Hybrid Machine Learning for Anomaly Detection in Industrial Time-Series Measurement Data
2022 IEEE International Instrumentation and Measurement Technology Conference (I2MTC)(2022)
摘要
This paper presents a parallel hybrid machine learning system for the identification of anomalies in large sets of multivariate time-series (MVTS) measurement data. The goal is to achieve a more reliable detection of anomalies in safety relevant applications. Key performance indicators (KPI) are used as a measure for predicted possible sources of error. Whereas, a long short-term memory (LSTM-VAE) variational autoencoder is used to model the system behavior; the variational portion ensures the statistical uncertainty of the data is taken into account during training of the network. Combined in a parallel hybrid manner this provides a more reliable anomaly detection. The proposed structure is validated with a case study relating to a ground improvement process for building foundations. The data consists of large sets of real-time multi-variate time-series sensor data, emanating from the instrumented drilling rig. The performance of the LSTM-VAE is optimized using a genetic algorithm to select the optimal values for the hyperparameters. The implemented framework will also support future research into hybrid learning systems applied to real-time machine data analysis.
更多查看译文
关键词
Hybrid Learning,Outlier Detection,Time Series
AI 理解论文
溯源树
样例
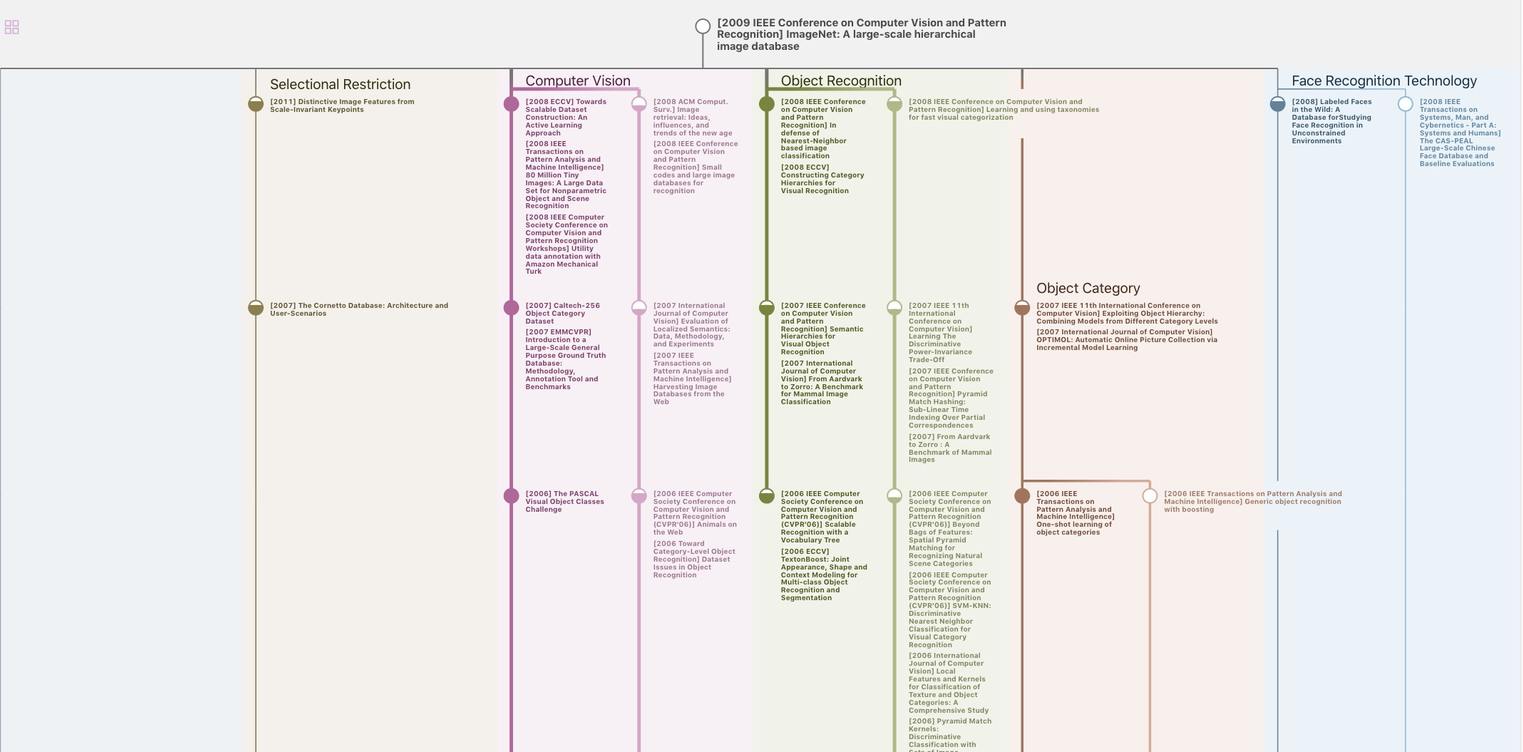
生成溯源树,研究论文发展脉络
Chat Paper
正在生成论文摘要