Revisit Overconfidence for OOD Detection: Reassigned Contrastive Learning with Adaptive Class-dependent Threshold
North American Chapter of the Association for Computational Linguistics (NAACL)(2022)
摘要
Detecting Out-of-Domain (OOD) or unknown intents from user queries is essential in a task-oriented dialog system. A key challenge of OOD detection is the overconfidence of neural models. In this paper, we comprehensively analyze overconfidence and classify it into two perspectives: over-confident OOD and in-domain (IND). Then according to intrinsic reasons, we respectively propose a novel reassigned contrastive learning (RCL) to discriminate IND intents for over-confident OOD and an adaptive class-dependent local threshold mechanism to separate similar IND and OOD intents for overconfident IND. Experiments and analyses show the effectiveness of our proposed method for both aspects of overconfidence issues.(1)
更多查看译文
AI 理解论文
溯源树
样例
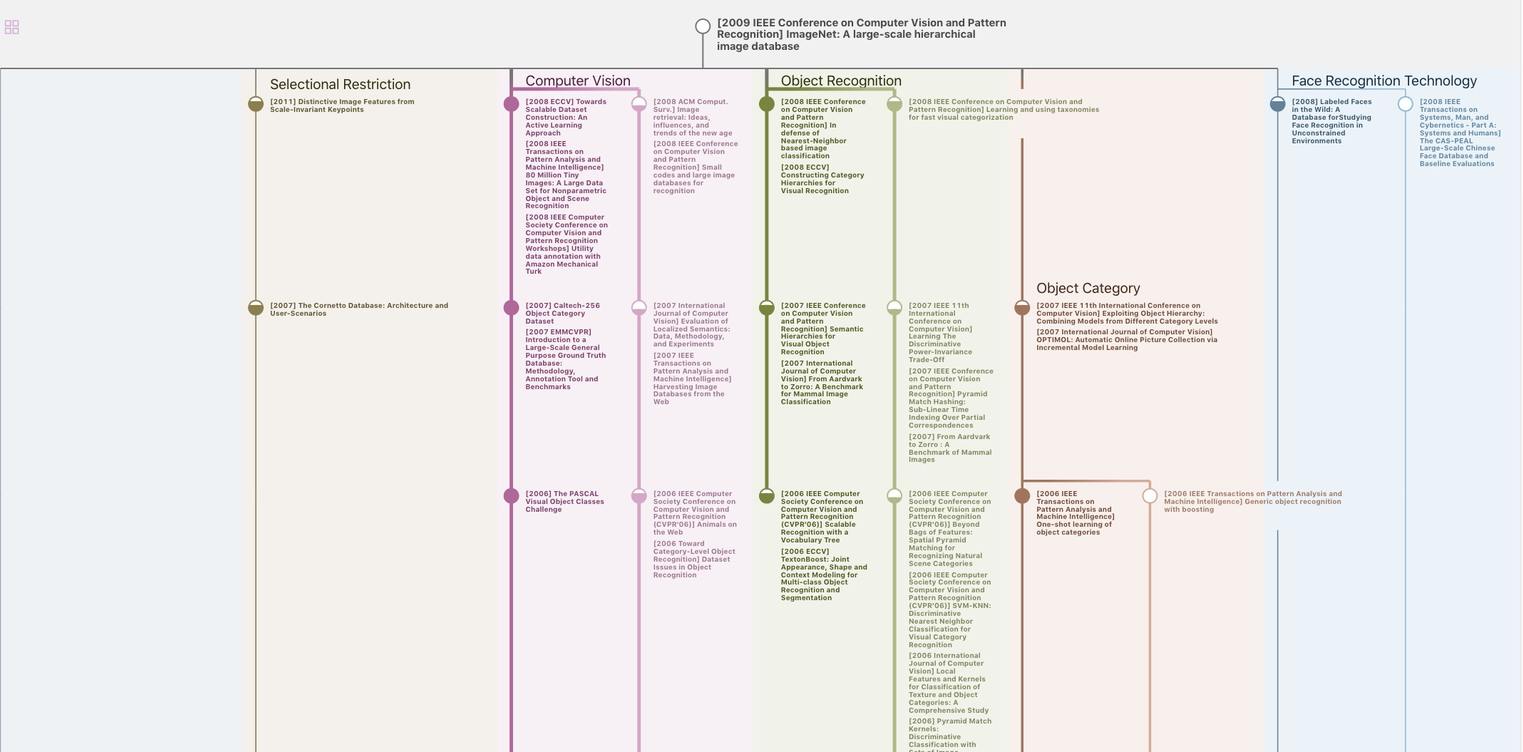
生成溯源树,研究论文发展脉络
Chat Paper
正在生成论文摘要