Dynamically Adaptive Adjustment Loss Function Biased Towards Few‐class Learning
IET IMAGE PROCESSING(2023)
摘要
Convolution neural networks have been widely used in the field of computer vision, which effectively solve practical problems. However, the loss function with fixed parameters will affect the training efficiency and even lead to poor prediction accuracy. In particular, when there is a class imbalance in the data, the final result tends to favor the large-class. In detection and recognition problems, the large-class will dominate due to its quantitative advantage, and the features of few-class can be not fully learned. In order to learn few-class, batch nuclear-norm maximization is introduced to the deep neural networks, and the mechanism of the adaptive composite loss function is established to increase the diversity of the network and thus improve the accuracy of prediction. The proposed loss function is added to the crowd counting, and verified on ShanghaiTech and UCF_CC_50 datasets. Experimental results show that the proposed loss function improves the prediction accuracy and convergence speed of deep neural networks.
更多查看译文
关键词
Deep Learning
AI 理解论文
溯源树
样例
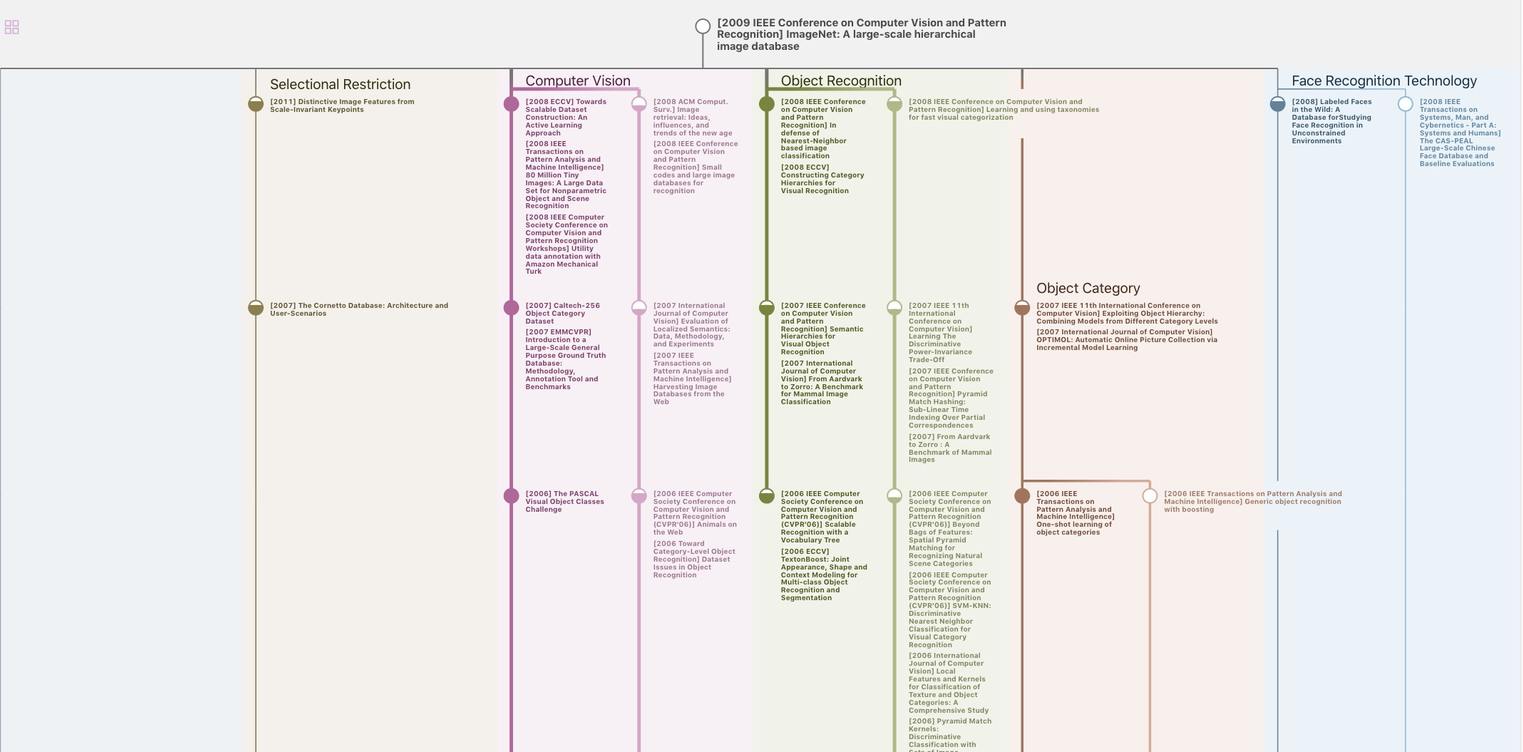
生成溯源树,研究论文发展脉络
Chat Paper
正在生成论文摘要