A New Notion of Individually Fair Clustering: α-Equitable k-Center
International Conference on Artificial Intelligence and Statistics (AISTATS)(2022)
摘要
Clustering is a fundamental problem in unsupervised machine learning, and due to its numerous societal implications fair variants of it have recently received significant attention. In this work we introduce a novel definition of individual fairness for clustering problems. Specifically, in our model, each point j has a set of other points S-j that it perceives as similar to itself, and it feels that it is being fairly treated if the quality of service it receives in the solution is alpha-close (in a multiplicative sense, for some given alpha >= 1) to that of the points in S-j. We begin our study by answering questions regarding the combinatorial structure of the problem, namely for what values of a the problem is well-defined, and what the behavior of the Price of Fairness (PoF) for it is. For the well-defined region of alpha, we provide efficient and easily-implementable approximation algorithms for the k-center objective, which in certain cases also enjoy bounded-PoF guarantees. We finally complement our analysis by an extensive suite of experiments that validates the effectiveness of our theoretical results.
更多查看译文
AI 理解论文
溯源树
样例
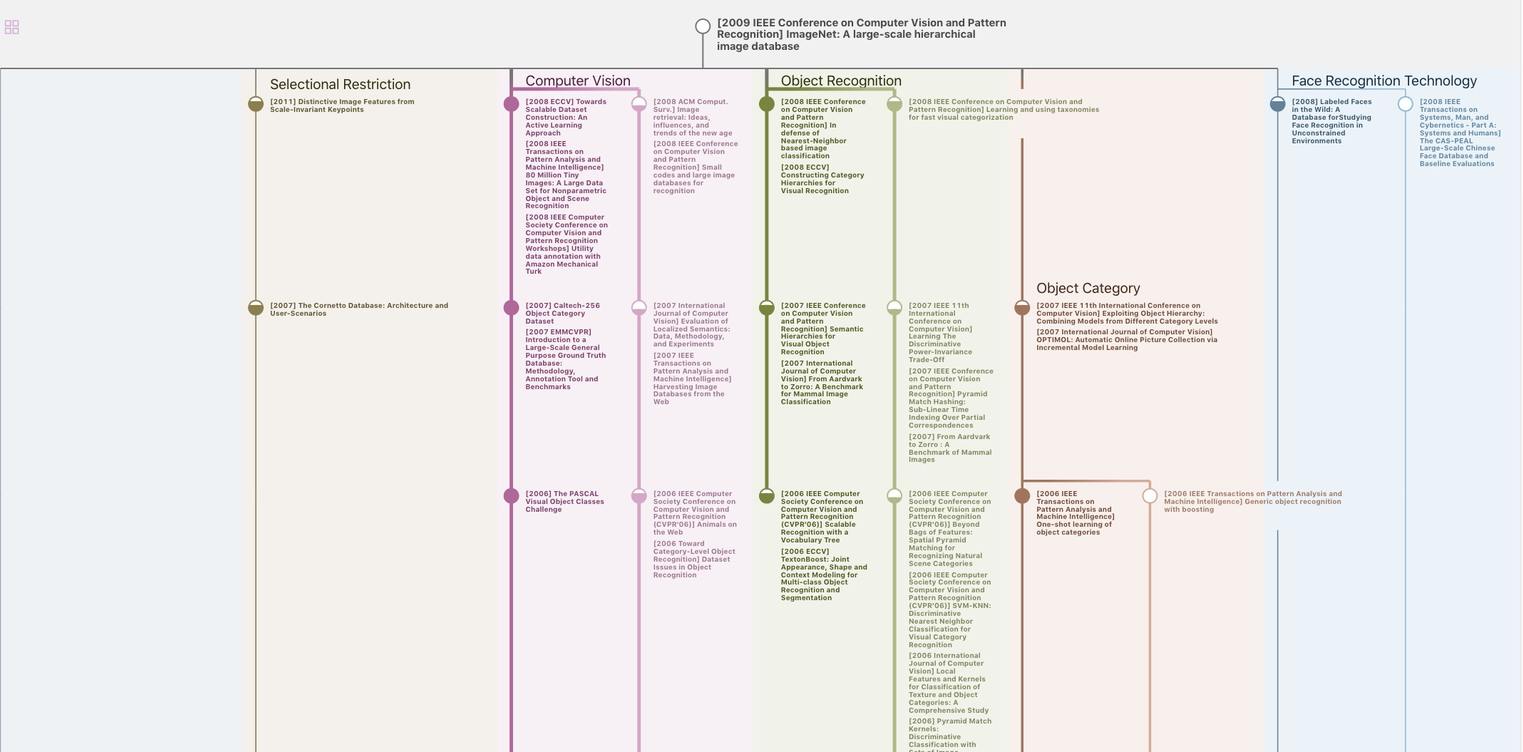
生成溯源树,研究论文发展脉络
Chat Paper
正在生成论文摘要