Limiting Reconstruction Capability of Autoencoders Using Moving Backward Pseudo Anomalies
2022 19th International Conference on Ubiquitous Robots (UR)(2022)
摘要
Video anomaly detection is one of important components in autonomous surveillance system. However, since anomalous events rarely occurs, it is common to approach this problem using one-class-classification problem in which only normal training data are provided. Typically, an autoencoder (AE) is trained to reconstruct the normal data. As the AE is not trained using the real anomalies, it is expected to poorly reconstruct anomalies in the test time. However, the expectation is often not met as AE can also reconstruct anomalous data as well. Several researchers propose to limit the reconstruction capability of AE using pseudo anomalies constructed from the normal data. In this work, we explore another type of pseudo anomaly, i.e., moving backward. Experiments in two video anomaly detection benchmark datasets, i.e., Ped2 and Avenue, show the effectiveness of our method in limiting the reconstruction capability of AE.
更多查看译文
关键词
reconstruction capability,autoencoder,moving backward pseudoanomalies,autonomous surveillance system,anomalous events,one-class-classification problem,normal training data,normal data,anomalous data,pseudoanomaly,video anomaly detection benchmark datasets
AI 理解论文
溯源树
样例
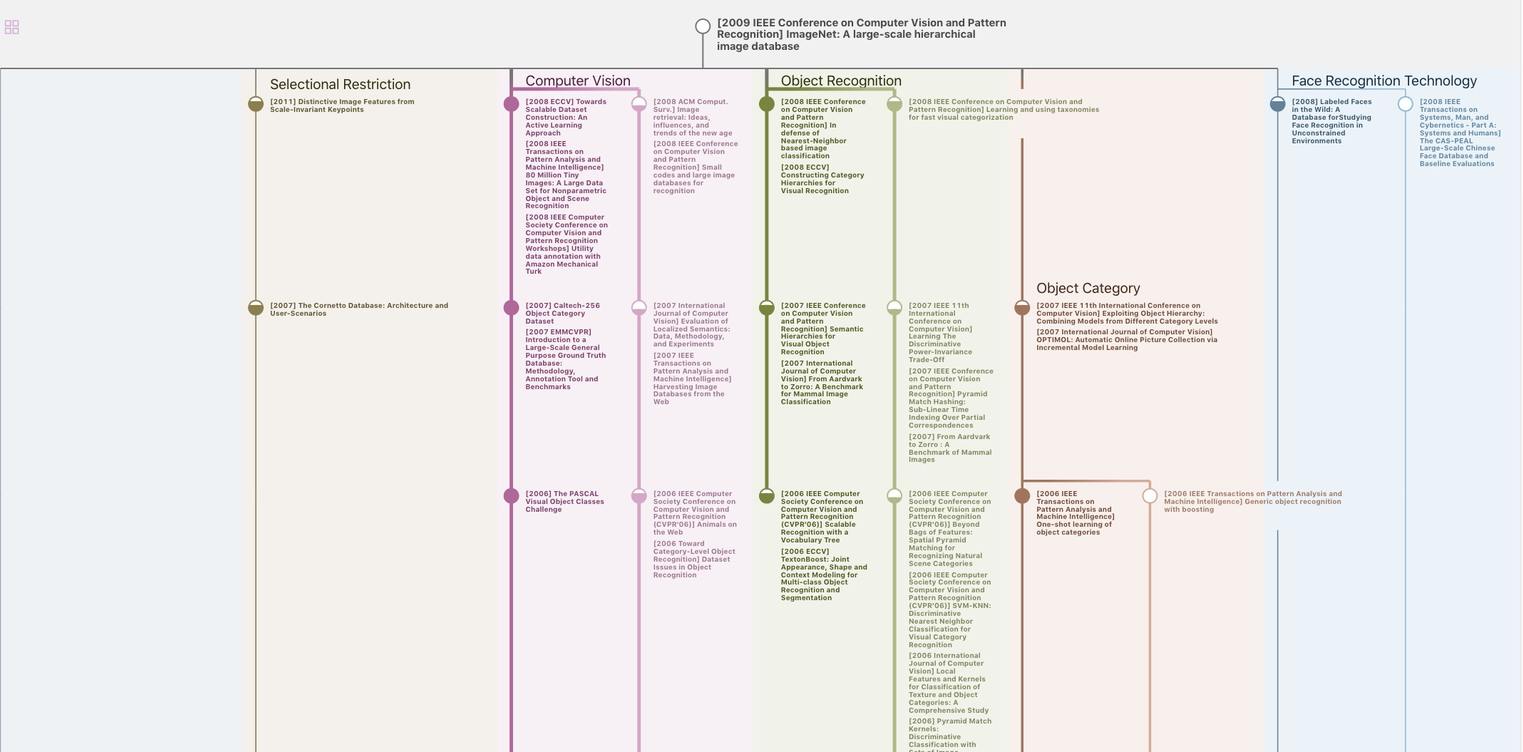
生成溯源树,研究论文发展脉络
Chat Paper
正在生成论文摘要