoken form inputs which generally lack both lexical and syntactic information. Pre-trained language models, for example BERT [1] or XLM-RoBERTa [2], which are often pre-trained on written form corpora perform decreased performance on NLU tasks with spoken form inputs. In this paper, we propose a novel model to train a language model namely CapuBERT that is able to deal with spoken form input from ASR module. The experimental results show that the proposed model achieves state-of-the-art results on several NLU tasks included Part-of-speech tagging, Named-entity recognition and Chunking in English, German, and Vietnamese languages.
更多查看译文
关键词
Spoken Language Understanding,BERT,CapuBERT,ASR
AI 理解论文
溯源树
样例
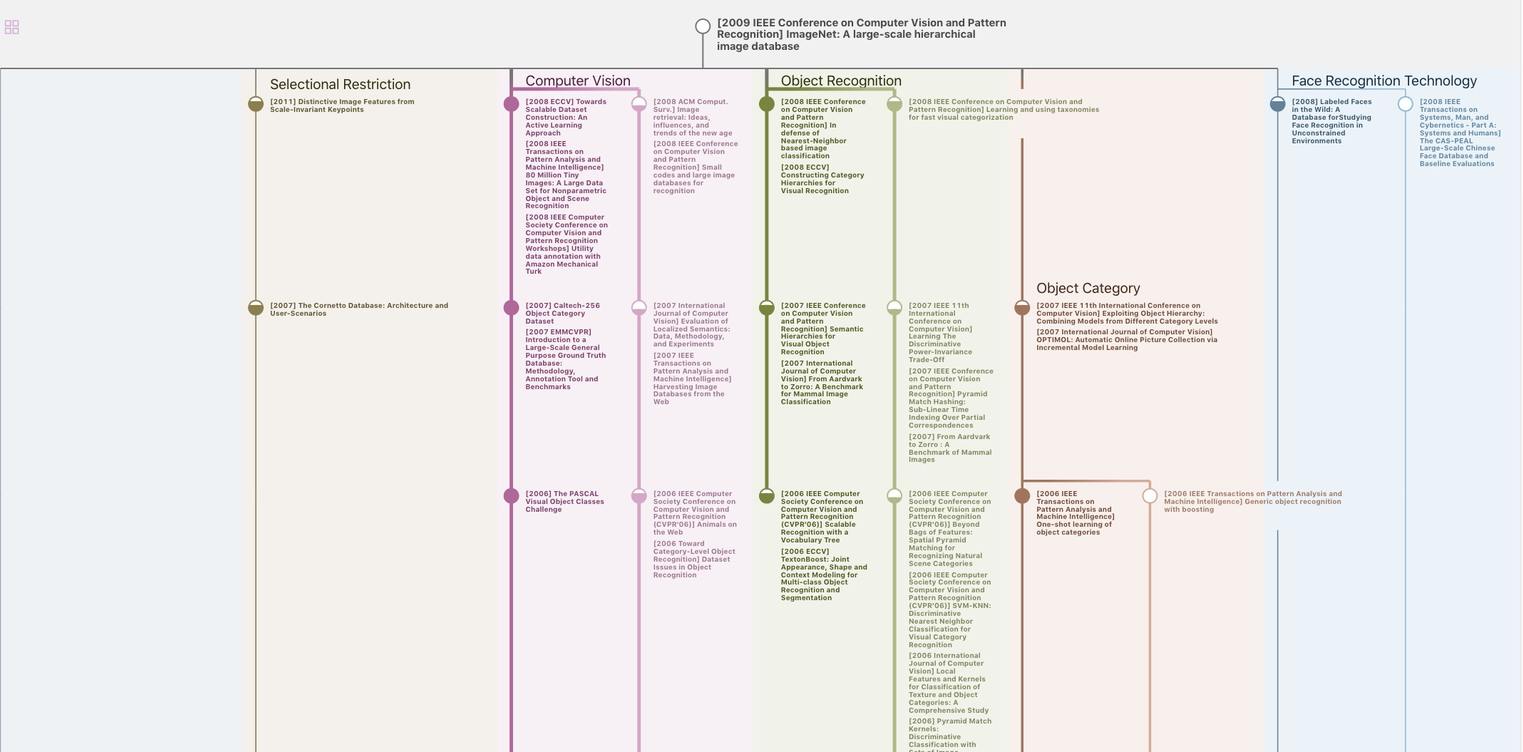
生成溯源树,研究论文发展脉络
Chat Paper
正在生成论文摘要