Traffic Sign Classifiers Under Physical World Realistic Sticker Occlusions: A Cross Analysis Study
2022 IEEE Intelligent Vehicles Symposium (IV)(2022)
摘要
Recent adversarial attacks with real world applications are capable of deceiving deep neural networks (DNN), which often appear as printed stickers applied to objects in physical world. Though achieving high success rate in lab tests and limited field tests, such attacks have not been tested on multiple DNN architectures with a standard setup to unveil the common robustness and weakness points of both the DNNs and the attacks. Furthermore, realistic looking stickers applied by normal people as acts of vandalism are not studied to discover their potential risks as well the risk of optimizing the location of such realistic stickers to achieve the maximum performance drop. In this paper, (a) we study the case of realistic looking sticker application effects on traffic sign detectors performance; (b) we use traffic sign image classification as our use case and train and attack 11 of the modern architectures for our analysis; (c) by considering different factors like brightness, blurriness and contrast of the train images in our sticker application procedure, we show that simple image processing techniques can help realistic looking stickers fit into their background to mimic real world tests; (d) by performing structured synthetic and real-world evaluations, we study the difference of various traffic sign classes in terms of their crucial distinctive features among the tested DNNs.
更多查看译文
关键词
traffic sign detectors performance,traffic sign image classification,sticker application procedure,real-world evaluations,traffic sign classes,traffic sign classifiers,physical world realistic sticker occlusions,deep neural networks,printed stickers,lab tests,limited field tests,multiple DNN architectures,weakness points,realistic stickers,maximum performance drop,realistic looking sticker application effects,tested DNN
AI 理解论文
溯源树
样例
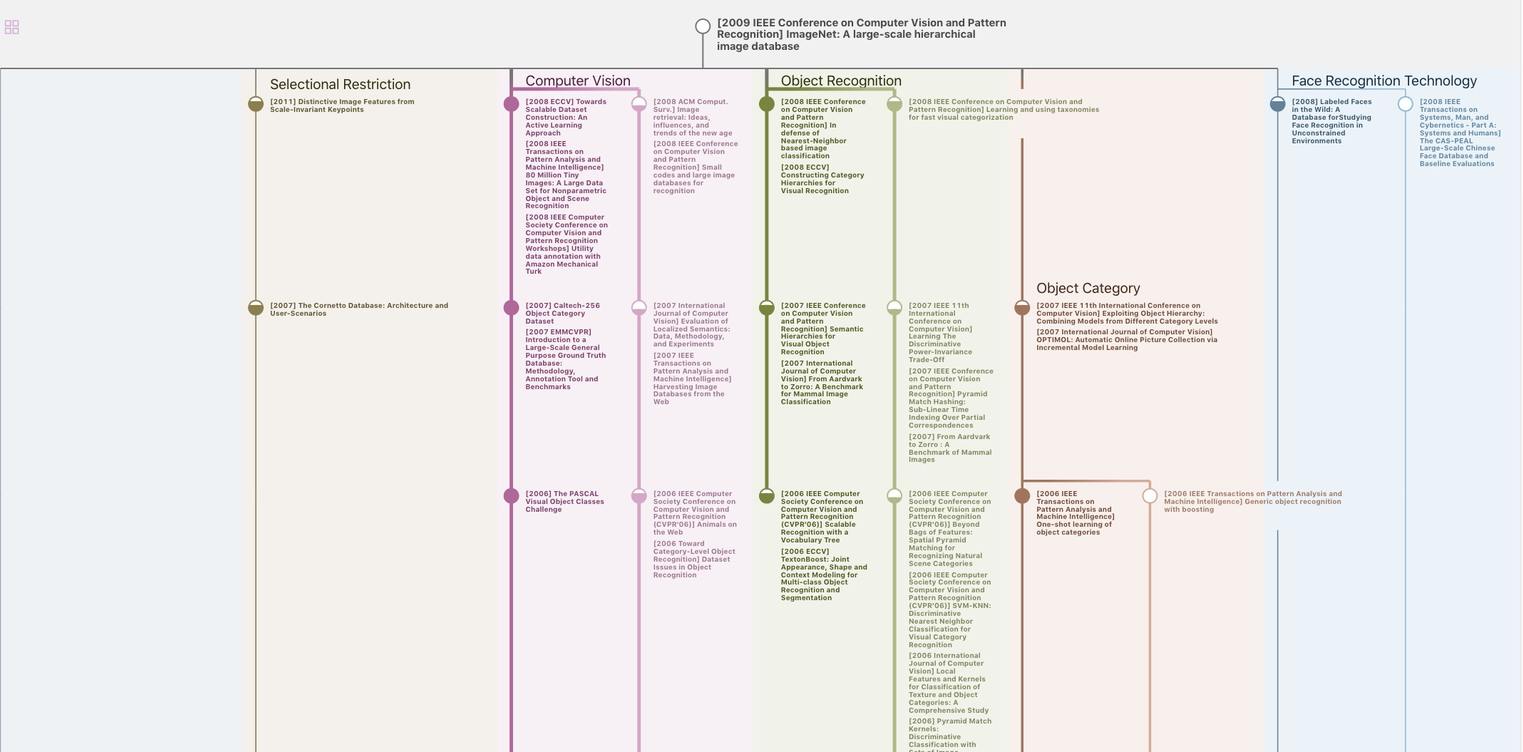
生成溯源树,研究论文发展脉络
Chat Paper
正在生成论文摘要