On Combining Global and Localized Self-Supervised Models of Speech
Conference of the International Speech Communication Association (INTERSPEECH)(2022)
摘要
Self supervised learning involves learning general-purpose representations that can be useful in a variety of downstream tasks. In this work, we study the application of speech-embeddings derived from popular self-supervised learning frameworks such as wav2vec-2.0 and HuBERT over four different speech-classification tasks such as sentiment classification, command detection, emotion classification and depression detection. We distinguish between and discuss self-supervised training tasks that induce localized and global features of speech based on their temporal granularity: noting that self-supervised representation learning frameworks based on the masked language-modeling objective - such as wav2vec-2.0 and HuBERT - induce localized embeddings, we define a self-supervised learning framework based on SimSiam for learning global features of speech. Through our evaluations, we find that these global representations are better suited for tasks such as depression detection and emotion classification while the localized embeddings of speech can be very useful in tasks such as speech-command detection; we also find that our proposed model outperforms TRILL - a popular model for learning global representations. Finally, we also propose and confirm empirically that combining the global and localized representations of speech helps obtain better performance across a range of downstream tasks than each of the individual embedding methods.
更多查看译文
关键词
speech,combining global,models,self-supervised
AI 理解论文
溯源树
样例
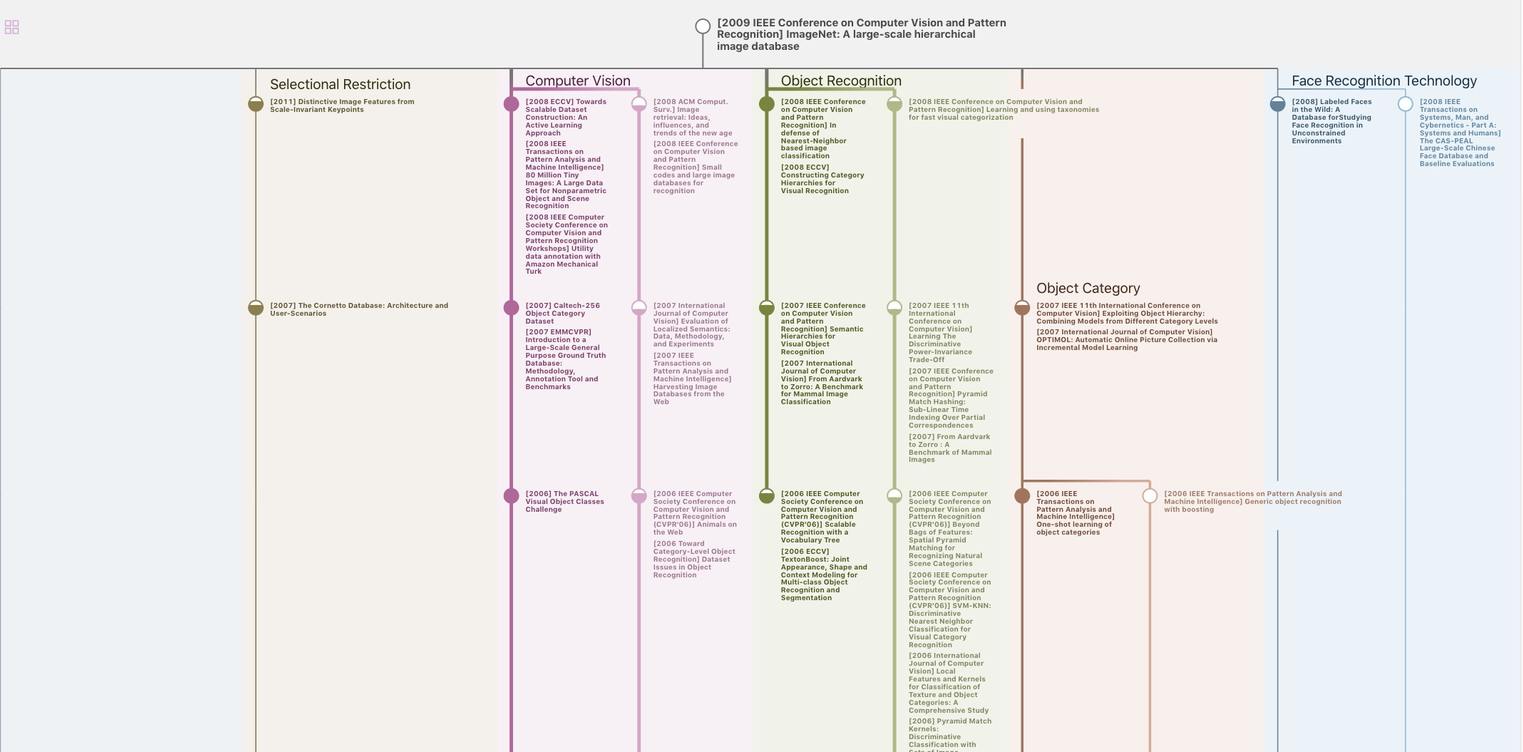
生成溯源树,研究论文发展脉络
Chat Paper
正在生成论文摘要