Real-Valued Group Testing for Quantitative Molecular Assays
Annual International Conference on Research in Computational Molecular Biology (RECOMB)(2022)
摘要
Combinatorial group testing and compressed sensing both focus on recovering a sparse vector of dimensionality n from a much smaller number m < n of measurements. In the first approach, the problem is defined over the Boolean field – the goal is to recover a Boolean vector and measurements are Boolean; in the second approach, the unknown vector and the measurements are over the reals. Here, we focus on real-valued group testing setting that more closely fits modern testing protocols relying on quantitative measurements, such as qPCR, where the goal is recovery of a sparse, Boolean vector and the pooling matrix needs to be Boolean and sparse, but the unknown input signal vector and the measurement outcomes are nonnegative reals, and the matrix algebra implied in the test protocol is over the reals. With the recent renewed interest in group testing, focus has been on quantitative measurements resulting from qPCR, but the method proposed for sample pooling were based on matrices designed with Boolean measurements in mind. Here, we investigate constructing pooling matrices dedicated for the real-valued group testing. We provide conditions for pooling matrices to guarantee unambiguous decoding of positives in this setting. We also show a deterministic algorithm for constructing matrices meeting the proposed condition, for small matrix sizes that can be implemented using a laboratory robot. Using simulated data, we show that the proposed approach leads to matrices that can be applied for higher positivity rates than combinatorial group testing matrices considered for viral testing previously. We also validate the approach through wet lab experiments involving SARS-CoV-2 nasopharyngeal swab samples.
更多查看译文
关键词
quantitative molecular assays,group testing,real-valued
AI 理解论文
溯源树
样例
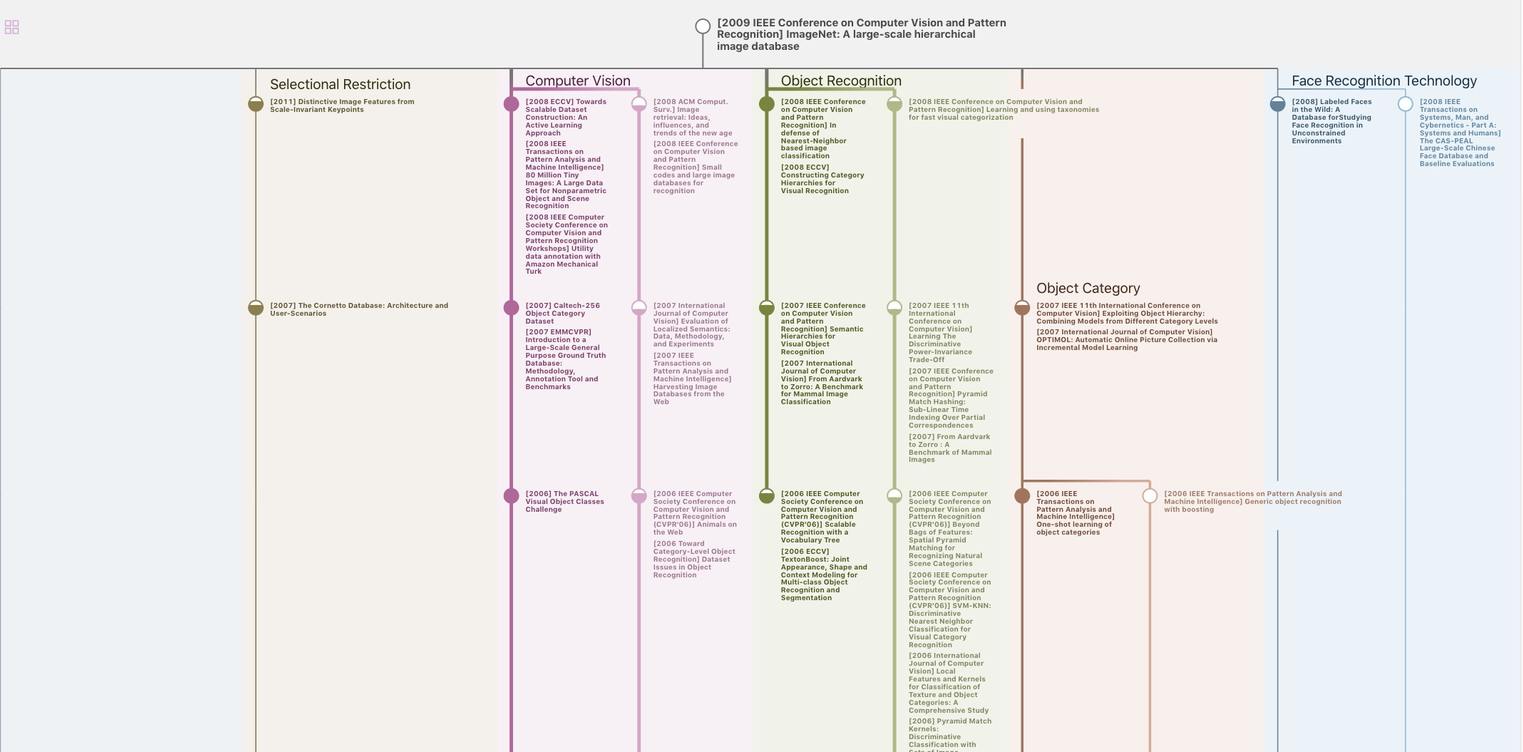
生成溯源树,研究论文发展脉络
Chat Paper
正在生成论文摘要