Adversarial Fine-Grained Fact Graph for Factuality-Oriented Abstractive Summarization
NATURAL LANGUAGE PROCESSING AND CHINESE COMPUTING, NLPCC 2022, PT I(2022)
摘要
Although neural abstractive summarization models can generate text with significant fluency and coherence, controlling the correctness of facts in generation remains a challenge. Recent work simply classifies factuality into two categories: factual or non-factual, which is coarse-grained and lacks a deep understanding of the linguistic typology of factual errors. In this paper, we present insights into two main and common factual errors (Semantic Frame Errors and Coreference Error) and propose a LASum model to enhance the factuality of abstractive summarization by adversarial fine-grained fact graph. Specifically, we first construct the fine-grained fact graph for the source document and summary to represent facts, respectively, consisting of a dependency channel and coreference channel to address the above two factual errors. And then we enhance the seq2seq model to grasp the internal facts in the fact graph from an intuitive perspective by adversarial learning (GAN). The experimental results show that our model can substantially improve the factuality compared to several strong baselines.
更多查看译文
关键词
Abstractive summarization, Factuality, Adversarial learning, Fine-grained fact graph
AI 理解论文
溯源树
样例
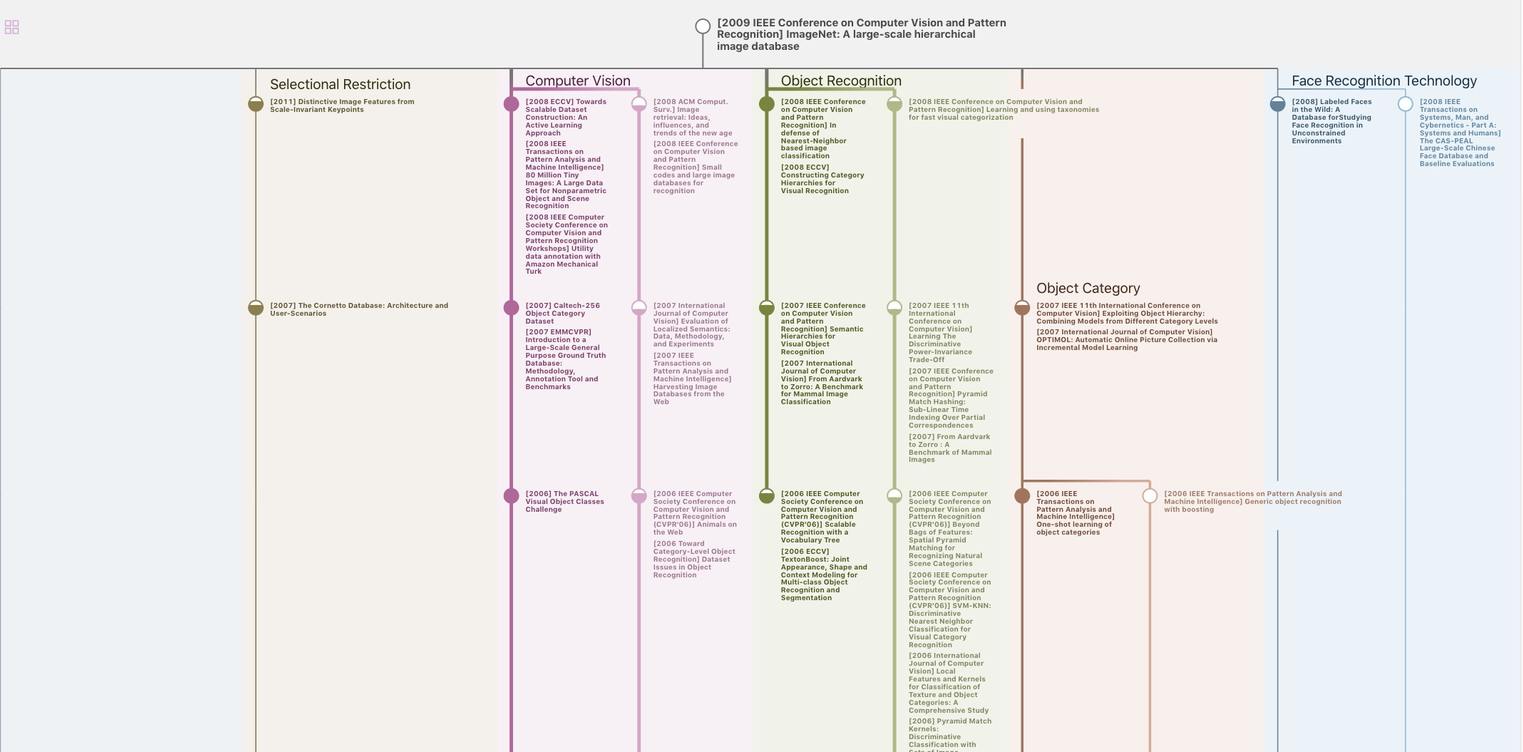
生成溯源树,研究论文发展脉络
Chat Paper
正在生成论文摘要