Conformer Space Neural Architecture Search for Multi-Task Audio Separation
Conference of the International Speech Communication Association (INTERSPEECH)(2022)
摘要
Multi-task audio source separation aims to separate the audios collected from the complex environment into three fixed types of signal sources. Existing methods like EAD-Conformer usually take a manually designed model to process the separation. These networks may be sub-optimal since it is hard for humans to train and test all possible architectures. Especially, it is natural to adopt different optimal sub-structures for decoding different types of signals, which, however, is very hard for humans to enumerate. In this paper, we quantitatively analyze the redundancy of the EAD-Conformer network and customize an effective and efficient search space. We propose an efficient K-path search method to search for the optimal architectures from the Conformer-based search space. We conduct a comprehensive search in terms of block numbers, head numbers, and channel numbers. Extensive experiments demonstrate that our searched architectures outperform existing methods in terms of efficiency and effectiveness.
更多查看译文
关键词
audio,separation,multi-task
AI 理解论文
溯源树
样例
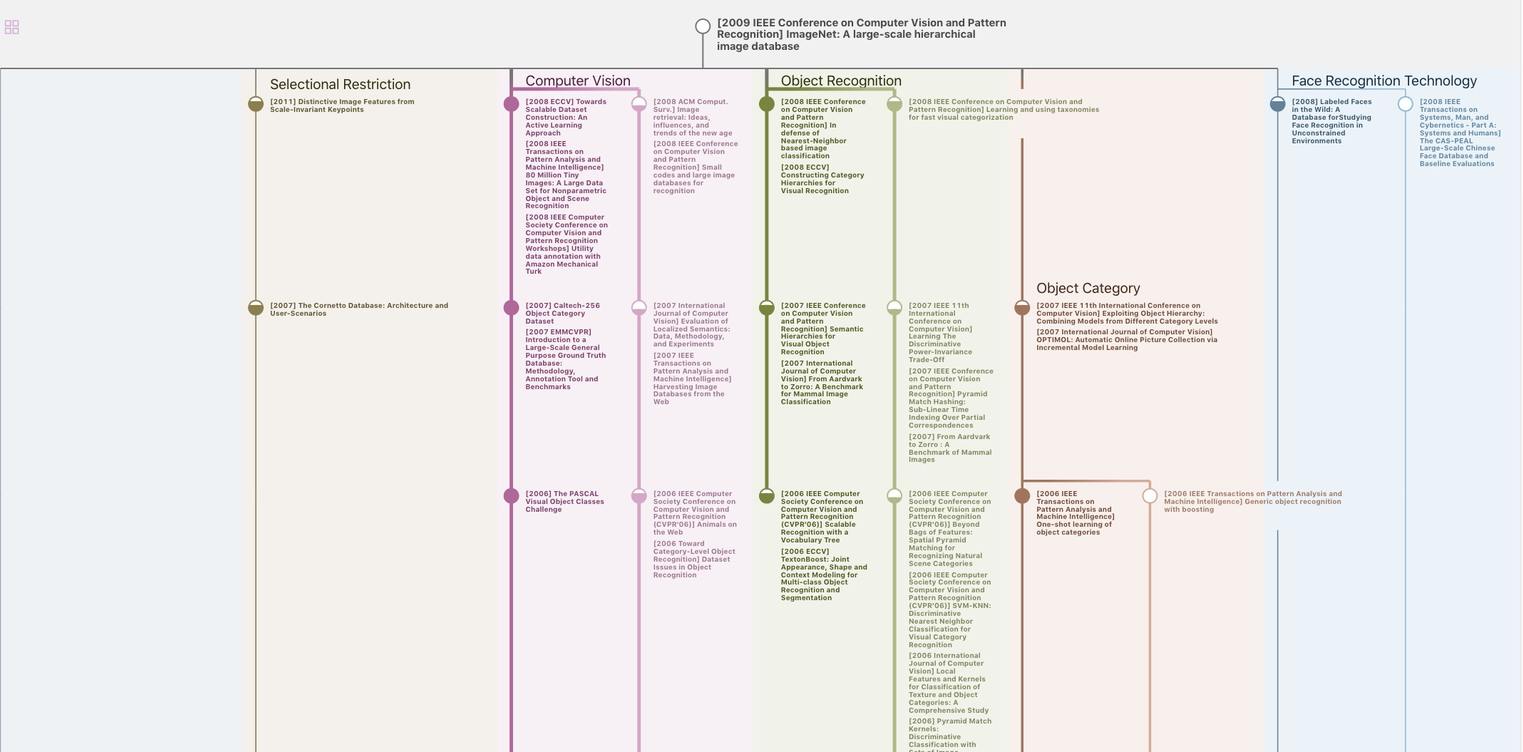
生成溯源树,研究论文发展脉络
Chat Paper
正在生成论文摘要