Anomaly detection to improve security of big data analytics
ACM International Conference on Computing Frontiers (CF)(2022)
摘要
Big data analytics largely rely on data. Because of their central role, it is fundamental to ensure the security and correctness of data used in these applications. Anomaly detection could help to increase the security of big data analytics applications. However, these applications are very diverse both for the properties of the data analyzed and for the computations to be carried out on them. As a result, the selection of the most appropriate anomaly detection method is a challenging and time consuming task for designers. Hierarchical Temporal Memory (HTM) is as an anomaly detection technique sufficiently generic to achieve satisfactory performance on a wide range of applications, thus suitable to ease the burden of selecting the anomaly detection method. To confirm this, in this paper we explore the performance of HTM on a dataset used for air quality prediction. Our preliminary results showthat HTM achieves excellent performance when compared to other popular anomaly detection methods.
更多查看译文
AI 理解论文
溯源树
样例
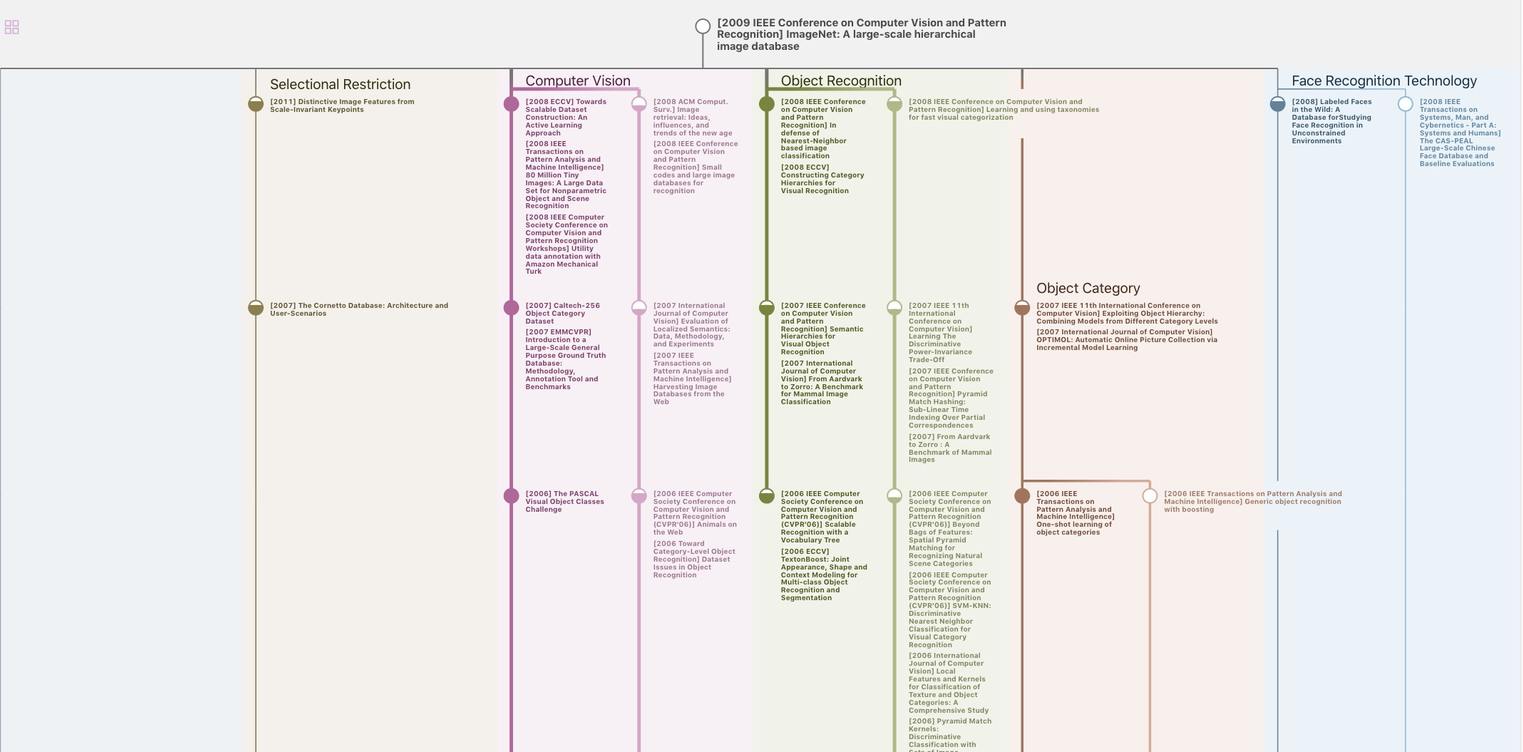
生成溯源树,研究论文发展脉络
Chat Paper
正在生成论文摘要