Data Drift in DL: Lessons Learned from Encrypted Traffic Classification
2022 IFIP Networking Conference (IFIP Networking)(2022)
摘要
Deep learning models have shown to achieve high performance in encrypted traffic classification. However, when it comes to production use, multiple factors challenge the performance of these models. The emergence of new network traffic protocols, especially at the application-layer, as well as updates to previous protocols affect the patterns in input data, making the model's previously learned patterns obsolete. Furthermore, proposed model architectures are usually tested on datasets collected in controlled settings, which makes the reported performances unreliable for production use. In this paper, we study how the performances of two high-performing traffic classifiers change on multiple real-world datasets collected over the course of two years. We investigate the changes in traffic data patterns showing the extent to which these changes reduce the performance of the two models. Furthermore, we propose architectural adaptations to a flow time-series based traffic classifier, showing that they improve accuracy by 4.8%.
更多查看译文
关键词
Data Drift,Encrypted Traffic Classification,Deep Learning,Web Traffic,HTTP/2,QUIC
AI 理解论文
溯源树
样例
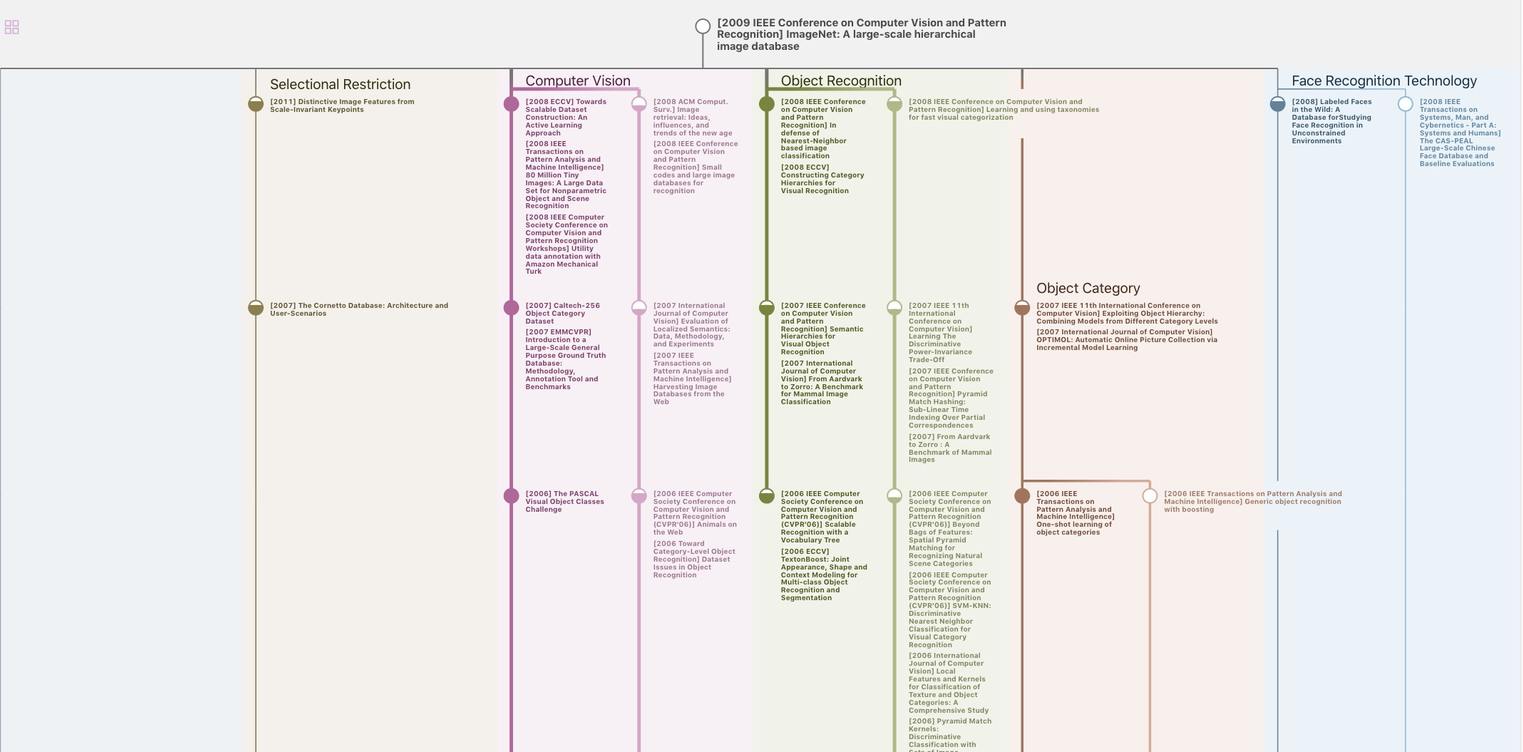
生成溯源树,研究论文发展脉络
Chat Paper
正在生成论文摘要