Employing Internal and External Knowledge to Factuality-Oriented Abstractive Summarization
NATURAL LANGUAGE PROCESSING AND CHINESE COMPUTING, NLPCC 2022, PT I(2022)
摘要
summarization models based on neural network have successfully generated human-readable and fluent summaries. However, the generated summary often has factual errors: it is inconsistent with the facts included in the source document (internal factual error) or commonsense knowledge (external factual error). To alleviate these two factual errors, we propose a novel Knowledge Aware Summarization model (KASum) that enhances the factuality of the summary by integrating internal and external knowledge simultaneously. First, KASum obtains external knowledge by utilizing the pre-trained model ERNIE combined with Knowledge Graph (KG) to reduce external factual errors. Besides, KASum obtains internal knowledge by extracting the source document's Semantic Role Information (SRI) to improve internal factuality. Finally, KASum captures the interaction of internal and external knowledge by an interactive attention module to avoid internal and external factual errors further. Experimental results on CNN/DM and XSUM show that KASum significantly improves the factuality of the generated summary compared with strong baseline models.
更多查看译文
关键词
Abstractive summarization, Factuality, Internal knowledge, External knowledge
AI 理解论文
溯源树
样例
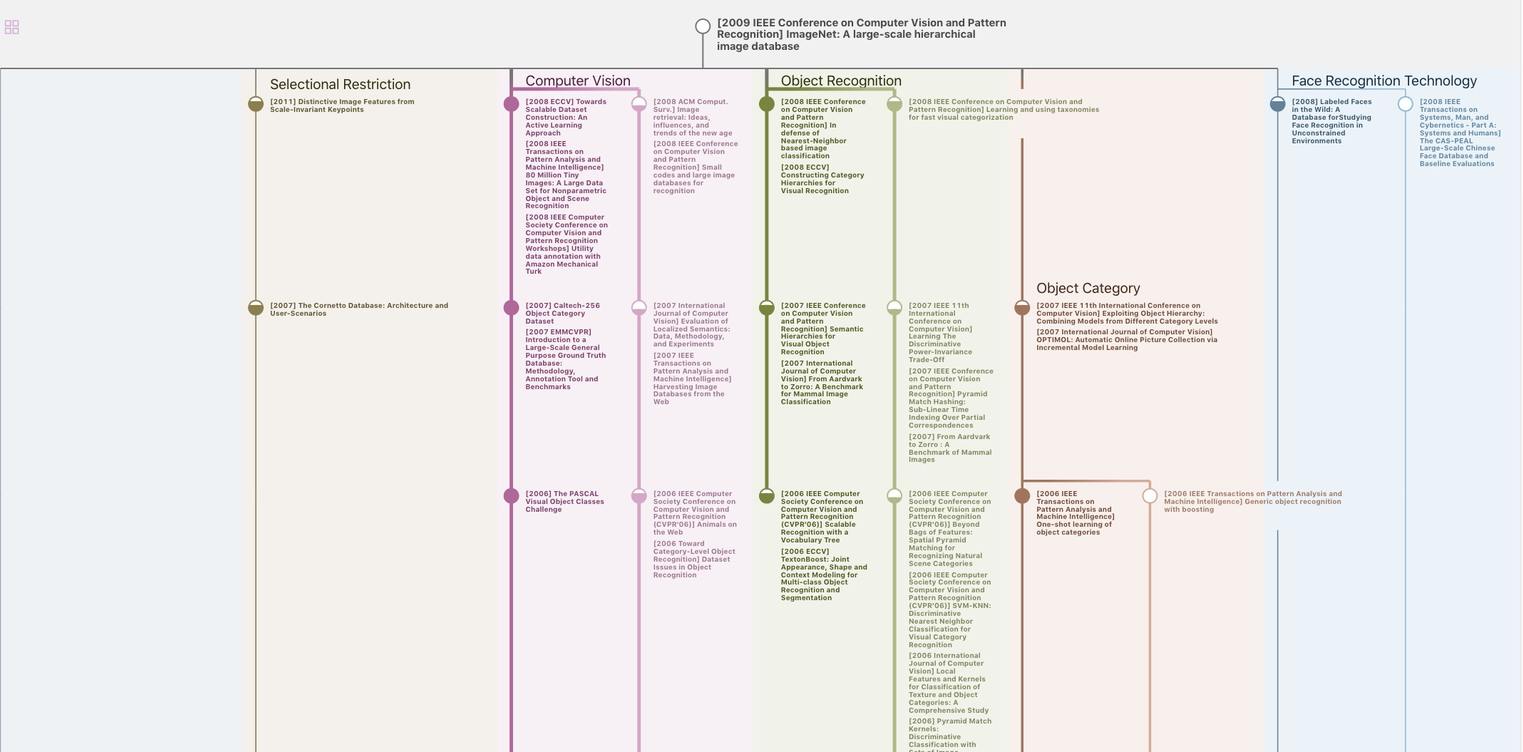
生成溯源树,研究论文发展脉络
Chat Paper
正在生成论文摘要