Can Humans Correct Errors From System? Investigating Error Tendencies in Speaker Identification Using Crowdsourcing
Conference of the International Speech Communication Association (INTERSPEECH)(2022)
摘要
An attempt was made to clarify the effectiveness of crowdsourcing on reducing errors in automatic speaker identification (ASID). It is possible to efficiently reduce errors by manually revalidating the unreliable results given by ASID systems. Ideally, errors should be corrected appropriately, and correct answers should not be miscorrected. In addition, a low false acceptance rate is desirable in authentication, but a high false rejection rate should be avoided from a usability viewpoint. It, however, is not certain that humans can achieve such an ideal SID, and in the case of crowdsourcing, the existence of malicious workers cannot be ignored. This study, therefore, investigates whether manual verification of error-prone inputs by crowd workers can reduce ASID errors and whether the resulting corrections are ideal. Experimental investigations on Amazon Mechanical Turk, in which 426 qualified workers identified 256 speech pairs from VoxCeleb data, demonstrated that crowdsourced verification can significantly reduce the number of false acceptances without increasing the number of false rejections compared to the results from the ASID system.
更多查看译文
关键词
Amazon Mechanical Turk, crowdsourcing, speaker identification, human-assisted pattern recognition
AI 理解论文
溯源树
样例
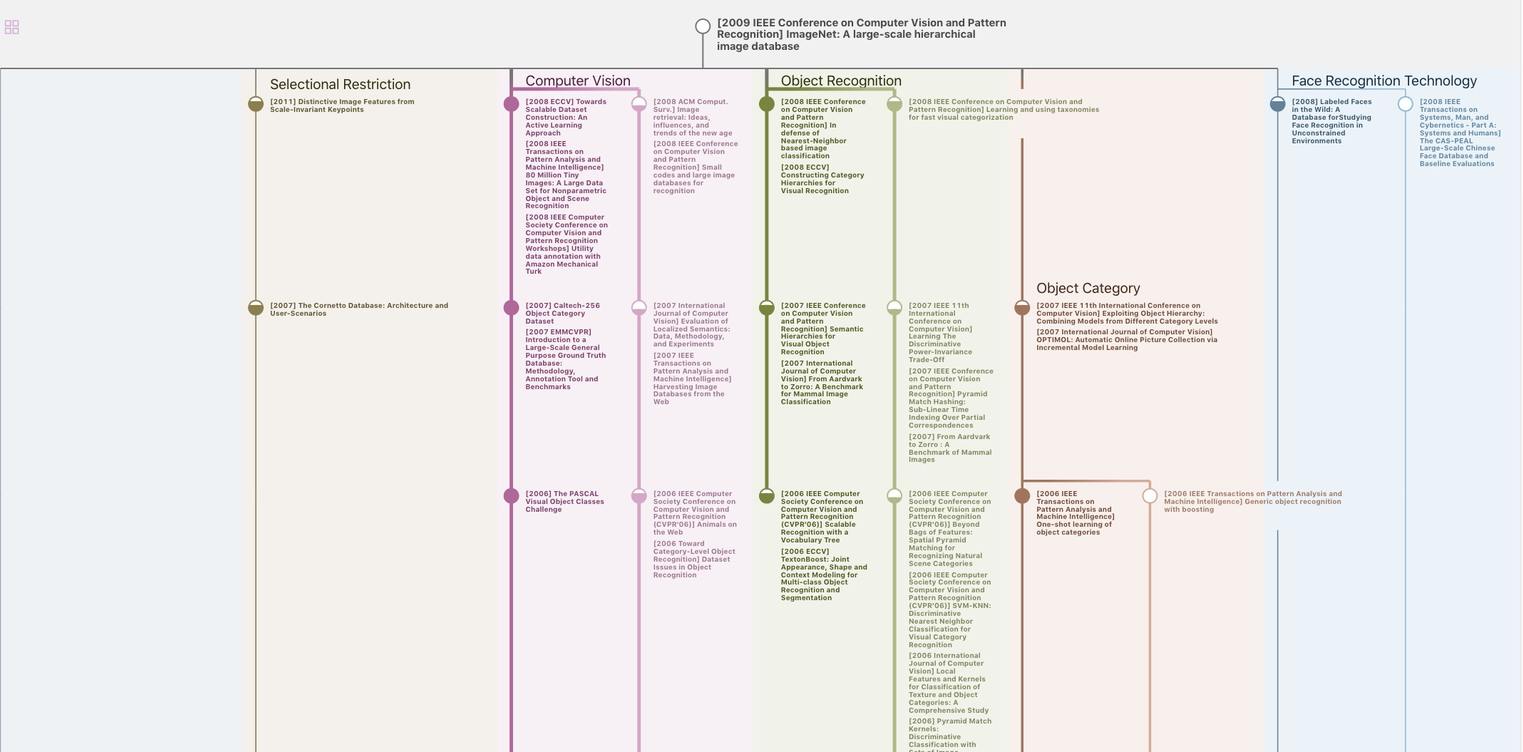
生成溯源树,研究论文发展脉络
Chat Paper
正在生成论文摘要