Predictive Maintenance for Maintenance-Effective Manufacturing Using Machine Learning Approaches
17th International Conference on Soft Computing Models in Industrial and Environmental Applications (SOCO 2022)(2022)
摘要
Recent advancements in technologies such as in Big data and Internet of Things have made Predictive Maintenance (PdM) a key strategy to reduce unnecessary maintenance costs and improve product quality in the manufacturing sector. The premise of this paper is to implement and explore some of the most promising machine learning models for PdM, a Gradient Boosting (GB) model, and a Support Vector Machine (SVM) model. An innovative methodology for model training is proposed that aims to improve model performance while also allowing for continuous training. Furthermore, it is proposed an automatic hyperparameter tunning approach for the GB and SVM models. A synthetic dataset that reflects industrial machine data was used to validate the proposed methodology. The implemented models can achieve up to 0.92 recall and 94.55% accuracy, highlighting the effectiveness of the proposed methodology.
更多查看译文
关键词
Data preprocessing, Gradient boosting, Hyperparameter optimization, Predictive maintenance, Support vector machine
AI 理解论文
溯源树
样例
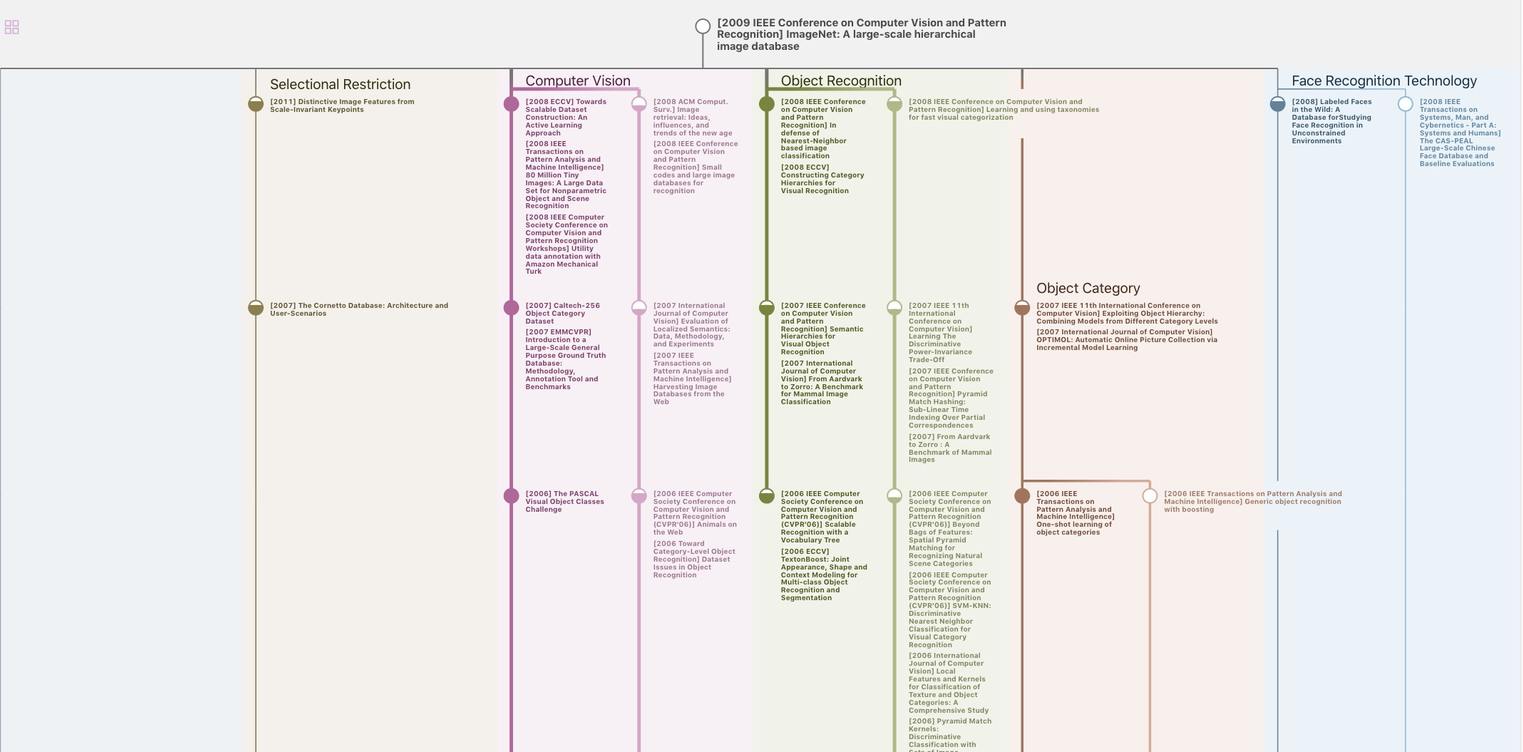
生成溯源树,研究论文发展脉络
Chat Paper
正在生成论文摘要