Mono-DCNet: Monocular 3D Object Detection via Depth-based Centroid Refinement and Pose Estimation
2022 IEEE Intelligent Vehicles Symposium (IV)(2022)
摘要
3D object detection is a well-known problem for autonomous systems. Most of the existing methods use sensor fusion techniques with Radar, LiDAR, and Cameras. However, one of the challenges is to estimate the 3D shape and location of the adjoining vehicles from a single monocular image without other 3D sensors; such as Radar or LiDAR. To solve the lack of the depth information, a novel method for 3D vehicle detection is presented. In this work, instead of using the whole depth map and the viewing angle (allocentric angle), only the depth mask of each object is used to refine the projected centroid and estimate its egocentric angle directly. The performance of the proposed method is tested and validated using the KITTI dataset, obtaining similar results to other state-of-the-art methods for Monocular 3D Object Detection.
更多查看译文
关键词
mono-DCNet,Monocular 3D,depth-based centroid refinement,pose estimation,autonomous systems,sensor fusion techniques,LiDAR,adjoining vehicles,single monocular image,depth information,3D vehicle detection,depth map,viewing angle,allocentric angle,depth mask,projected centroid,egocentric angle
AI 理解论文
溯源树
样例
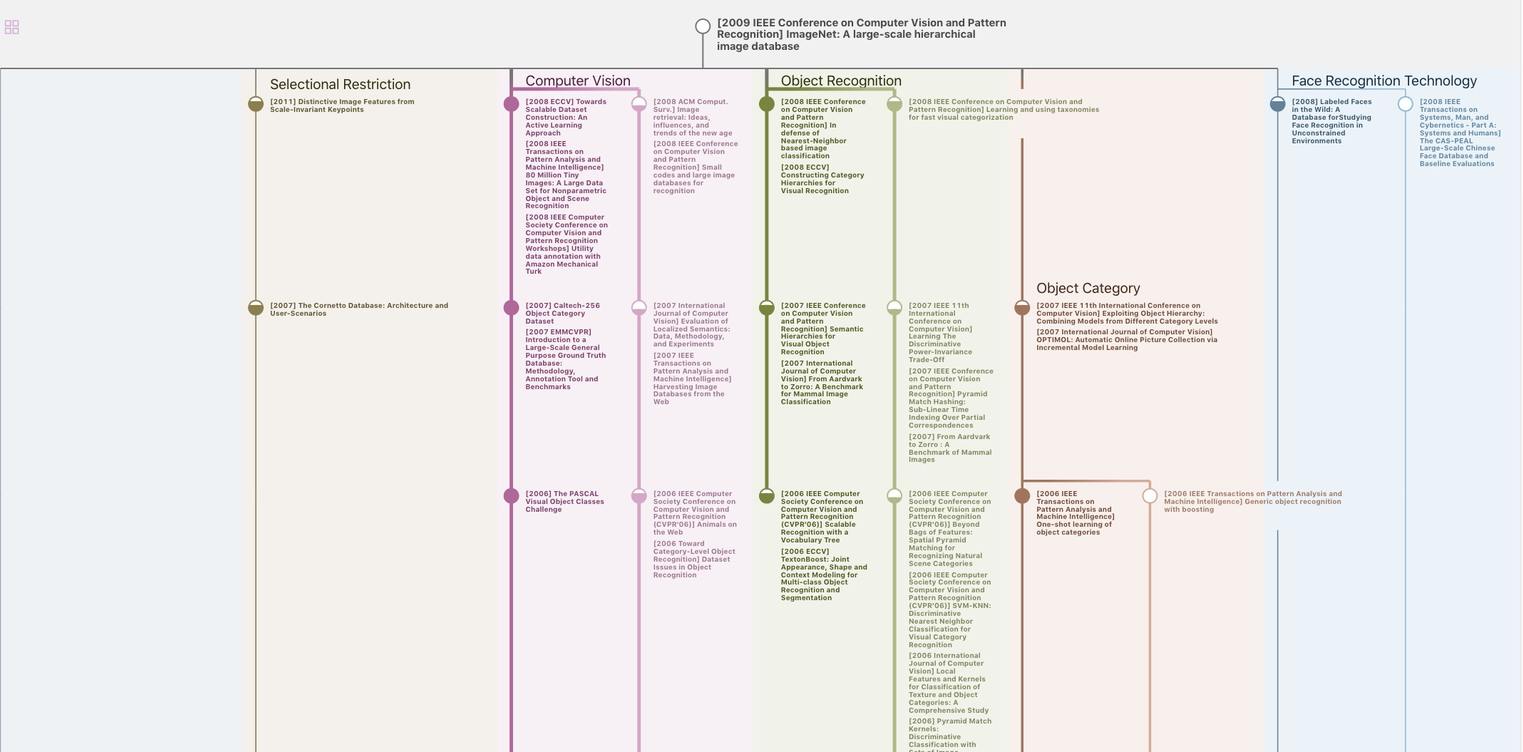
生成溯源树,研究论文发展脉络
Chat Paper
正在生成论文摘要