On Flows of Neural Ordinary Differential Equations That Are Solutions of Lotka-Volterra Dynamical Systems.
International Conference of the Catalan Association for Artificial Intelligence (CCIA)(2022)
摘要
Neural Ordinary Differential Equations (NODE) have emerged as a novel approach to deep learning, where instead of specifying a discrete sequence of hidden layers, it parameterizes the derivative of the hidden state using a neural network [1]. The solution to the underlying dynamical system is a flow, and various works have explored the universality of flows, in the sense of being able to approximate any analytical function. In this paper we present preliminary work aimed at identifying families of systems of ordinary differential equations (SODE) that are universal, in the sense that they encompass most of the systems of differential equations that appear in practice. Once one of these (candidate) universal SODEs is found, we define a process that generates a family of NODEs whose flows are precisely the solutions of the universal SODEs found above. The candidate universal SODE family that we present here is the generalized Lotka-Volterra (LV) families of differential equations. We present the NODE models built upon this LV systems and a description of their appropriate flows and some preliminary implementations of this process.
更多查看译文
关键词
neural ordinary differential equations,differential equations,flows,lotka-volterra
AI 理解论文
溯源树
样例
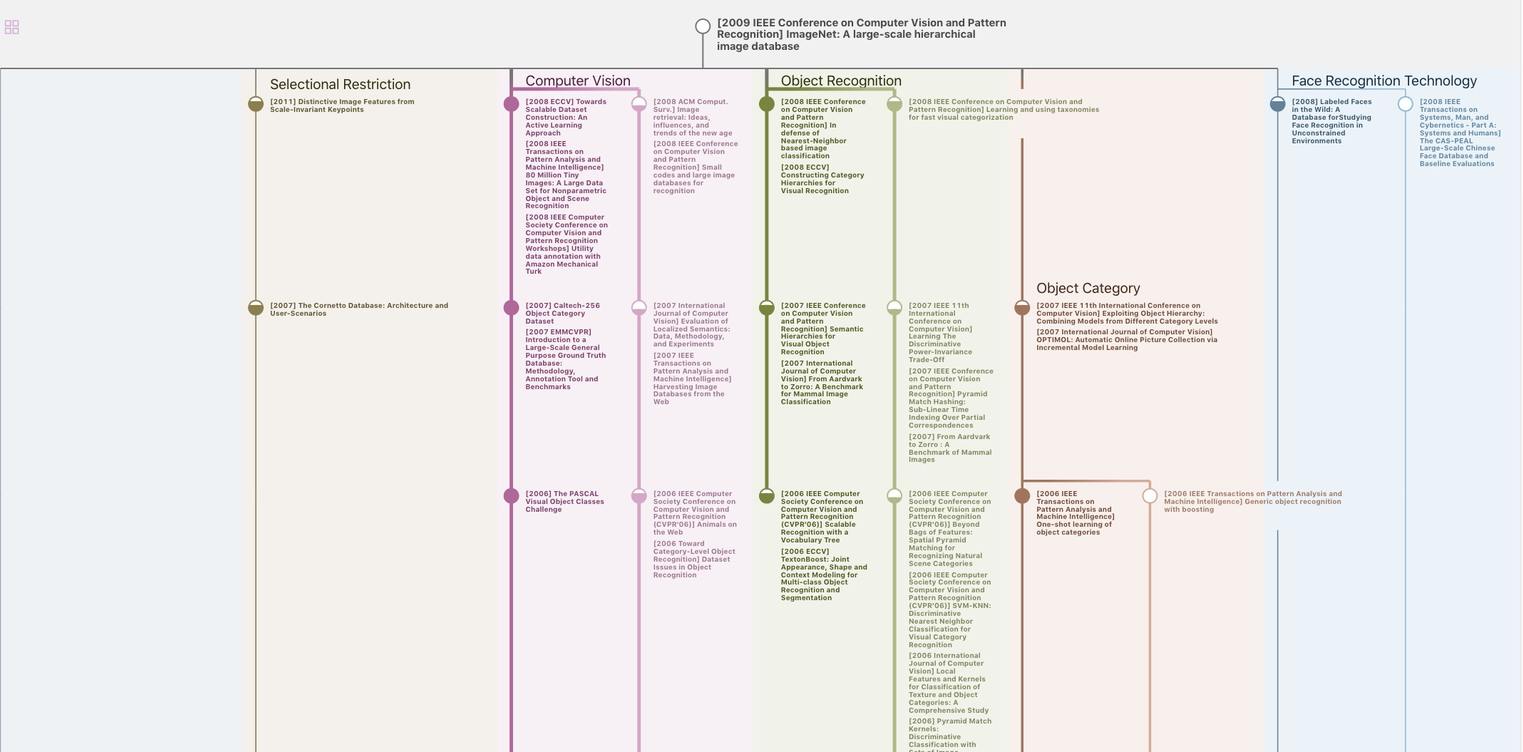
生成溯源树,研究论文发展脉络
Chat Paper
正在生成论文摘要