Linguistic Frameworks Go Toe-to-Toe at Neuro-Symbolic Language Modeling
North American Chapter of the Association for Computational Linguistics (NAACL)(2022)
摘要
We examine the extent to which, in principle, different syntactic and semantic graph representations can complement and improve neural language modeling. Specifically, by conditioning on a subgraph encapsulating the locally relevant sentence history, can a model make better next-word predictions than a pretrained sequential language model alone? With an ensemble setup consisting of GPT-2 and ground-truth graphs from one of 7 different formalisms, we find that the graph information indeed improves perplexity and other metrics. Moreover, this architecture provides a new way to compare different frameworks of linguistic representation. In our oracle graph setup, training and evaluating on English WSJ, semantic constituency structures prove most useful to language modeling performance-outpacing syntactic constituency structures as well as syntactic and semantic dependency structures.
更多查看译文
关键词
language,modeling,toe-to-toe,neuro-symbolic
AI 理解论文
溯源树
样例
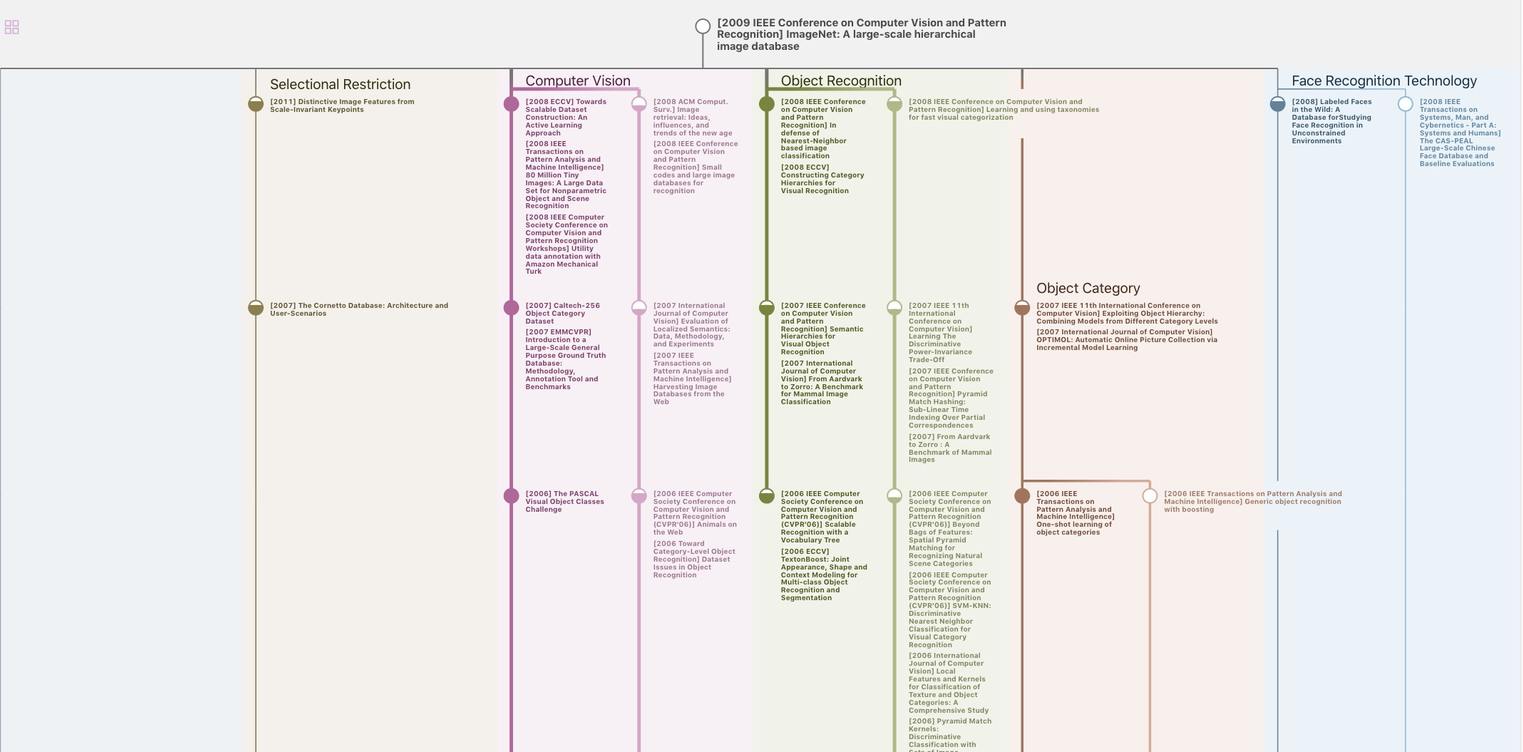
生成溯源树,研究论文发展脉络
Chat Paper
正在生成论文摘要