Accelerating Traffic Engineering in Segment Routing Networks: A Data-driven Approach
IEEE International Conference on Communications (ICC)(2022)
摘要
Segment routing (SR) is an emerging architecture that can benefit traffic engineering (TE). To solve TE in SR networks (we call it SR-TE), linear programming (LP) is often used. But LP methods proposed so far for SR-TE are computationally expensive thus do not scale well in practice. To achieve trade-off between performance and time, we can select a set of nodes as candidates for intermediate nodes to route all traffic instead of considering all the nodes. However, existing node selection methods are all rule-based and only pay attention to the structure of network topology without considering flows, so they are not flexible and may lead to poor performance. In this paper, we for the first time formulate node selection for SR-TE as a reinforcement learning (RL) task. When performing node selection, we consider the impact of both topology and traffic matrix. Also, a customized training algorithm for our task is proposed because existing RL algorithms can not be used directly. Performance evaluations on various real-world topologies and traffic matrices show that our method can achieve good TE performance with much less running time.
更多查看译文
关键词
Traffic Engineering,Segment Routing,Linear Programming,Reinforcement Learning
AI 理解论文
溯源树
样例
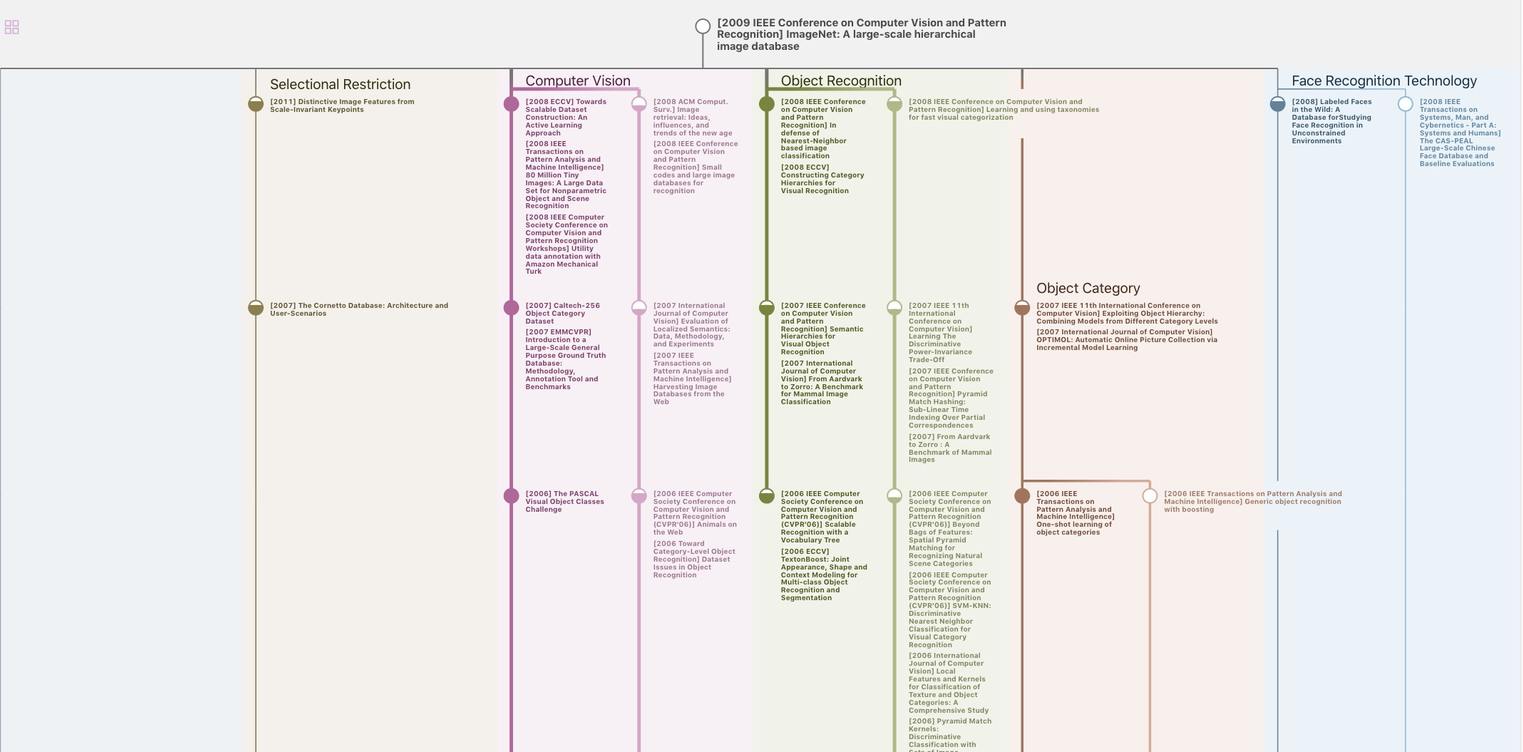
生成溯源树,研究论文发展脉络
Chat Paper
正在生成论文摘要