Enabling Off-the-Shelf Disfluency Detection and Categorization for Pathological Speech
Conference of the International Speech Communication Association (INTERSPEECH)(2022)
摘要
A speech disfluency, such as a filled pause, repetition, or revision, disrupts the typical flow of speech. Disfluency modeling has grown as a research area, as recent work has shown that these disfluencies may help in assessing health conditions. For example, for individuals with cognitive impairment, changes in disfluencies may indicate worsening symptoms. However, work on disfluency modeling has focused heavily on detection and less on categorization. Work that has focused on categorization has suffered with two specific classes: repetitions and revisions. In this paper, we evaluate how BERT (Bidirectional Encoder Representations from Transformers) compares to other models on disfluency detection and categorization. We also propose adding a second fine-tuning task where BERT learns to distance repetitions and revisions from their repairs with triplet loss. We find that BERT and BERT with triplet loss outperform previous work on disfluency detection and categorization, particularly for repetitions and revisions. In this paper we present the first analysis of how these models can be fine-tuned on widely available disfluency data, and then used in an off-the-shelf manner on small corpora of pathological speech.
更多查看译文
关键词
categorization,off-the-shelf
AI 理解论文
溯源树
样例
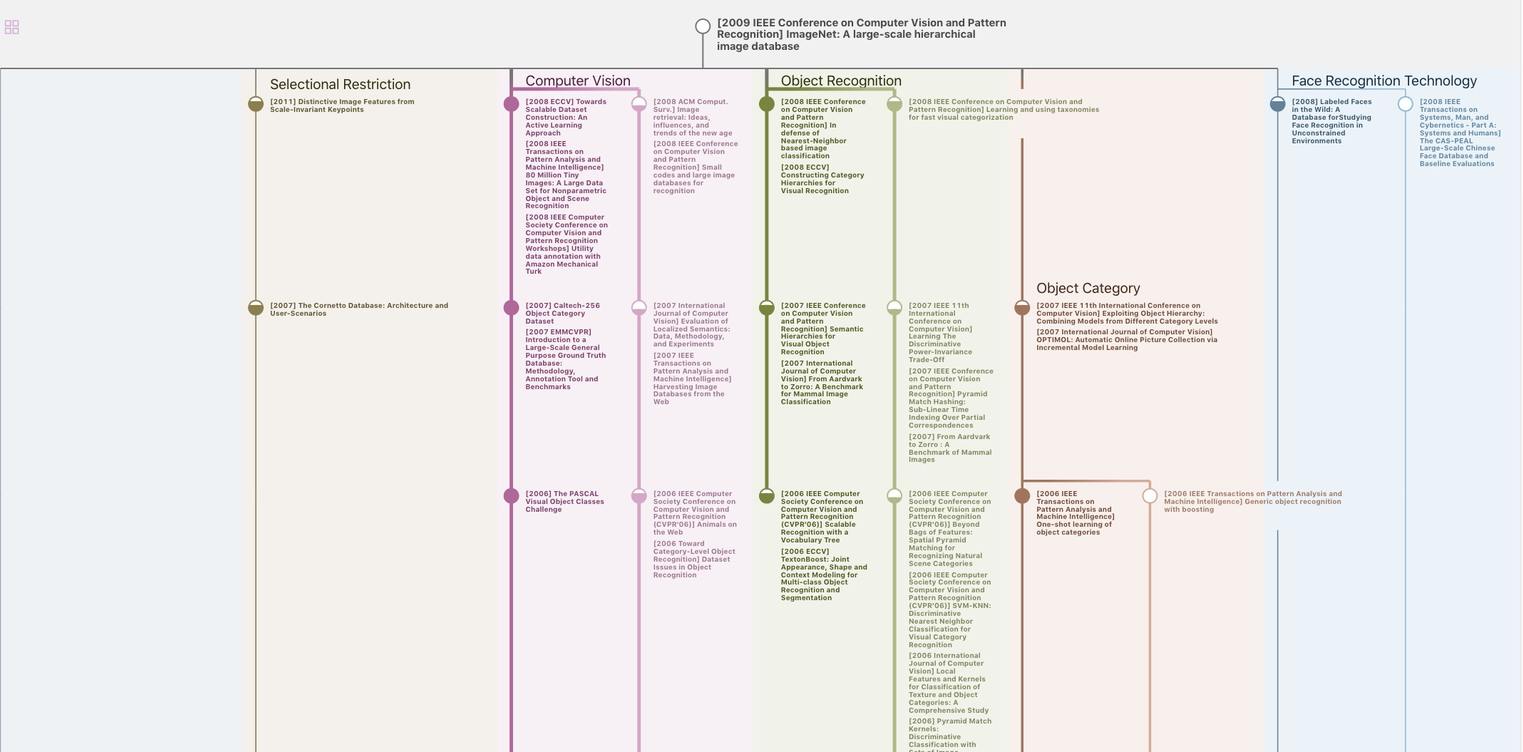
生成溯源树,研究论文发展脉络
Chat Paper
正在生成论文摘要