Heterogeneous Feature Distillation Network for SAR Image Semantic Segmentation
arXiv (Cornell University)(2022)
摘要
Semantic segmentation for SAR (Synthetic Aperture Radar) images has attracted increasing attention in the remote sensing community recently, due to SAR's all-time and all-weather imaging capability. However, SAR images are generally more difficult to be segmented than their EO (Electro-Optical) counterparts, since speckle noises and layovers are inevitably involved in SAR images. To address this problem, we investigate how to introduce EO features to assist the training of a SAR-segmentation model, and propose a heterogeneous feature distillation network for segmenting SAR images, called HFD-Net, where a SAR-segmentation student model gains knowledge from a pre-trained EO-segmentation teacher model. In the proposed HFD-Net, both the student and teacher models employ an identical architecture but different parameter configurations, and a heterogeneous feature distillation model is explored for transferring latent EO features from the teacher model to the student model and then enhancing the ability of the student model for SAR image segmentation. In addition, a heterogeneous feature alignment module is explored to aggregate multi-scale features for segmentation in each of the student model and teacher model. Extensive experimental results on two public datasets demonstrate that the proposed HFD-Net outperforms seven state-of-the-art SAR image semantic segmentation methods.
更多查看译文
关键词
sar image semantic
AI 理解论文
溯源树
样例
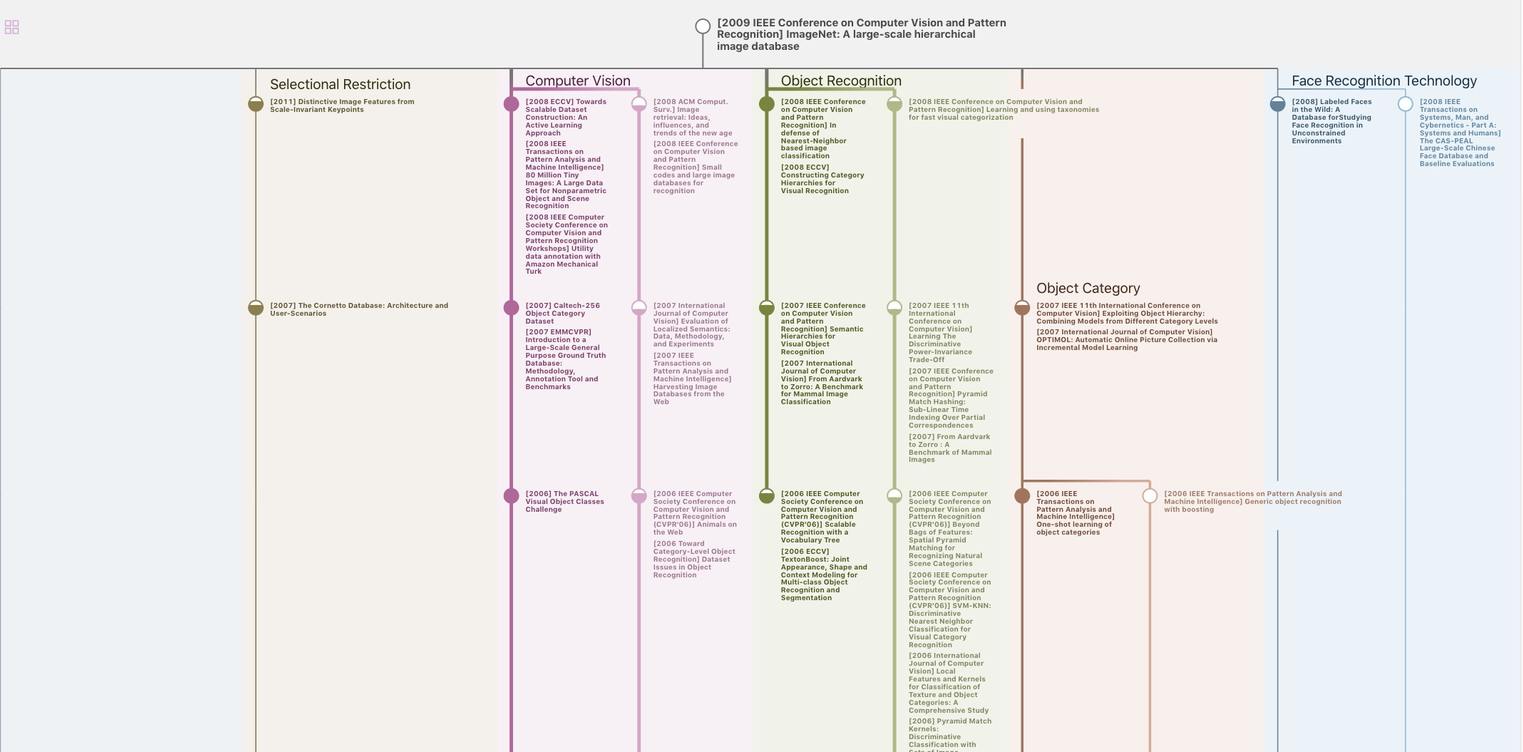
生成溯源树,研究论文发展脉络
Chat Paper
正在生成论文摘要