Deep Learning Approach for the Prediction of the Concentration of Chlorophyll ɑ in Seawater. A Case Study in El Mar Menor (Spain)
17th International Conference on Soft Computing Models in Industrial and Environmental Applications (SOCO 2022)(2022)
摘要
The goal of this research is to develop accurate and reliable forecasting models for chlorophyll ɑ concentrations in seawater at multiple depth levels in El Mar Menor (Spain). Chlorophyll ɑ can be used as a eutrophication indicator, which is especially essential in a rich yet vulnerable ecosystem like the study area. Bayesian regularized artificial neural networks and Long Short-term Memory Neural Networks (LSTMs) employing a rolling window approach were used as forecasting algorithms with a one-week prediction horizon. Two input strategies were tested: using data from the own time series or including exogenous variables among the inputs. In this second case, mutual information and the Minimum-Redundancy-Maximum-Relevance approach were utilized to select the most relevant variables. The models obtained reasonable results for the univariate input scheme with
$$\overline{\sigma }$$
values over 0.75 in levels between 0.5 and 2 m. The inclusion of exogenous variables increased these values to above 0.85 for the same depth levels. The models and methodologies presented in this paper can constitute a very useful tool to help predict eutrophication episodes and act as decision-making tools that allow the governmental and environmental agencies to prevent the degradation of El Mar Menor.
更多查看译文
关键词
Chlorophyll, Machine learning, Deep learning, LSTM, Artificial neural networks, Mar Menor, Eutrophication, Bayesian regularization
AI 理解论文
溯源树
样例
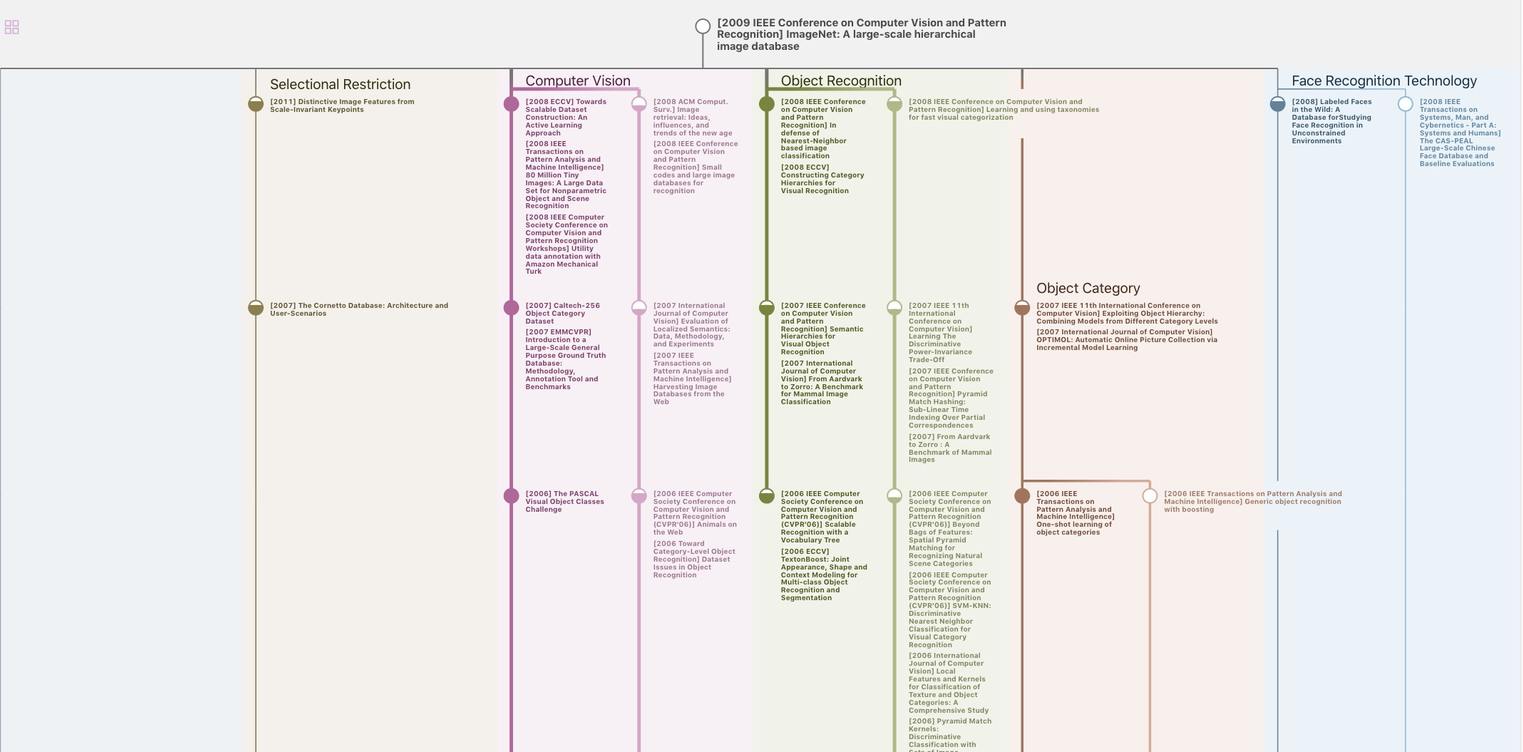
生成溯源树,研究论文发展脉络
Chat Paper
正在生成论文摘要