Semi-supervised Machine Learning of Optical In-Situ Monitoring Data for Anomaly Detection in Laser Powder Bed Fusion
Virtual and physical prototyping(2022)
摘要
Laser powder bed fusion (L-PBF) is one of the most widely used metal additive manufacturing technology for fabrication of functional and structural components. However, inconsistency in quality and reliability of L-PBF products is still a significant barrier preventing it from wider adoption. Machine learning (ML) of monitoring data offers a unique solution to effectively identify possible defects and predict the quality of L-PBF products. In this work, we introduce a semi-supervised ML approach to detect anomalies that occurred in L-PBF products. We train the ML model to classify surface appearances in the reference monitoring data. We then correlate the classified appearances to post-process characteristics, e.g. surface roughness, morphology, or tensile strength. We demonstrate that the established correlation enables the determination of key appearances indicative of the quality of the printed samples including anomaly-free, lack-of-fusion and overheated. We further validate our ML approach by performing prediction on test samples having various geometries.
更多查看译文
关键词
Machine learning,additive manufacturing,laser powder bed fusion,quality control
AI 理解论文
溯源树
样例
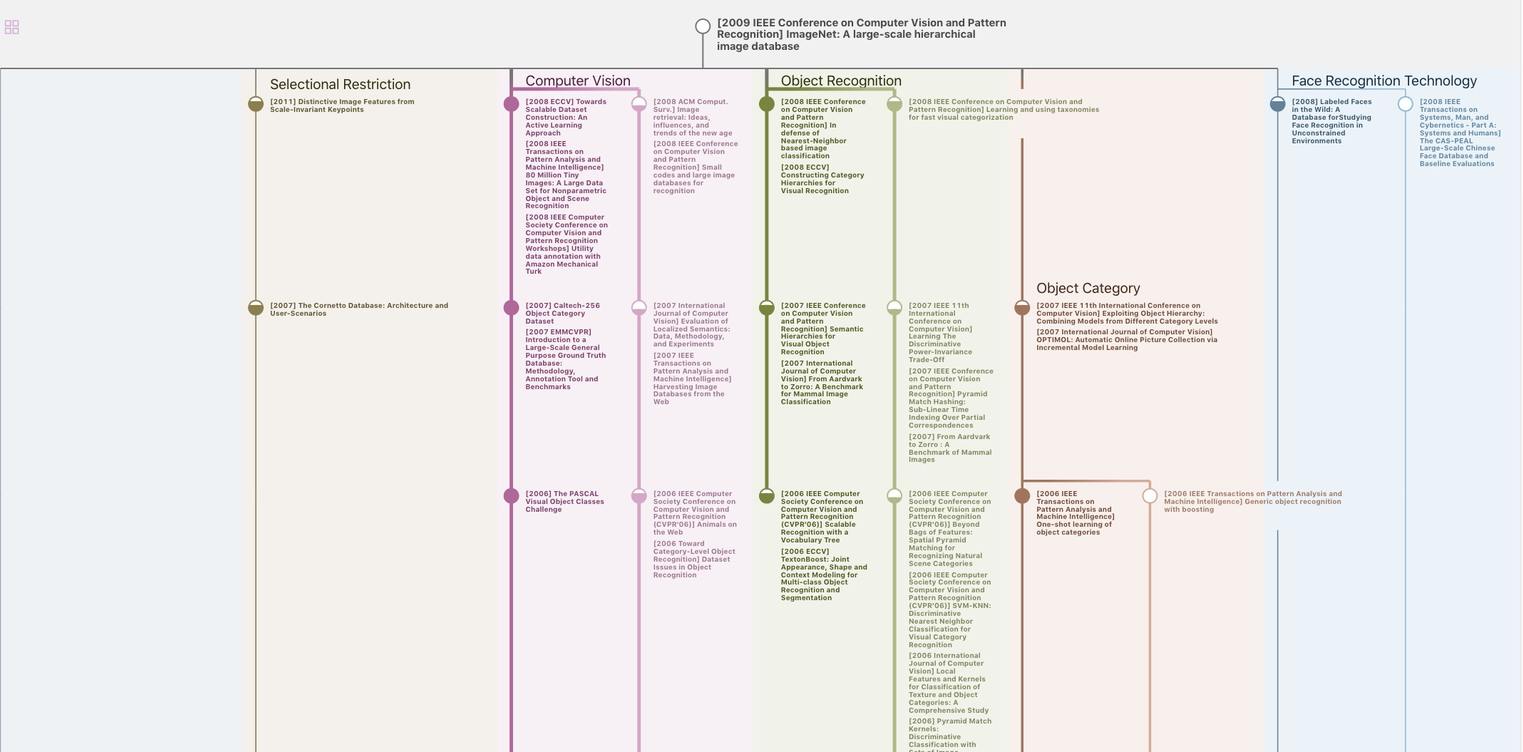
生成溯源树,研究论文发展脉络
Chat Paper
正在生成论文摘要