GFlowCausal: Generative Flow Networks for Causal Discovery
CoRR(2023)
摘要
Causal discovery aims to uncover causal structure among a set of variables. Score-based approaches mainly focus on searching for the best Directed Acyclic Graph (DAG) based on a predefined score function. However, most of them are not applicable on a large scale due to the limited searchability. Inspired by the active learning in generative flow networks, we propose a novel approach to learning a DAG from observational data called GFlowCausal. It converts the graph search problem to a generation problem, in which direct edges are added gradually. GFlowCausal aims to learn the best policy to generate high-reward DAGs by sequential actions with probabilities proportional to predefined rewards. We propose a plug-and-play module based on transitive closure to ensure efficient sampling. Theoretical analysis shows that this module could guarantee acyclicity properties effectively and the consistency between final states and fully-connected graphs. We conduct extensive experiments on both synthetic and real datasets, and results show the proposed approach to be superior and also performs well in a large-scale setting.
更多查看译文
关键词
generative gflowcausal networks,discovery
AI 理解论文
溯源树
样例
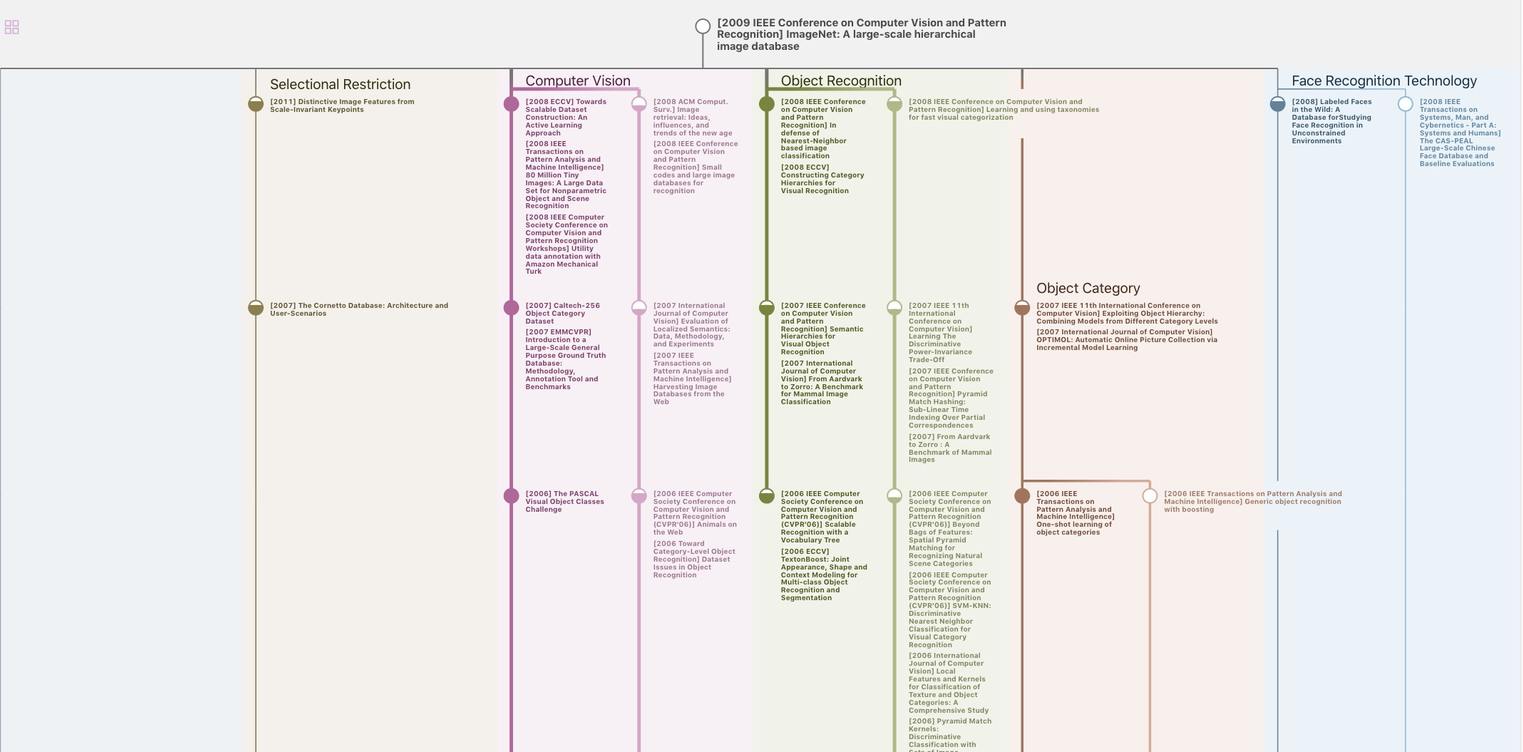
生成溯源树,研究论文发展脉络
Chat Paper
正在生成论文摘要