Induce Spoken Dialog Intents via Deep Unsupervised Context Contrastive Clustering
Conference of the International Speech Communication Association (INTERSPEECH)(2022)
摘要
Intent detection is one of most critical tasks in spoken language understanding. However, most systems could only identify a predefined set of intents, without covering a ubiquitous space of real-world semantics. Discovering new dialog intents with clustering to explore additional requests is crucial particularly in complex domains like customer support services. Leveraging the strong coherence between the user query utterance and their following contexts in the dialog, we present an effective intent induction approach with fine-tuning and clustering with contrastive learning. In particular, we first transform pretrained LMs into conversational encoders with in-domain dialogs. Then we conduct context-aware contrastive learning to reveal latent intent semantics via the coherence from dialog contexts. After obtaining the initial representations on both views of the query and their contexts, we propose a novel clustering method to iteratively refine the representation by minimizing semantic distances between pairs of utterances or contexts, under the same cluster assignment on the opposite view. The experimental results validate the robustness and versatility of our framework, which also achieves superior performances over competitive baselines without the label supervision.
更多查看译文
关键词
Spoken Language, Intent, Clustering, Context
AI 理解论文
溯源树
样例
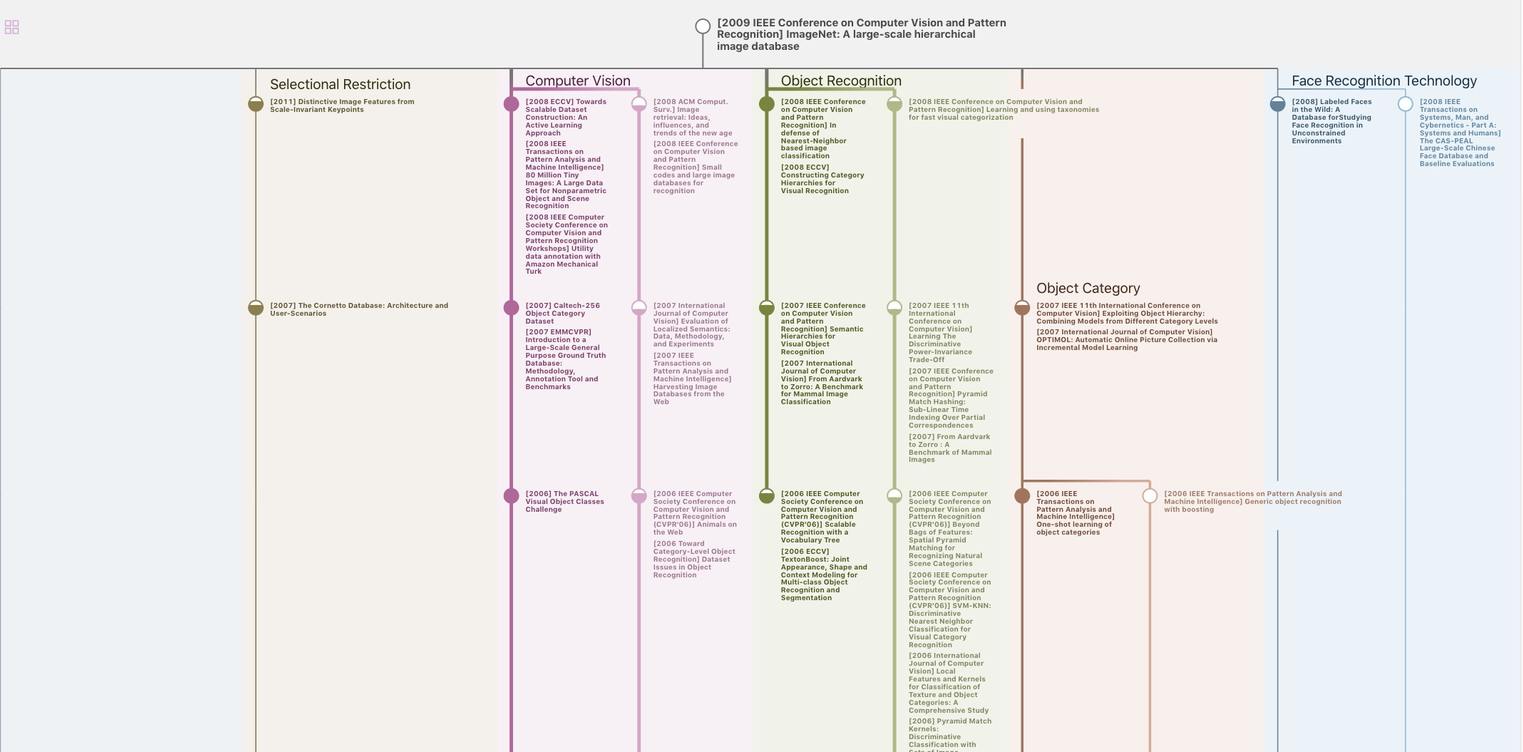
生成溯源树,研究论文发展脉络
Chat Paper
正在生成论文摘要