Graph-based Transform based on 3D Convolutional Neural Network for Intra-Prediction of Imaging Data
2022 Data Compression Conference (DCC)(2022)
摘要
This paper presents a novel class of Graph-based Transform based on 3D convolutional neural networks (GBT-CNN) within the context of block-based predictive transform coding of imaging data. The proposed GBT-CNN uses a 3D convolutional neural network (3D-CNN) to predict the graph information needed to compute the transform and its inverse, thus reducing the signalling cost to reconstruct the data after transformation. The GBT-CNN outperforms the DCT and DCT /DST, which are commonly employed in current video codecs, in terms of the percentage of energy preserved by a subset of transform coefficients, the mean squared error of the reconstructed data, and the transform coding gain according to evaluations on several video frames and medical images.
更多查看译文
关键词
Graph-Based Transforms,Convolutional Neural Network,Deep Learning,KLT,DCT,Coding Gain,HEVC,VVC,Video Coding,Video Compression
AI 理解论文
溯源树
样例
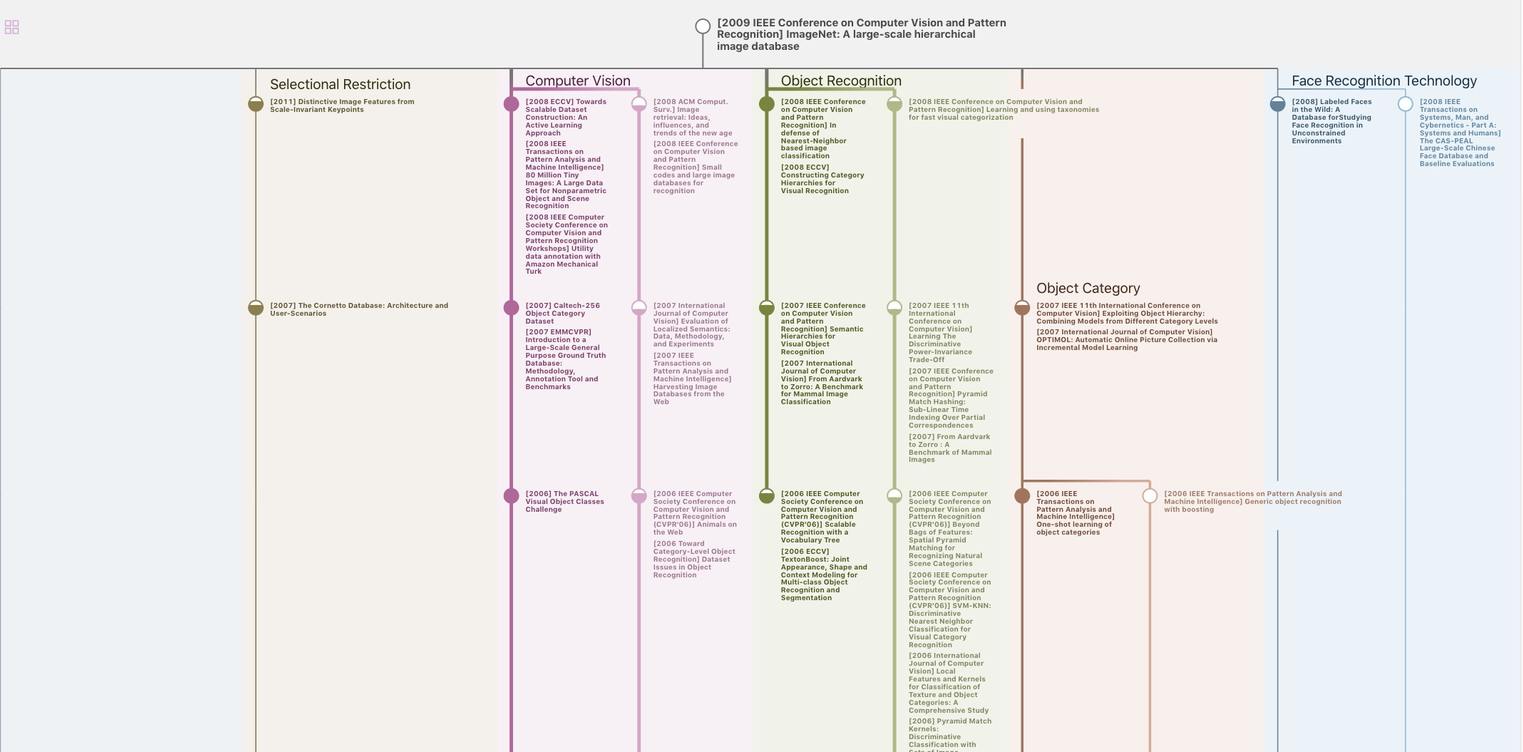
生成溯源树,研究论文发展脉络
Chat Paper
正在生成论文摘要