Auxiliary Local Variables for Improving Regularization/Prior Approach in Continual Learning
ADVANCES IN KNOWLEDGE DISCOVERY AND DATA MINING, PAKDD 2022, PT I(2022)
摘要
Regularization/prior approach emerges as one of the major directions in continual learning to help a neural network reduce forgetting the learned knowledge. This approach measures the importance of weights for previous tasks and then imposes a constraint on them in the current task without retraining on past data as well as extending the network architecture. However, regularization/prior-based methods face the problem in which weights can be moved intensively to the parameter region obtaining good performance for the current task but getting bad ones for previous tasks. In this paper, we present a novel solution in order to deal with this problem. Rather than using global variables as in the original methods, we add auxiliary local variables for each task that are considered as adjusting factors to suitably change the global ones to this task. As a result, the global variables can be preserved in a good region for all tasks to reduce the forgetting phenomenon. In particular, by imposing a variational distribution on the auxiliary local variables which are employed as multiplicative noise to the input of layers, we can achieve theoretical properties: Uncorrelated likelihoods, correlated pre-activation, and data-dependent regularization which are missing in the existing methods. These properties bring several benefits as follows: (1) Uncorrelated likelihoods between different data instances lead to reduce the high variance of stochastic gradient variational Bayes; (2) correlated pre-activation helps increase the representation ability for each task; and (3) data-dependent regularization guarantees to preserve the global variables in good region for all tasks. Our extensive experiments show that adding the local variables improves the performances of regularization/prior-based methods with significant magnitudes on several datasets. In particular, it makes several standard baselines approach SOTA results.
更多查看译文
关键词
Continual learning, Regularization/prior-based approach, Variational dropout, Local and global variables
AI 理解论文
溯源树
样例
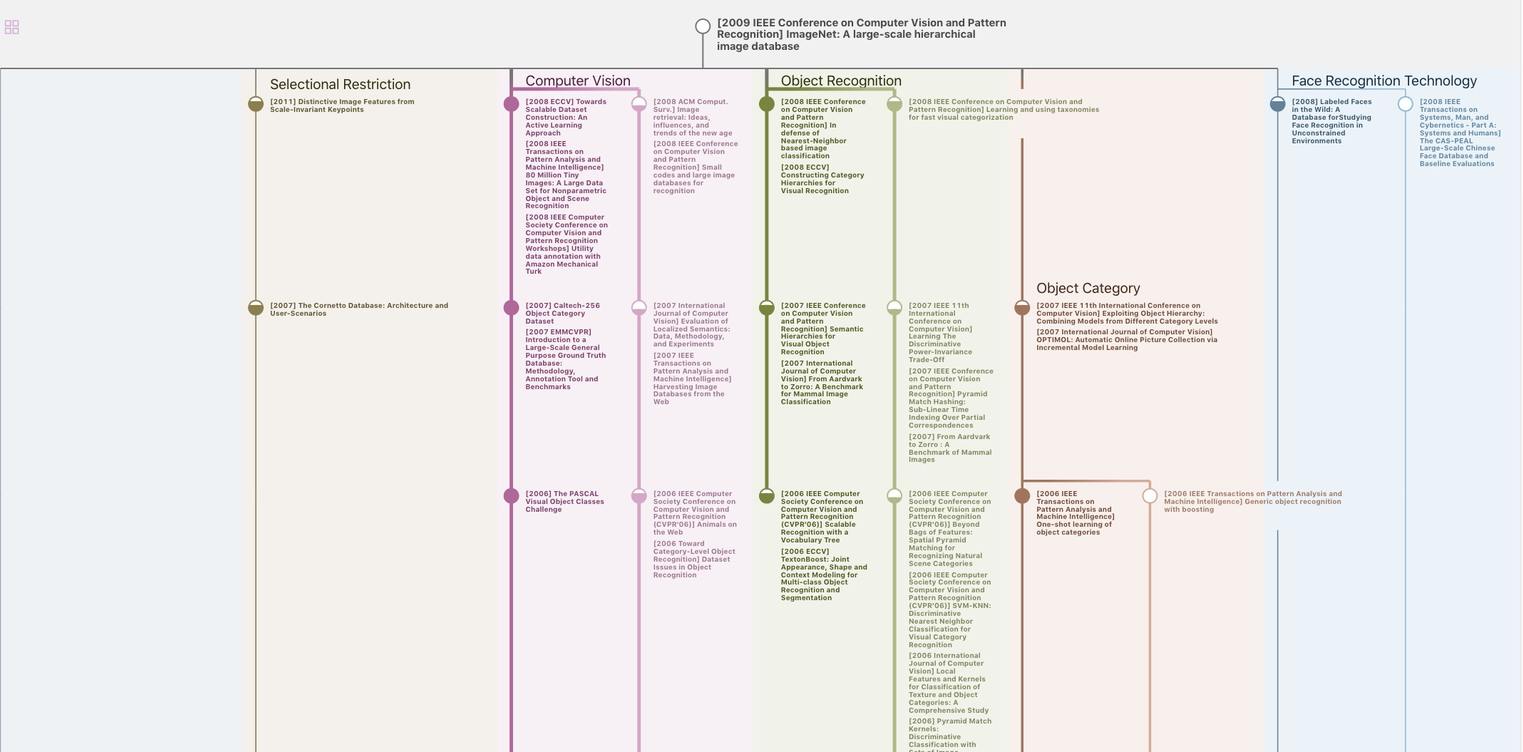
生成溯源树,研究论文发展脉络
Chat Paper
正在生成论文摘要